OlĂĄ Habr! De repente, percebemos que nosso Ășltimo artigo sobre Elbrus saiu um ano atrĂĄs. Portanto, decidimos corrigir essa supervisĂŁo irritante, porque nĂŁo abandonamos esse tĂłpico!
Ă difĂcil imaginar o reconhecimento sem redes neurais, portanto, falaremos sobre como lançamos grades de 8 bits no Elbrus e o que aconteceu com ele. Em geral, um modelo com coeficientes e entradas de 8 bits e cĂĄlculos intermediĂĄrios de 32 bits Ă© extremamente popular. Por exemplo, o Google [1] e o Facebook [2] lançaram suas prĂłprias implementaçÔes que otimizam o acesso Ă memĂłria, usam o SIMD e permitem acelerar os cĂĄlculos em 25% ou mais sem uma diminuição perceptĂvel na precisĂŁo (isso obviamente depende da arquitetura da rede neural e da calculadora, mas vocĂȘ precisa foi explicado como Ă© legal?).

A idĂ©ia de substituir nĂșmeros reais por nĂșmeros inteiros para acelerar os cĂĄlculos estĂĄ no ar:
- . , ââ , float , ;
- . - , , ⊠, . , (SIMD). c 128- SIMD 4 floatâ 16 uint8. 4 , ;
- , . â !
8- [3] . , , .
8- , 16-, . 32- . , 8- . , .
, â . (. . 1) :
â , â , â , â .
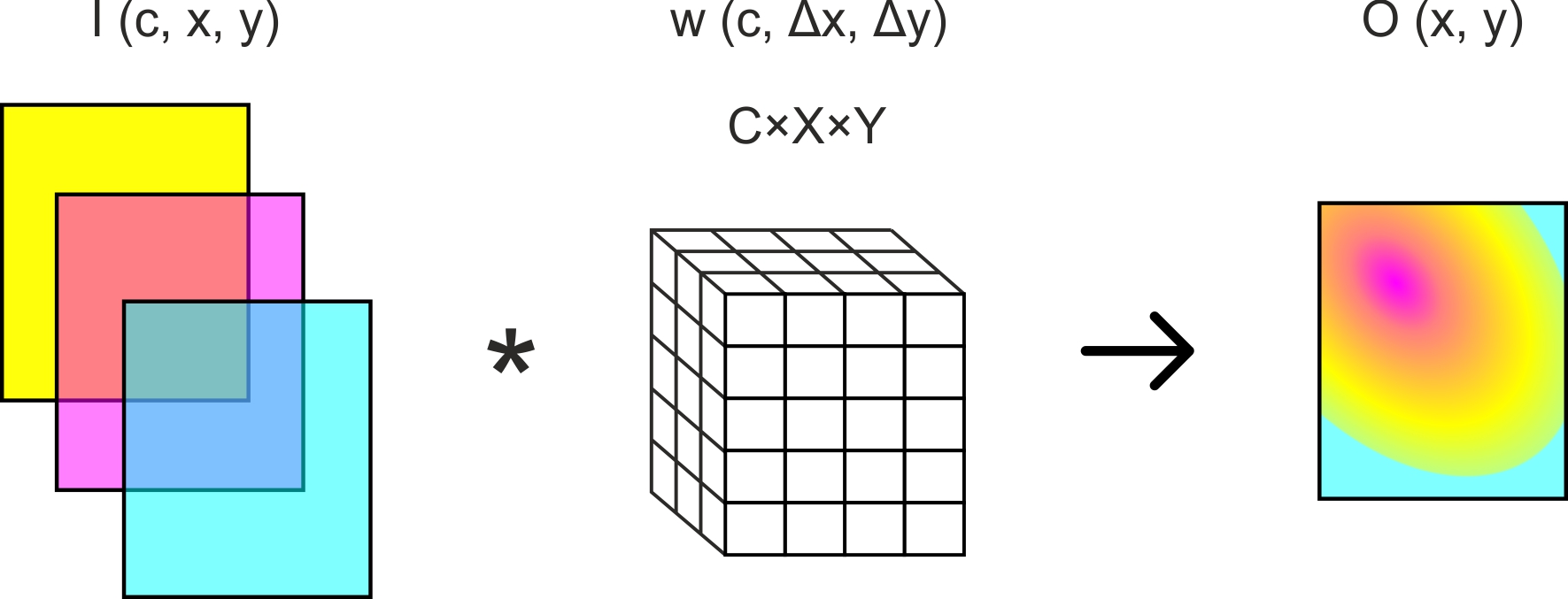
. 1. C x X x Y.
â [4]. , , . , "" â .. , . , .. (1). "" , .. ( ), ..
8- 32- .
- , , .
â , . , , â . . , , , [5-7].
6 ( ), . : - , . , , , , , . , .
. 1 (). 32 .
1. . "/" , , .
, - APB (array prefetch buffer). APB n- , . -, , APB , . APB :
, APB :
, , . , . Goto [8] . , , , . .
, , (. . 2-3). â . , .. . , APB - : , 8 ( 64- ) 2 .
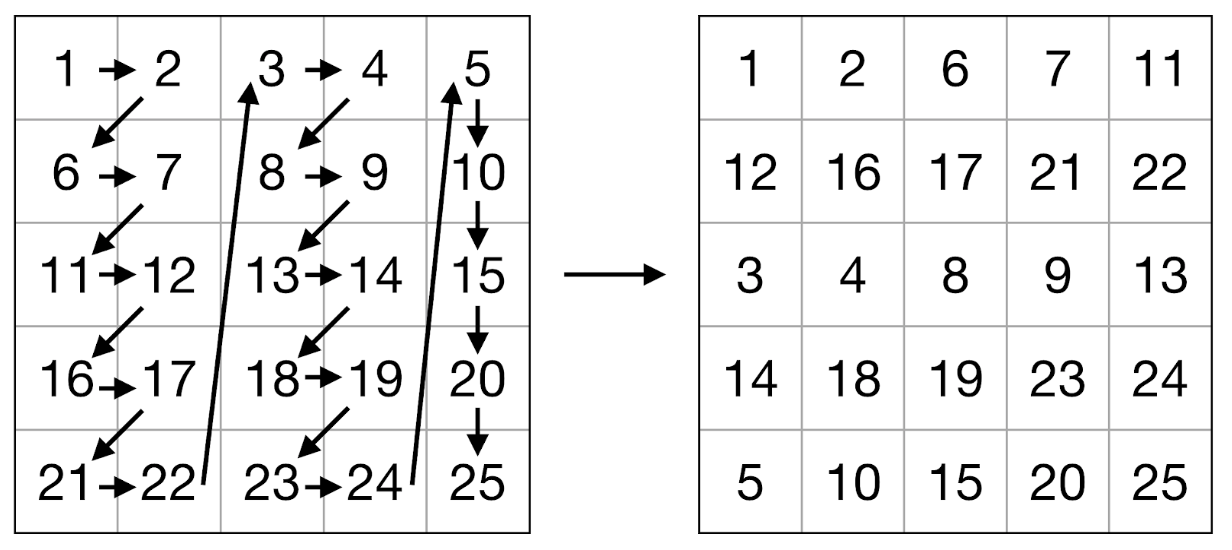
. 2. .

3. .
:
for bl_r in block_r(rhs):
packed_r <- pack_rhs(bl_r)
for bl_l in block_l(lhs):
packed_l <- pack_lhs(bl_l)
packed_res <- pack_res(bl_res)
kernel(packed_res, packed_l, packed_r)
bl_res <- unpack_res(packed_res)
lhs
rhs
â , block_l(.)
, block_r(.)
â , lhs
rhs
. pack_rhs
pack_lhs
, pack_res
â , unpack_res
â . kernel
.
kernel
:
for j in {0, ..., cols / nr}
{dst0, dst1} <-
for i in {0, ..., rows / mr}
for k in {0, ..., depth / 2}
bl_r <-
bl_l <-
lhs <- pshufb(zero, bl_l, 0x0901080009010800LL)
rhs0 <- punpcklbh(zero, bl_r)
rhs1 <- punpckhbh(zero, bl_r)
dst0 <- dst0 + pmaddh(rhs0, lhs)
dst1 <- dst1 + pmaddh(rhs1, lhs);
//
pshufb
â , ( ), punpckhbh
â , 16- , punpcklbh
â , 16- , pmaddh
â , 16- .
. nr mr = 12 8.
, 8 48 . 8 3, 14 , â 48 +, 8 .
2 8- -4, EML. N = 10^6
.
2. EML .
, -4 . , .
8- , x86 ARM, . , , , . 8- . , , ( , x86 ARM), : 6 64- , (64 128 ) 2 6 .
, 8- 32- . 3 (-4), 4 5 (-8 -8).
? , . , ââ , . - , . , , / .
- 8- ? , , , - ( ).
P.S.
Limonova E. E., Neyman-Zade M. I., Arlazarov V. L. Special aspects of matrix operation implementations for low-precision neural network model on the Elbrus platform // . . â 2020. â . 13. â â 1. â . 118-128. â DOI: 10.14529/mmp200109.
- https://github.com/google/gemmlowp
- https://engineering.fb.com/ml-applications/qnnpack/
- Vanhoucke, Vincent, Andrew Senior, and Mark Z. Mao. "Improving the speed of neural networks on CPUs." (2011).
- K. Chellapilla, S. Puri, P. Simard. High Performance Convolutional Neural Networks for Document Processing // Tenth International Workshop on Frontiers in Handwriting Recognition. â Universite de Rennes, 1 Oct 2006. â La Baule (France). â 2006.
- .., . ., . . ââ. â .: , â 2013. â 272 c.
- , .. / .. , .. , .. // - . â .: - ââ . . .. . â 2015. â No4 (8). â cc. 64-68.
- Limonova E. E., Skoryukina N. S., Neyman-Zade M. I. Fast Hamming Distance Computation for 2D Art Recognition on VLIW-Architecture in Case of Elbrus Platform // ICMV 2018 / SPIE. 2019. . 11041. ISSN 0277-786X. ISBN 978-15-10627-48-2. 2019. . 11041. 110411N. DOI: 10.1117/12.2523101
- Goto, K. Anatomy of high-performance matrix multiplication / K. Goto, R.A. Geijn // ACM Transactions on Mathematical Software (TOMS) â 2008. â 34(3). â p.12.