在本文中,我将讨论六个可以大大加快熊猫代码速度的工具。我按照一种原则组装了工具-易于集成到现有代码库中。对于大多数工具,您只需要安装模块并添加几行代码即可。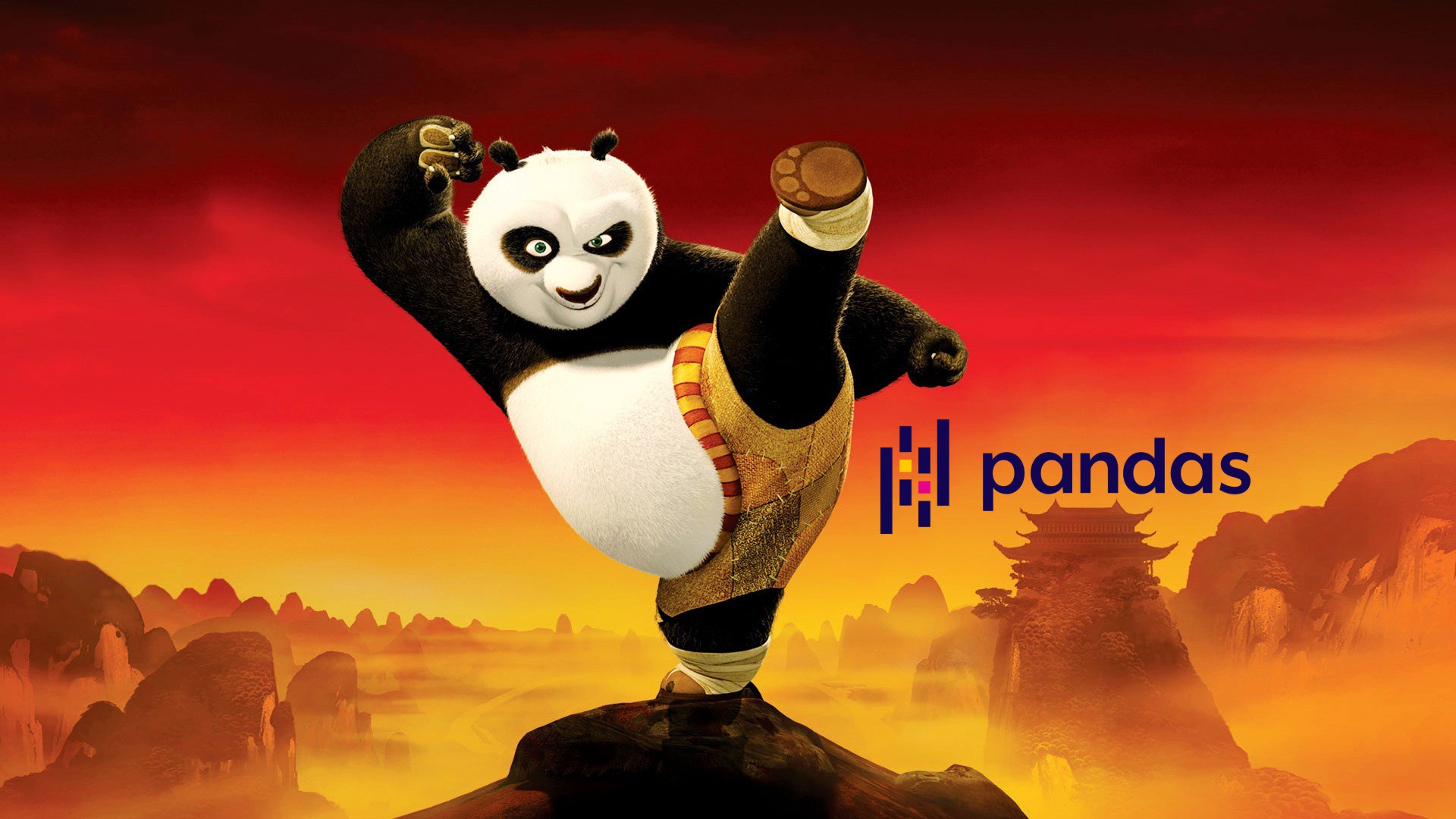
熊猫一直以来都是任何开发人员必不可少的工具,这要归功于简单易懂的API,以及用于清理,研究和分析数据的丰富工具集。一切都会好起来的,但是当涉及到不适合RAM或需要复杂计算的数据时,其性能就会开始被忽略。
在本文中,我将不会描述质的数据分析方法,例如Spark或DataFlow。相反,我将描述六个有趣的工具并演示其使用结果:
第1部分:
Numba
Python. Numba — JIT , , Numpy, Pandas. , .
— , - apply
.
import numpy as np
import numba
df = pd.DataFrame(np.random.randint(0,100,size=(100000, 4)),columns=['a', 'b', 'c', 'd'])
def multiply(x):
return x * 5
@numba.vectorize
def multiply_numba(x):
return x * 5
, . . .
In [1]: %timeit df['new_col'] = df['a'].apply(multiply)
23.9 ms ± 1.93 ms per loop (mean ± std. dev. of 7 runs, 10 loops each)
In [2]: %timeit df['new_col'] = df['a'] * 5
545 µs ± 21.4 µs per loop (mean ± std. dev. of 7 runs, 1000 loops each)
In [3]: %timeit df['new_col'] = multiply_numba(df['a'].to_numpy())
329 µs ± 2.37 µs per loop (mean ± std. dev. of 7 runs, 1000 loops each)
~70 ! , , Pandas , . :
def square_mean(row):
row = np.power(row, 2)
return np.mean(row)
@numba.njit
def square_mean_numba(arr):
res = np.empty(arr.shape[0])
arr = np.power(arr, 2)
for i in range(arr.shape[0]):
