神经网络非常流行。它们的主要优势在于,它们能够概括其他算法显示质量较低的相当复杂的数据。但是,如果神经网络的质量仍然不能令人满意怎么办?
乐团来了...
什么是合奏
一组机器学习算法是使用多个(不一定不同)模型而不是一个模型。也就是说,我们首先训练每个模型,然后结合它们的预测。事实证明,我们的模型共同构成了一个更复杂的模型(就泛化能力而言,即“理解”数据的能力),通常称为元模型。通常,元模型不再在我们的初始数据样本上训练,而是在其他模型的预测上训练。它似乎考虑了所有模型的经验,这有助于减少错误。
计划
- 首先,我们看一个简单的PyTorch模型并获得其质量。
- 然后,我们将使用Scikit-learn组装几个模型,并学习如何达到更高的水平
一个模型
因此,我们将使用Sklearn的Digits数据集,因为可以通过两行代码非常简单地获取它:
from sklearn.datasets import load_digits
import numpy as np
import matplotlib.pyplot as plt
x, y = load_digits(n_class=10, return_X_y=True)
让我们看看数据如何:
print(x.shape)
>>> (1797, 64)
print(np.unique(y))
>>> array([0, 1, 2, 3, 4, 5, 6, 7, 8, 9])
index = 0
print(y[index])
plt.imshow(x[index].reshape(8, 8), cmap="Greys")
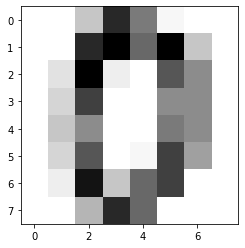
x[index]
>>> array([ 0., 0., 5., 13., 9., 1., 0., 0., 0., 0., 13., 15., 10.,
15., 5., 0., 0., 3., 15., 2., 0., 11., 8., 0., 0., 4.,
12., 0., 0., 8., 8., 0., 0., 5., 8., 0., 0., 9., 8.,
0., 0., 4., 11., 0., 1., 12., 7., 0., 0., 2., 14., 5.,
10., 12., 0., 0., 0., 0., 6., 13., 10., 0., 0., 0.])
是的,数字的取值范围是0到15.因此,我们需要归一化:
from sklearn.model_selection import train_test_split
from sklearn.preprocessing import StandardScaler
x_train, x_test, y_train, y_test = train_test_split(x, y, test_size=0.2,
shuffle=True, random_state=42)
print(x_train.shape)
>>> (1437, 64)
print(x_test.shape)
>>> (360, 64)
我们使用StandardScaler:它将所有数据从-1转移到-1的边界。我们仅在训练样本上对其进行训练,以使其不适应测试-因此测试后模型的得分将更接近真实数据的得分:
scaler = StandardScaler()
scaler.fit(x_train)
我们将数据标准化:
x_train_scaled = scaler.transform(x_train)
x_test_scaled = scaler.transform(x_test)
火炬的时间!
import torch
from torch.utils.data import TensorDataset, DataLoader
import torch.nn as nn
import torch.nn.functional as F
from torch.optim import Adam
一个简单的卷积网络足以证明:
class SimpleCNN(nn.Module):
def __init__(self):
super(SimpleCNN, self).__init__()
self.conv1 = nn.Conv2d(in_channels=1, out_channels=3, kernel_size=5, stride=1, padding=2)
self.conv1_s = nn.Conv2d(in_channels=3, out_channels=3, kernel_size=5, stride=2, padding=2)
self.conv2 = nn.Conv2d(in_channels=3, out_channels=6, kernel_size=3, stride=1, padding=1)
self.conv2_s = nn.Conv2d(in_channels=6, out_channels=6, kernel_size=3, stride=2, padding=1)
self.conv3 = nn.Conv2d(in_channels=6, out_channels=10, kernel_size=3, stride=1, padding=1)
self.conv3_s = nn.Conv2d(in_channels=10, out_channels=10, kernel_size=3, stride=2, padding=1)
self.flatten = nn.Flatten()
self.fc1 = nn.Linear(10, 10)
def forward(self, x):
x = F.relu(self.conv1(x))
x = F.relu(self.conv1_s(x))
x = F.relu(self.conv2(x))
x = F.relu(self.conv2_s(x))
x = F.relu(self.conv3(x))
x = F.relu(self.conv3_s(x))
x = self.flatten(x)
x = self.fc1(x)
x = F.softmax(x)
return x
定义一些超参数:
batch_size = 64
learning_rate = 1e-3
epochs = 200
首先,将数据转换为PyTorch使用的张量:
x_train_tensor = torch.tensor(x_train_scaled.reshape(-1, 1, 8, 8).astype(np.float32))
x_test_tensor = torch.tensor(x_test_scaled.reshape(-1, 1, 8, 8).astype(np.float32))
y_train_tensor = torch.tensor(y_train.astype(np.long))
y_test_tensor = torch.tensor(y_test.astype(np.long))
PyTorch , — . — , X y ( ), DataLoader — , <X, y> 64 :
train_dataset = TensorDataset(x_train_tensor, y_train_tensor)
train_loader = DataLoader(train_dataset, batch_size=batch_size)
test_dataset = TensorDataset(x_test_tensor, y_test_tensor)
test_loader = DataLoader(test_dataset, batch_size=batch_size)
, :
simple_cnn = SimpleCNN().cuda()
criterion = nn.CrossEntropyLoss()
optimizer = Adam(simple_cnn.parameters(), lr=learning_rate)
