Neste pequeno exemplo, quero mostrar como analisar dados de sites e como usá-los ainda mais para análise. Para fazer isso, analisei a tabela de classificações de clãs do jogo World of Tanks e verifiquei como a classificação de clãs pode se correlacionar com outros dados.
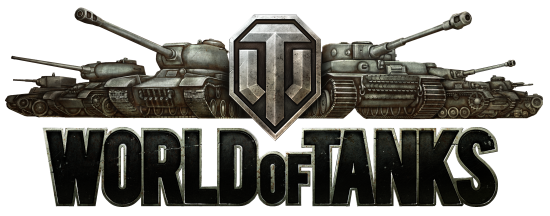
1. Analisando Dados
import numpy as np
import pandas as pd
from scrapy.selector import Selector
import matplotlib.pyplot as plt
import seaborn as sns
%matplotlib inline
sns.set(rc={'figure.figsize':(20, 5)})
javascript , - scrapy html , ( selenium) scrapy Selector
.
with open(' _ Wargaming.net.html', 'r') as f:
html_text = f.read()
selector = Selector(text=html_text)
xpath
- youtube.com, . , .. (. ), , text
, ( ), , , .
df = pd.DataFrame()
table = selector.xpath('//div[@class="js-widget-content"]/div[2]/div[2]/div') + selector.xpath('//div[@class="js-widget-content"]/div[2]/div[4]/div')
for row in table:
text = row.xpath('*//text()').extract()
clan = text[-10]
cr = int(text[-7].replace(' ',''))
wpr = int(text[-6].replace(' ',''))
abd = float(text[-5].replace(',', '.'))
avl_10 = int(text[-4])
fsh = int(text[-3].replace(' ',''))
wgm = int(text[-2].replace(' ',''))
wsh = int(text[-1].replace(' ',''))
df = df.append({'Clan' : clan,
'CR' : cr,
'wPR' : wpr,
'aB_D' : abd,
'aVL10' : avl_10,
'fSH' : fsh,
'wGM' : wgm,
'wSH' : wsh},
ignore_index=True)
df
, 25, 5 .
print('- :', len(df))
- : 25
df.head()
| CR | Clan | aB_D | aVL10 | fSH | wGM | wPR | wSH |
---|
0 | 15486.0 | [CM-1] | 18.20 | 34.0 | 4083.0 | 2253.0 | 10325.0 | 2294.0 |
---|
1 | 15148.0 | [R-BOY] | 18.86 | 37.0 | 3745.0 | 1943.0 | 10267.0 | 2066.0 |
---|
2 | 15041.0 | [CYS] | 17.47 | 32.0 | 3649.0 | 2300.0 | 10251.0 | 1857.0 |
---|
3 | 14984.0 | [I-YAN] | 16.85 | 28.0 | 4080.0 | 2468.0 | 8992.0 | 2290.0 |
---|
4 | 14952.0 | [YETT1] | 17.41 | 29.0 | 4222.0 | 2159.0 | 8387.0 | 2474.0 |
---|
2.
, - , . .
plt.xticks(rotation=45, ha="right")
ax = sns.lineplot(x='Clan', y='CR', data=df, marker='o', color='r', sort=False)
ax.set(xlabel='', ylabel=' ')
ax.set(xticks=df['Clan'].values);

. , ?
def draw_corr(df, y1, y1_label, y2='CR', y2_label=' '):
fig, ax = plt.subplots()
plt.xticks(rotation=45, ha="right")
sns.lineplot(x='Clan', y=y1, data=df, marker='o', color='b', label=y1_label, sort=False)
ax.set(xlabel='', ylabel=y1_label)
plt.legend(bbox_to_anchor=(0.01, 0.95), loc='upper left')
ax2 = ax.twinx()
sns.lineplot(x='Clan', y=y2, data=df, marker='o', color='r', label=y2_label, sort=False)
ax2.set(ylabel=y2_label)
plt.legend(bbox_to_anchor=(0.01, 0.85), loc='upper left');
draw_corr(df, 'wPR', ' ')

draw_corr(df, 'aVL10', ' ')

3.
Este artigo foi especialmente escrito simplesmente para mostrar que não há nada complicado na análise e análise de dados. A partir dos dados obtidos, é possível fazer mais gráficos de correlação, construir histogramas, se necessário, fazer algumas previsões, etc.
→ Código Fonte