res[i] = np.mean(arr[i])
return res
:
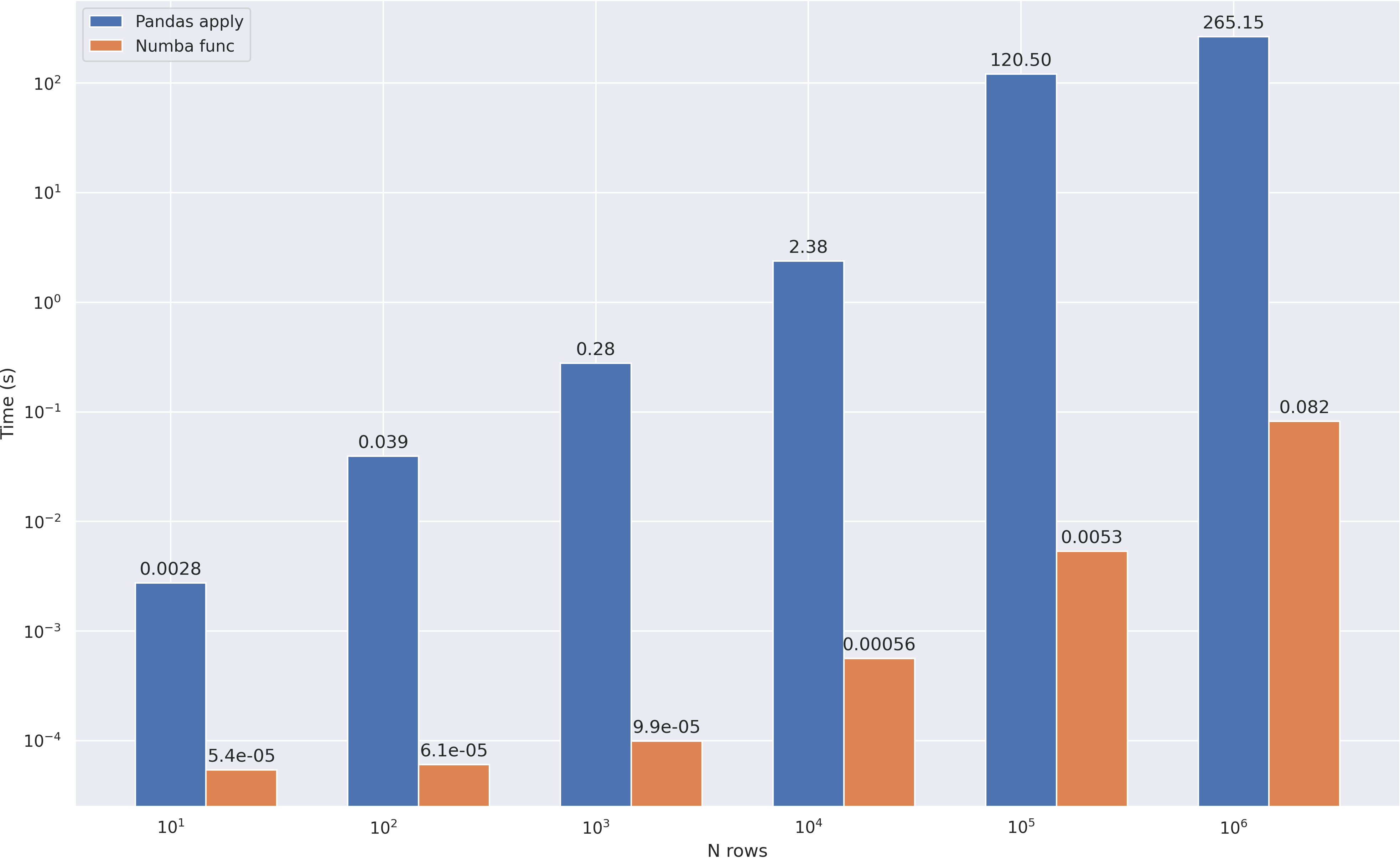
Multiprocessing
, , , . - , python.
. . , apply
:
df = pd.read_csv('abcnews-date-text.csv', header=0)
df = pd.concat([df] * 10)
df.head()
def mean_word_len(line):
for i in range(6):
words = [len(i) for i in line.split()]
res = sum(words) / len(words)
return res
def compute_avg_word(df):
return df['headline_text'].apply(mean_word_len)
:
from multiprocessing import Pool
n_cores = 4
pool = Pool(n_cores)
def apply_parallel(df, func):
df_split = np.array_split(df, n_cores)
df = pd.concat(pool.map(func, df_split))
return df
:

Pandarallel
Pandarallel — pandas, . , , + progress bar ;) , pandarallel.

. , . pandarallel — :
from pandarallel import pandarallel
pandarallel.initialize()
, — apply
parallel_aply
:
df['headline_text'].parallel_apply(mean_word_len)
:

- overhead 0.5 .
parallel_apply
, . 1 , , . - , , , 2-3 .
- Pandarallel
parallel_apply
(groupby
), .
, , . / , API progress bar.
To be continued
在这一部分中,我们研究了2种相当简单的熊猫优化方法- 使用jit编译和任务并行化。在接下来的部分,我会谈论更有趣,更复杂的工具,但现在我建议你测试自己的工具,以确保它们是有效的。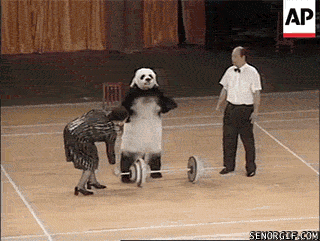
PS:信任,但请验证-本文中使用的所有代码(基准和图表),我已在github上发布了