:
for epoch in range(epochs):
simple_cnn.train()
train_samples_count = 0
true_train_samples_count = 0
running_loss = 0
for batch in train_loader:
x_data = batch[0].cuda()
y_data = batch[1].cuda()
y_pred = simple_cnn(x_data)
loss = criterion(y_pred, y_data)
optimizer.zero_grad()
loss.backward()
optimizer.step()
running_loss += loss.item()
y_pred = y_pred.argmax(dim=1, keepdim=False)
true_classified = (y_pred == y_data).sum().item()
true_train_samples_count += true_classified
train_samples_count += len(x_data)
train_accuracy = true_train_samples_count / train_samples_count
print(f"[{epoch}] train loss: {running_loss}, accuracy: {round(train_accuracy, 4)}")
simple_cnn.eval()
test_samples_count = 0
true_test_samples_count = 0
running_loss = 0
for batch in test_loader:
x_data = batch[0].cuda()
y_data = batch[1].cuda()
y_pred = simple_cnn(x_data)
loss = criterion(y_pred, y_data)
loss.backward()
running_loss += loss.item()
y_pred = y_pred.argmax(dim=1, keepdim=False)
true_classified = (y_pred == y_data).sum().item()
true_test_samples_count += true_classified
test_samples_count += len(x_data)
test_accuracy = true_test_samples_count / test_samples_count
print(f"[{epoch}] test loss: {running_loss}, accuracy: {round(test_accuracy, 4)}")
,- Torch' , . . 20 .
[180] train loss: 40.52328181266785, accuracy: 0.6966
[180] test loss: 10.813781499862671, accuracy: 0.6583
[181] train loss: 40.517325043678284, accuracy: 0.6966
[181] test loss: 10.811877608299255, accuracy: 0.6611
[182] train loss: 40.517088294029236, accuracy: 0.6966
[182] test loss: 10.814386487007141, accuracy: 0.6611
[183] train loss: 40.515315651893616, accuracy: 0.6966
[183] test loss: 10.812204122543335, accuracy: 0.6611
[184] train loss: 40.5108939409256, accuracy: 0.6966
[184] test loss: 10.808713555335999, accuracy: 0.6639
[185] train loss: 40.50885498523712, accuracy: 0.6966
[185] test loss: 10.80833113193512, accuracy: 0.6639
[186] train loss: 40.50892996788025, accuracy: 0.6966
[186] test loss: 10.809209108352661, accuracy: 0.6639
[187] train loss: 40.508036971092224, accuracy: 0.6966
[187] test loss: 10.806900978088379, accuracy: 0.6667
[188] train loss: 40.507275462150574, accuracy: 0.6966
[188] test loss: 10.79791784286499, accuracy: 0.6611
[189] train loss: 40.50368785858154, accuracy: 0.6966
[189] test loss: 10.799399137496948, accuracy: 0.6667
[190] train loss: 40.499858379364014, accuracy: 0.6966
[190] test loss: 10.795265793800354, accuracy: 0.6611
[191] train loss: 40.498780846595764, accuracy: 0.6966
[191] test loss: 10.796114206314087, accuracy: 0.6639
[192] train loss: 40.497228503227234, accuracy: 0.6966
[192] test loss: 10.790620803833008, accuracy: 0.6639
[193] train loss: 40.44325613975525, accuracy: 0.6973
[193] test loss: 10.657087206840515, accuracy: 0.7
[194] train loss: 39.62049174308777, accuracy: 0.7495
[194] test loss: 10.483307123184204, accuracy: 0.7222
[195] train loss: 39.24516046047211, accuracy: 0.7613
[195] test loss: 10.462445378303528, accuracy: 0.7278
[196] train loss: 39.16947162151337, accuracy: 0.762
[196] test loss: 10.488057255744934, accuracy: 0.7222
[197] train loss: 39.196797251701355, accuracy: 0.7634
[197] test loss: 10.502906918525696, accuracy: 0.7222
[198] train loss: 39.395434617996216, accuracy: 0.7537
[198] test loss: 10.354896545410156, accuracy: 0.7472
[199] train loss: 39.331292152404785, accuracy: 0.7509
[199] test loss: 10.367400050163269, accuracy: 0.7389
, : .
, : 74% . Not great, not terrible.
!
Bagging. : , , . : "" , - . .
sklearn ensembles
, . — , BaggingClassifier
:
import sklearn
from sklearn.ensemble import BaggingClassifier
. sklearn, — , sklearn.base.BaseEstimator
. , . fit
( ), predict_proba
, ( , ), predict
( ), .
, "" .
. , , .
-, ( 2 — get_params
set_params
), , . , net_type
, __init__
net_type
.
-, , , ( sklearn , ) copy
, deepcopy
. , , () , , .
class PytorchModel(sklearn.base.BaseEstimator):
def __init__(self, net_type, net_params, optim_type, optim_params, loss_fn,
input_shape, batch_size=32, accuracy_tol=0.02, tol_epochs=10,
cuda=True):
self.net_type = net_type
self.net_params = net_params
self.optim_type = optim_type
self.optim_params = optim_params
self.loss_fn = loss_fn
self.input_shape = input_shape
self.batch_size = batch_size
self.accuracy_tol = accuracy_tol
self.tol_epochs = tol_epochs
self.cuda = cuda
: fit
. , — , Loss, . : , ? . , 200 ( ). , . — , accuracy . , accuracy . , (tol_epochs
) ( accuracy , accuracy_tol
).
def fit(self, X, y):
self.net = self.net_type(**self.net_params)
if self.cuda:
self.net = self.net.cuda()
self.optim = self.optim_type(self.net.parameters(), **self.optim_params)
uniq_classes = np.sort(np.unique(y))
self.classes_ = uniq_classes
X = X.reshape(-1, *self.input_shape)
x_tensor = torch.tensor(X.astype(np.float32))
y_tensor = torch.tensor(y.astype(np.long))
train_dataset = TensorDataset(x_tensor, y_tensor)
train_loader = DataLoader(train_dataset, batch_size=self.batch_size,
shuffle=True, drop_last=False)
last_accuracies = []
epoch = 0
keep_training = True
while keep_training:
self.net.train()
train_samples_count = 0
true_train_samples_count = 0
for batch in train_loader:
x_data, y_data = batch[0], batch[1]
if self.cuda:
x_data = x_data.cuda()
y_data = y_data.cuda()
y_pred = self.net(x_data)
loss = self.loss_fn(y_pred, y_data)
self.optim.zero_grad()
loss.backward()
self.optim.step()
y_pred = y_pred.argmax(dim=1, keepdim=False)
true_classified = (y_pred == y_data).sum().item()
true_train_samples_count += true_classified
train_samples_count += len(x_data)
train_accuracy = true_train_samples_count / train_samples_count
last_accuracies.append(train_accuracy)
if len(last_accuracies) > self.tol_epochs:
last_accuracies.pop(0)
if len(last_accuracies) == self.tol_epochs:
accuracy_difference = max(last_accuracies) - min(last_accuracies)
if accuracy_difference <= self.accuracy_tol:
keep_training = False
. — , Loss, - :
def predict_proba(self, X, y=None):
X = X.reshape(-1, *self.input_shape)
x_tensor = torch.tensor(X.astype(np.float32))
if y:
y_tensor = torch.tensor(y.astype(np.long))
else:
y_tensor = torch.zeros(len(X), dtype=torch.long)
test_dataset = TensorDataset(x_tensor, y_tensor)
test_loader = DataLoader(test_dataset, batch_size=self.batch_size,
shuffle=False, drop_last=False)
self.net.eval()
predictions = []
for batch in test_loader:
x_data, y_data = batch[0], batch[1]
if self.cuda:
x_data = x_data.cuda()
y_data = y_data.cuda()
y_pred = self.net(x_data)
predictions.append(y_pred.detach().cpu().numpy())
predictions = np.concatenate(predictions)
return predictions
:
def predict(self, X, y=None):
predictions = self.predict_proba(X, y)
predictions = predictions.argmax(axis=1)
return predictions
class PytorchModel(sklearn.base.BaseEstimator):
def __init__(self, net_type, net_params, optim_type, optim_params, loss_fn,
input_shape, batch_size=32, accuracy_tol=0.02, tol_epochs=10,
cuda=True):
self.net_type = net_type
self.net_params = net_params
self.optim_type = optim_type
self.optim_params = optim_params
self.loss_fn = loss_fn
self.input_shape = input_shape
self.batch_size = batch_size
self.accuracy_tol = accuracy_tol
self.tol_epochs = tol_epochs
self.cuda = cuda
def fit(self, X, y):
self.net = self.net_type(**self.net_params)
if self.cuda:
self.net = self.net.cuda()
self.optim = self.optim_type(self.net.parameters(), **self.optim_params)
uniq_classes = np.sort(np.unique(y))
self.classes_ = uniq_classes
X = X.reshape(-1, *self.input_shape)
x_tensor = torch.tensor(X.astype(np.float32))
y_tensor = torch.tensor(y.astype(np.long))
train_dataset = TensorDataset(x_tensor, y_tensor)
train_loader = DataLoader(train_dataset, batch_size=self.batch_size,
shuffle=True, drop_last=False)
last_accuracies = []
epoch = 0
keep_training = True
while keep_training:
self.net.train()
train_samples_count = 0
true_train_samples_count = 0
for batch in train_loader:
x_data, y_data = batch[0], batch[1]
if self.cuda:
x_data = x_data.cuda()
y_data = y_data.cuda()
y_pred = self.net(x_data)
loss = self.loss_fn(y_pred, y_data)
self.optim.zero_grad()
loss.backward()
self.optim.step()
y_pred = y_pred.argmax(dim=1, keepdim=False)
true_classified = (y_pred == y_data).sum().item()
true_train_samples_count += true_classified
train_samples_count += len(x_data)
train_accuracy = true_train_samples_count / train_samples_count
last_accuracies.append(train_accuracy)
if len(last_accuracies) > self.tol_epochs:
last_accuracies.pop(0)
if len(last_accuracies) == self.tol_epochs:
accuracy_difference = max(last_accuracies) - min(last_accuracies)
if accuracy_difference <= self.accuracy_tol:
keep_training = False
def predict_proba(self, X, y=None):
X = X.reshape(-1, *self.input_shape)
x_tensor = torch.tensor(X.astype(np.float32))
if y:
y_tensor = torch.tensor(y.astype(np.long))
else:
y_tensor = torch.zeros(len(X), dtype=torch.long)
test_dataset = TensorDataset(x_tensor, y_tensor)
test_loader = DataLoader(test_dataset, batch_size=self.batch_size,
shuffle=False, drop_last=False)
self.net.eval()
predictions = []
for batch in test_loader:
x_data, y_data = batch[0], batch[1]
if self.cuda:
x_data = x_data.cuda()
y_data = y_data.cuda()
y_pred = self.net(x_data)
predictions.append(y_pred.detach().cpu().numpy())
predictions = np.concatenate(predictions)
return predictions
def predict(self, X, y=None):
predictions = self.predict_proba(X, y)
predictions = predictions.argmax(axis=1)
return predictions
, :
base_model = PytorchModel(net_type=SimpleCNN, net_params=dict(), optim_type=Adam,
optim_params={"lr": 1e-3}, loss_fn=nn.CrossEntropyLoss(),
input_shape=(1, 8, 8), batch_size=32, accuracy_tol=0.02,
tol_epochs=10, cuda=True)
base_model.fit(x_train_scaled, y_train)
preds = base_model.predict(x_test_scaled)
true_classified = (preds == y_test).sum()
test_accuracy = true_classified / len(y_test)
print(f"Test accuracy: {test_accuracy}")
>>> Test accuracy: 0.7361111111111112
.
meta_classifier = BaggingClassifier(base_estimator=base_model, n_estimators=10)
meta_classifier.fit(x_train_scaled.reshape(-1, 64), y_train)
>>> BaggingClassifier(
base_estimator=PytorchModel(accuracy_tol=0.02, batch_size=32,
cuda=True, input_shape=(1, 8, 8),
loss_fn=CrossEntropyLoss(),
net_params={},
net_type=<class '__main__.SimpleCNN'>,
optim_params={'lr': 0.001},
optim_type=<class 'torch.optim.adam.Adam'>,
tol_epochs=10),
bootstrap=True, bootstrap_features=False, max_features=1.0,
max_samples=1.0, n_estimators=10, n_jobs=None,
oob_score=False, random_state=None, verbose=0,
warm_start=False)
score
accuracy :
print(meta_classifier.score(x_test_scaled.reshape(-1, 64), y_test))
>>> 0.95
, . . . , , ( "" - ).
?
-, . , Loss' - .
-, Boosting. Bagging' , , . ( ) . sklearn, Loss - .
, . , "" . , .
, . — - , , , , , .
. , Data science' . Computer vision, . ( — !) — FARADAY Lab. — , .
c: