眼动追踪市场预计将不断增长:从2020年的5.6亿美元增长到2025年的 1.786万亿美元。那么,相对昂贵的设备又有什么选择呢?当然,有一个简单的网络摄像头!与其他方法一样,这种方法也遇到很多困难,无论是:各种各样的设备(因此,很难选择在所有相机上都能正常使用的设置),参数的可变性强(从照明到相机倾斜及其相对于面部的位置),体面的计算力量(几个cuda核心和Xeon-就是这样)...
尽管要等一会儿,是否真的有必要在高端硬件上花钱甚至买显卡?也许有一种方法可以使所有计算都适合cpu,而不会同时损失速度?
(好吧,如果没有这种方法,就不会有文章介绍如何在PyTorch上训练神经元)
数据
与数据科学一样,最重要的问题。经过一段时间的搜索,我找到了MPIIGaze 数据集。本文的作者提出了许多很酷的方法来处理它(例如,标准化头部的位置),但是我们将采用简单的方法。
因此,启动Colab,加载笔记本电脑并启动:
import os
import numpy as np
import pandas as pd
import scipy
import scipy.io
from PIL import Image
import cv2
import seaborn as sns
import matplotlib
import matplotlib.pyplot as plt
在Colab中,您可以直接从笔记本电脑使用系统实用程序,进行播种,下载和解压缩数据集:
!wget https://datasets.d2.mpi-inf.mpg.de/MPIIGaze/MPIIGaze.tar.gz
!tar xvzf MPIIGaze.tar.gz MPIIGaze
Data/Original . 15, . Annotation Subset , — . header', , .
database_path = "/content/MPIIGaze"
def load_image_data(patient_name):
global database_path
annotation_path = os.path.join(database_path, "Annotation Subset", patient_name + ".txt")
data_folder = os.path.join(database_path, "Data", "Original", patient_name)
annotation = pd.read_csv(annotation_path, sep=" ", header=None)
points = np.array(annotation.loc[:, list(range(1, 17))])
filenames = np.array(annotation.loc[:, [0]]).reshape(-1)
images = [np.array(Image.open(os.path.join(data_folder, filename))) for filename in filenames]
return images, points
images, points = load_image_data("p00")
plt.imshow(images[0])
colors = ["r", "g", "b", "magenta", "y", "cyan", "brown", "lightcoral"]
for i in range(0, len(points[0]), 2):
x, y = points[0, i:i+2]
plt.scatter([x], [y], c=colors[i//2])
:
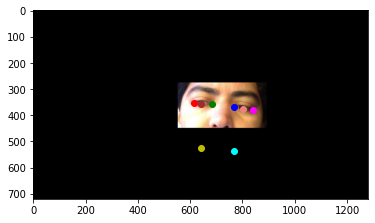
, : , , .
, . , : , (, , ), , 2:1. 2 1 , .
def distance(x1, y1, x2, y2):
return int(((x1 - x2) ** 2 + (y1 - y2) ** 2) ** 0.5)
image_shape = (16, 32)
def handle_eye(image, p1, p2, pupil):
global image_shape
line_len = distance(*p1, *p2)
p1 = p1[::-1]
p2 = p2[::-1]
pupil = pupil[::-1]
corner1 = p1 - np.array([line_len//4, 0])
corner2 = p2 + np.array([line_len//4, 0])
sub_image = image[corner1[0]:corner2[0]+1, corner1[1]:corner2[1]+1]
pupil_new = pupil - corner1
pupil_new = pupil_new / sub_image.shape[:2]
sub_image = cv2.resize(sub_image, image_shape[::-1], interpolation=cv2.INTER_AREA)
sub_image = cv2.cvtColor(sub_image, cv2.COLOR_RGB2GRAY)
return sub_image, pupil_new
2 , — :
def image_to_train_data(image, points):
eye_right_p1 = points[0:2]
eye_right_p2 = points[2:4]
eye_right_pupil = points[12:14]
right_image, right_pupil = handle_eye(image, eye_right_p1, eye_right_p2, eye_right_pupil)
eye_left_p1 = points[4:6]
eye_left_p2 = points[6:8]
eye_left_pupil = points[14:16]
left_image, left_pupil = handle_eye(image, eye_left_p1, eye_left_p2, eye_left_pupil)
return right_image, right_pupil, left_image, left_pupil
( ):
right_image, right_pupil, left_image, left_pupil = image_to_train_data(images[10], points[10])
plt.imshow(right_image, cmap="gray")
r_p_x = int(right_pupil[1] * image_shape[1])
r_p_y = int(right_pupil[0] * image_shape[0])
plt.scatter([r_p_x], [r_p_y], c="red")

, - . :
images_left_conc = []
images_right_conc = []
pupils_left_conc = []
pupils_right_conc = []
patients_path = os.path.join(database_path, "Data", "Original")
for patient in os.listdir(patients_path):
print(patient)
images, points = load_image_data(patient)
for i in range(len(images)):
signle_image_data = image_to_train_data(images[i], points[i])
if any(stuff is None for stuff in signle_image_data):
continue
right_image, right_pupil, left_image, left_pupil = signle_image_data
if any(right_pupil < 0) or any(left_pupil < 0):
continue
images_right_conc.append(right_image)
images_left_conc.append(left_image)
pupils_right_conc.append(right_pupil)
pupils_left_conc.append(left_pupil)
images_left_conc = np.array(images_left_conc)
images_right_conc = np.array(images_right_conc)
pupils_left_conc = np.array(pupils_left_conc)
pupils_right_conc = np.array(pupils_right_conc)
:
images_left_conc = images_left_conc / 255
images_right_conc = images_right_conc / 255
, : :
pupils_conc = np.zeros_like(pupils_left_conc)
for i in range(2):
pupils_conc[:, i] = (pupils_left_conc[:, i] + pupils_right_conc[:, i]) / 2
:
viz_pupils = np.zeros(image_shape)
for y, x in pupils_conc:
y = int(y * image_shape[0])
x = int(x * image_shape[1])
viz_pupils[y, x] += 1
max_val = viz_pupils.max()
viz_pupils = viz_pupils / max_val
plt.imshow(viz_pupils, cmap="hot")
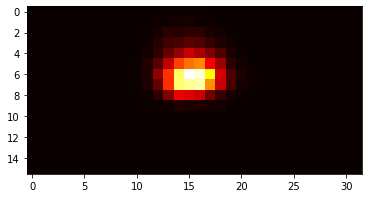
, .
from sklearn.model_selection import train_test_split
import torch
from torch.utils.data import DataLoader, TensorDataset
def make_2eyes_datasets(images_left, images_right, pupils, train_size=0.8):
n, height, width = images_left.shape
images_left = images_left.reshape(n, 1, height, width)
images_right = images_right.reshape(n, 1, height, width)
images_left_train, images_left_val, images_right_train, images_right_val, pupils_train, pupils_val = train_test_split(
images_left, images_right, pupils, train_size=train_size
)
def make_dataset(im_left, im_right, pups):
return TensorDataset(
torch.from_numpy(im_left.astype(np.float32)), torch.from_numpy(im_right.astype(np.float32)), torch.from_numpy(pups.astype(np.float32))
)
train_dataset = make_dataset(images_left_train, images_right_train, pupils_train)
val_dataset = make_dataset(images_left_val, images_right_val, pupils_val)
return train_dataset, val_dataset
def make_dataloaders(train_dataset, val_dataset, batch_size=256):
train_dataloader = DataLoader(train_dataset, batch_size=batch_size)
val_dataloader = DataLoader(val_dataset, batch_size=batch_size)
return train_dataloader, val_dataloader
batch_size = 256
eyes_datasets = make_2eyes_datasets(images_left_conc, images_right_conc, pupils_conc)
eyes_train_loader, eyes_val_loader = make_dataloaders(*eyes_datasets, batch_size=batch_size)
import torch
import torch.nn as nn
import torch.nn.functional as F
class Reshaper(nn.Module):
def __init__(self, target_shape):
super(Reshaper, self).__init__()
self.target_shape = target_shape
def forward(self, input):
return torch.reshape(input, (-1, *self.target_shape))
class EyesNet(nn.Module):
def __init__(self):
super(EyesNet, self).__init__()
self.features_left = nn.Sequential(
nn.Conv2d(in_channels=1, out_channels=32, kernel_size=5, stride=2, padding=2),
nn.LeakyReLU(),
nn.Conv2d(in_channels=32, out_channels=64, kernel_size=3, stride=2, padding=1),
nn.LeakyReLU(),
nn.Conv2d(in_channels=64, out_channels=64, kernel_size=3, stride=2, padding=1),
nn.LeakyReLU(),
nn.Conv2d(in_channels=64, out_channels=64, kernel_size=3, stride=2, padding=1),
nn.LeakyReLU(),
nn.Conv2d(in_channels=64, out_channels=64, kernel_size=3, stride=2, padding=1),
nn.LeakyReLU(),
Reshaper([64])
)
self.features_right = nn.Sequential(
nn.Conv2d(in_channels=1, out_channels=32, kernel_size=5, stride=2, padding=2),
nn.LeakyReLU(),
nn.Conv2d(in_channels=32, out_channels=64, kernel_size=3, stride=2, padding=1),
nn.LeakyReLU(),
nn.Conv2d(in_channels=64, out_channels=64, kernel_size=3, stride=2, padding=1),
nn.LeakyReLU(),
nn.Conv2d(in_channels=64, out_channels=64, kernel_size=3, stride=2, padding=1),
nn.LeakyReLU(),
nn.Conv2d(in_channels=64, out_channels=64, kernel_size=3, stride=2, padding=1),
nn.LeakyReLU(),
Reshaper([64])
)
self.fc = nn.Sequential(
nn.Linear(128, 64),
nn.LeakyReLU(),
nn.Linear(64, 16),
nn.LeakyReLU(),
nn.Linear(16, 2),
nn.Sigmoid()
)
def forward(self, x_left, x_right):
x_left = self.features_left(x_left)
x_right = self.features_right(x_right)
x = torch.cat((x_left, x_right), 1)
x = self.fc(x)
return x
, GPU ( CPU ≈ ), 8 .
def train(model, train_loader, test_loader, epochs, lr, folder="gazenet"):
os.makedirs(folder, exist_ok=True)
optimizer = torch.optim.Adam(model.parameters(), lr=lr)
mse = nn.MSELoss()
for epoch in range(epochs):
running_loss = 0
error_mean = []
error_std = []
for i, (*xs_batch, y_batch) in enumerate(train_loader):
xs_batch = [x_batch.cuda() for x_batch in xs_batch]
y_batch = y_batch.cuda()
optimizer.zero_grad()
y_batch_pred = model(*xs_batch)
loss = mse(y_batch_pred, y_batch)
loss.backward()
optimizer.step()
running_loss += loss.item()
difference = (y_batch - y_batch_pred).detach().cpu().numpy().reshape(-1)
error_mean.append(np.mean(difference))
error_std.append(np.std(difference))
error_mean = np.mean(error_mean)
error_std = np.mean(error_std)
print(f"Epoch {epoch+1}/{epochs}, train loss: {running_loss}, error mean: {error_mean}, error std: {error_std}")
running_loss = 0
error_mean = []
error_std = []
for i, (*xs_batch, y_batch) in enumerate(train_loader):
xs_batch = [x_batch.cuda() for x_batch in xs_batch]
y_batch = y_batch.cuda()
y_batch_pred = model(*xs_batch)
loss = mse(y_batch_pred, y_batch)
loss.backward()
running_loss += loss.item()
difference = (y_batch - y_batch_pred).detach().cpu().numpy().reshape(-1)
error_mean.append(np.mean(difference))
error_std.append(np.std(difference))
error_mean = np.mean(error_mean)
error_std = np.mean(error_std)
print(f"Epoch {epoch+1}/{epochs}, val loss: {running_loss}, error mean: {error_mean}, error std: {error_std}")
epoch_path = os.path.join(folder, f"epoch_{epoch+1}.pth")
torch.save(model.state_dict(), epoch_path)
eyesnet = EyesNet().cuda()
train(eyesnet, eyes_train_loader, eyes_val_loader, 300, 1e-3, "eyes_net")
, 300 ( , ):
Epoch 1/300, train loss: 0.3125856015831232, error mean: -0.019309822469949722, error std: 0.08668763190507889
Epoch 1/300, val loss: 0.18365296721458435, error mean: -0.008721884340047836, error std: 0.07283741235733032
Epoch 2/300, train loss: 0.1700970521196723, error mean: 0.0001489206333644688, error std: 0.07033108174800873
Epoch 2/300, val loss: 0.1475073655601591, error mean: -0.001808341359719634, error std: 0.06572529673576355
...
Epoch 299/300, train loss: 0.003378463063199888, error mean: -8.133996743708849e-05, error std: 0.009488753043115139
Epoch 299/300, val loss: 0.004163481352406961, error mean: -0.001996406354010105, error std: 0.010547727346420288
Epoch 300/300, train loss: 0.003569353237253381, error mean: -9.1125002654735e-05, error std: 0.00977678969502449
Epoch 300/300, val loss: 0.004456713928448153, error mean: 0.0008482271223329008, error std: 0.010923181660473347
299 , .
:
import random
def show_output(model, data_loader, batch_num=0, samples=5, grid_shape=(5, 1), figsize=(10, 10)):
for i, (*xs, y) in enumerate(data_loader):
if i == batch_num:
break
xs = [x.cuda() for x in xs]
y_pred = model(*xs).detach().cpu().numpy().reshape(-1, 2)
xs = [x.detach().cpu().numpy().reshape(-1, 16, 32) for x in xs]
imgs_conc = np.hstack(xs)
y = y.cpu().numpy().reshape(-1, 2)
indices = random.sample(range(len(y_pred)), samples)
fig, axes = plt.subplots(*grid_shape, figsize=figsize)
for i, index in enumerate(indices):
row = i // grid_shape[1]
column = i % grid_shape[1]
axes[row, column].imshow(imgs_conc[index])
axes[row, column].scatter([y_pred[index, 1]*32, y_pred[index, 1]*32], [y_pred[index, 0]*16, (y_pred[index, 0]+1)*16], c="r")
axes[row, column].scatter([y[index, 1]*32, y[index, 1]*32], [y[index, 0]*16, (y[index, 0]+1)*16], c="g")
eyesnet.load_state_dict(torch.load("eyes_net/epoch_299.pth"))
show_output(eyesnet, eyes_val_loader, 103, 16, (4, 4))
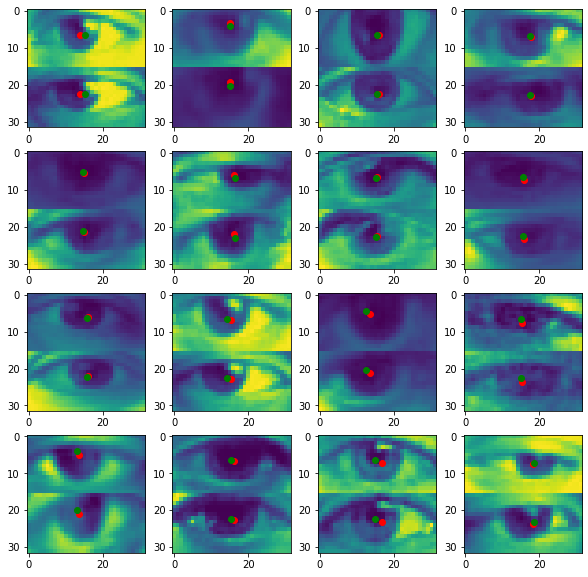
, , "" , . — -, - , -, . , .
( X Y), :
def error_distribution(model, data_loader, image_shape=(16, 32), bins=32, digits=2, figsize=(10,10)):
ys_true = []
ys_pred = []
for *xs, y in data_loader:
xs = [x.cuda() for x in xs]
y_pred = model(*xs)
ys_true.append(y.detach().cpu().numpy())
ys_pred.append(y_pred.detach().cpu().numpy())
ys_true = np.concatenate(ys_true)
ys_pred = np.concatenate(ys_pred)
indices = np.arange(len(ys_true))
fig, axes = plt.subplots(2, figsize=figsize)
for ax_num in range(2):
ys_true_subset = ys_true[:, ax_num]
ys_pred_subset = ys_pred[:, ax_num]
counts, ranges = np.histogram(ys_true_subset, bins=bins)
errors = []
labels = []
for i in range(len(counts)):
begin, end = ranges[i], ranges[i + 1]
range_indices = indices[(ys_true_subset >= begin) & (ys_true_subset <= end)]
diffs = np.abs(ys_pred_subset[range_indices] - ys_true_subset[range_indices])
label = (begin + end) / 2
if image_shape:
diffs = diffs * image_shape[ax_num]
label = label * image_shape[ax_num]
else:
label = round(label, digits)
errors.append(diffs)
labels.append(str(label)[:2+digits])
axes[ax_num].boxplot(errors, labels=labels)
if image_shape:
y_label = "difference, px"
x_label = "true position, px"
else:
y_label = "difference"
x_label = "true position"
axes[ax_num].set_ylabel(y_label)
axes[ax_num].set_xlabel(x_label)
if ax_num == 0:
title = "Y"
else:
title = "X"
axes[ax_num].set_title(title)
error_distribution(eyesnet, eyes_val_loader, figsize=(20, 10))

,
-, . , . :
import time
def measure_time(model, data_loader, n_batches=5):
begin_time = time.time()
batch_num = 0
n_samples = 0
predicted = []
for *xs, y in data_loader:
xs = [x.cpu() for x in xs]
y_pred = model(*xs)
predicted.append(y_pred.detach().cpu().numpy().reshape(-1))
batch_num += 1
n_samples += len(y)
if batch_num >= n_batches:
break
end_time = time.time()
time_per_sample = (end_time - begin_time) / n_samples
return time_per_sample
eyesnet_cpu = EyesNet().cpu()
eyesnet_cpu.load_state_dict(torch.load("eyes_net/epoch_299.pth", map_location="cpu"))
_, eyes_val_loader_single = make_dataloaders(*eyes_datasets, batch_size=1)
tps = measure_time(eyesnet_cpu, eyes_val_loader_single)
print(f"{tps} seconds per sample")
>>> 0.003347921371459961 seconds per sample
, VGG16 ( , ):
import torchvision.models as models
class VGG16Based(nn.Module):
def __init__(self):
super(VGG16Based, self).__init__()
self.vgg = models.vgg16(pretrained=False)
self.vgg.classifier = nn.Sequential(
nn.Linear(25088, 256),
nn.LeakyReLU(),
nn.Linear(256, 2),
nn.Sigmoid()
)
def forward(self, x_left, x_right):
x_mid = (x_left + x_right) / 2
x = torch.cat((x_left, x_mid, x_right), dim=1)
x_pad = torch.zeros((x.shape[0], 3, 32, 32))
x_pad[:, :, :16, :] = x
x = self.vgg(x_pad)
return x
vgg16 = VGG16Based()
vgg16_tps = measure_time(vgg16, eyes_val_loader_single)
print(f"{vgg16_tps} seconds per sample")
>>> 0.023713159561157226 seconds per sample
, (AMD A10-4600M APU, 1500 MHz):
python benchmark.py
0.003980588912963867 seconds per sample, EyesNet
0.12246298789978027 seconds per sample, VGG16-based
, , , ( VGG16 80 , EyesNet — 1 ; , , ). , , . , :
- ( ).
- . , float8 float32 ( , , ).
- PyTorch Mobile — PyTorch . .
- . — GazeCapture. , , — :
- TFLite — TensorFlow . !
, . Data science ( — *^*) . — FARADAY Lab. — , .
c:
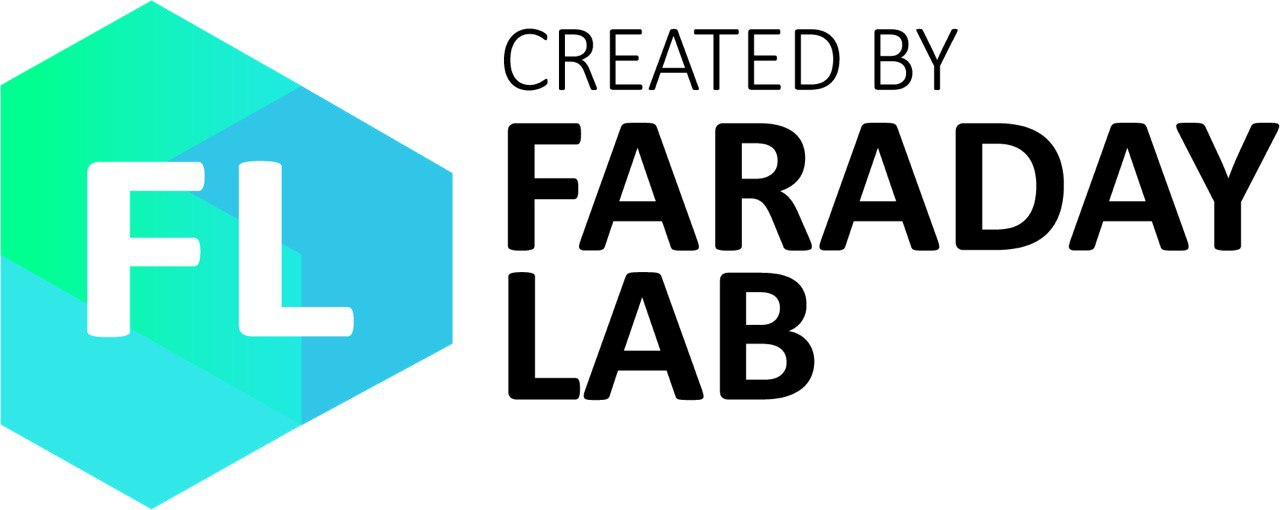
: