O treinamento de redes neurais no reconhecimento de padrões é um processo longo e com muitos recursos. Especialmente quando há apenas um laptop barato, e não um computador com uma poderosa placa gráfica. Nesse caso, o Google Colaboratory virá em socorro , que oferece o uso gratuito da GPU de nível Tesla K80 ( mais ).
Este artigo descreve o processo de preparação de dados, treinamento do modelo de tensorflow no Google Colaboratory e seu lançamento no dispositivo Android.
Preparação de dados
Como exemplo, vamos tentar treinar uma rede neural para reconhecer dados brancos em um fundo preto. Assim, para iniciantes, você precisa criar um conjunto de dados suficiente para o treinamento (por enquanto, vamos parar em ~ 100 fotos).

Para treinamento, usaremos a API de detecção de objetos do Tensorflow . Prepararemos todos os dados necessários para o treinamento em um laptop. Precisamos de um ambiente conda e gerente de gerenciamento de dependências . Instruções de instalação aqui .
Vamos criar um ambiente para o trabalho:
conda create -n object_detection_prepare pip python=3.6
E ative-o:
conda activate object_detection_prepare
Defina as dependências que precisamos:
pip install --ignore-installed --upgrade tensorflow==1.14
pip install --ignore-installed pandas
pip install --ignore-installed Pillow
pip install lxml
conda install pyqt=5
object_detection, object_detection/images.
Google Colab , , "tcmalloc: large alloc....".
object_detection/preprocessing .
:
python ./object_detection/preprocessing/image_resize.py -i ./object_detection/images --imageWidth=800 --imageHeight=600
, 800x600 object_detection/images/resized. object_detection/images.
labelImg.
labelImg object_detection
labelImg
cd [FULL_PATH]/object_detection/labelImg
:
pyrcc5 -o libs/resources.py resources.qrc
( ):
python labelImg.py

“Open dir” object_detection/images , . (1, 2, 3, 4, 5, 6). ( *.xml) .
object_detection/training_demo, Google Colab .
( ) 80/20 object_detection/training_demo/images/train object_detection/training_demo/images/test.
object_detection/training_demo/annotations, . label_map.pbtxt, . :
label_map.pbtxtitem {
id: 1
name: '1'
}
item {
id: 2
name: '2'
}
item {
id: 3
name: '3'
}
item {
id: 4
name: '4'
}
item {
id: 5
name: '5'
}
item {
id: 6
name: '6'
}
, ? , TFRecord. [1].
: xml -> csv csv -> record
preprocessing :
cd [FULL_PATH]\object_detection\preprocessing
1. xml csv
:
python xml_to_csv.py -i [FULL_PATH]/object_detection/training_demo/images/train -o [FULL_PATH]/object_detection/training_demo/annotations/train_labels.csv
:
python xml_to_csv.py -i [FULL_PATH]/object_detection/training_demo/images/test -o [FULL_PATH]/object_detection/training_demo/annotations/test_labels.csv
2. csv record
:
python generate_tfrecord.py --label_map_path=[FULL_PATH]\object_detection\training_demo\annotations\label_map.pbtxt --csv_input=[FULL_PATH]\object_detection\training_demo\annotations\train_labels.csv --output_path=[FULL_PATH]\object_detection\training_demo\annotations\train.record --img_path=[FULL_PATH]\object_detection\training_demo\images\train
:
python generate_tfrecord.py --label_map_path=[FULL_PATH]\object_detection\training_demo\annotations\label_map.pbtxt --csv_input=[FULL_PATH]\object_detection\training_demo\annotations\test_labels.csv --output_path=[FULL_PATH]\object_detection\training_demo\annotations\test.record --img_path=[FULL_PATH]\object_detection\training_demo\images\test
, , .
.
ssdlite_mobilenet_v2_coco, android .
object_detection/training_demo/pre-trained-model.
-
object_detection/training_demo/pre-trained-model/ssdlite_mobilenet_v2_coco_2018_05_09
pipeline.config object_detection/training_demo/training ssdlite_mobilenet_v2_coco.config.
, :
1.
model.ssd.num_classes: 6
2. ( ), ,
train_config.batch_size: 18
train_config.num_steps: 20000
train_config.fine_tune_checkpoint:"./training_demo/pre-trained-model/ssdlite_mobilenet_v2_coco_2018_05_09/model.ckpt"
3. (object_detection/training_demo/images/train)
eval_config.num_examples: 64
4.
train_input_reader.label_map_path: "./training_demo/annotations/label_map.pbtxt"
train_input_reader.tf_record_input_reader.input_path:"./training_demo/annotations/train.record"
5.
eval_input_reader.label_map_path: "./training_demo/annotations/label_map.pbtxt"
eval_input_reader.tf_record_input_reader.input_path:"./training_demo/annotations/test.record"
, .
training_demo training_demo.zip Google Drive.
google drive Google Colab,
, .
Google Drive training_demo.zip, Get shareable link id :
drive.google.com/open?id=[YOUR_FILE_ID_HERE]
Google Colab — Google Drive.

CPU. GPU, runtime.


.
:
1. TensorFlow Models:
!git clone https://github.com/tensorflow/models.git
2. protobuf object_detection:
!apt-get -qq install libprotobuf-java protobuf-compiler
%cd ./models/research/
!protoc object_detection/protos/*.proto --python_out=.
%cd ../..
3. PYTHONPATH:
import os
os.environ['PYTHONPATH'] += ":/content/models/research/"
os.environ['PYTHONPATH'] += ":/content/models/research/slim"
os.environ['PYTHONPATH'] += ":/content/models/research/object_detection"
os.environ['PYTHONPATH'] += ":/content/models/research/object_detection/utils"
4. Google Drive PyDrive :
!pip install -U -q PyDrive
from pydrive.auth import GoogleAuth
from pydrive.drive import GoogleDrive
from google.colab import auth
from oauth2client.client import GoogleCredentials
auth.authenticate_user()
gauth = GoogleAuth()
gauth.credentials = GoogleCredentials.get_application_default()
drive = GoogleDrive(gauth)
5. ( id ) :
drive_file_id="[YOUR_FILE_ID_HERE]"
training_demo_zip = drive.CreateFile({'id': drive_file_id})
training_demo_zip.GetContentFile('training_demo.zip')
!unzip training_demo.zip
!rm training_demo.zip
6. :
!python ./models/research/object_detection/legacy/train.py --logtostderr --train_dir=./training_demo/training --pipeline_config_path=./training_demo/training/ssdlite_mobilenet_v2_coco.config
--train_dir=./training_demo/training — ,
--pipeline_config_path=./training_demo/training/ssdlite_mobilenet_v2_coco.config —
7. frozen graph, :
!python /content/models/research/object_detection/export_inference_graph.py --input_type image_tensor --pipeline_config_path /content/training_demo/training/ssdlite_mobilenet_v2_coco.config --trained_checkpoint_prefix /content/training_demo/training/model.ckpt-[CHECKPOINT_NUMBER]
--output_directory /content/training_demo/training/output_inference_graph_v1.pb
--pipeline_config_path /content/training_demo/training/ssdlite_mobilenet_v2_coco.config —
--trained_checkpoint_prefix /content/training_demo/training/model.ckpt-[CHECKPOINT_NUMBER] — , .
--output_directory /content/training_demo/training/output_inference_graph_v1.pb —
[CHECKPOINT_NUMBER], content/training_demo/training/. model.ckpt-1440.index, model.ckpt-1440.meta. 1440 — [CHECKPOINT_NUMBER] .

. ~20000 .

8. tflite.
tensorflow lite tflite. frozen graph tflite ( export_inference_graph.py):
!python /content/models/research/object_detection/export_tflite_ssd_graph.py --pipeline_config_path /content/training_demo/training/ssdlite_mobilenet_v2_coco.config --trained_checkpoint_prefix /content/training_demo/training/model.ckpt-[CHECKPOINT_NUMBER] --output_directory /content/training_demo/training/output_inference_graph_tf_lite.pb
tflite , output_inference_graph_tf_lite.pb:

Netron. .
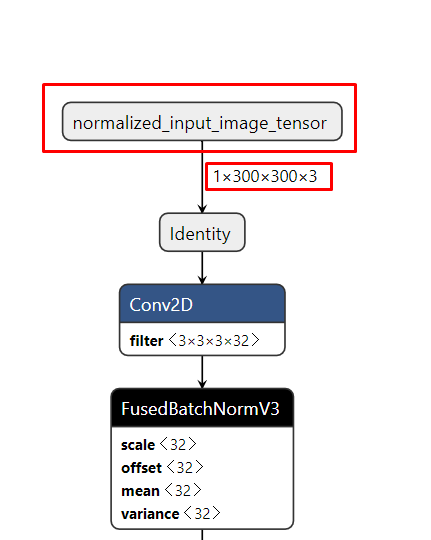

pb tflite :
!tflite_convert --output_file=/content/training_demo/training/model_q.tflite --graph_def_file=/content/training_demo/training/output_inference_graph_tf_lite_v1.pb/tflite_graph.pb --input_arrays=normalized_input_image_tensor --output_arrays='TFLite_Detection_PostProcess','TFLite_Detection_PostProcess:1','TFLite_Detection_PostProcess:2','TFLite_Detection_PostProcess:3' --input_shapes=1,300,300,3 --enable_select_tf_ops --allow_custom_ops --inference_input_type=QUANTIZED_UINT8 --inference_type=FLOAT --mean_values=128 --std_dev_values=128
--output_file=/content/training_demo/training/model_q.tflite —
--graph_def_file=/content/training_demo/training/output_inference_graph_tf_lite_v1.pb/tflite_graph.pb — frozen graph,
--input_arrays=normalized_input_image_tensor — ,
--output_arrays='TFLite_Detection_PostProcess','TFLite_Detection_PostProcess:1','TFLite_Detection_PostProcess:2','TFLite_Detection_PostProcess:3' — ,
--input_shapes=1,300,300,3 — ,
--enable_select_tf_ops — runtime TensorFlow Lite
--allow_custom_ops — TensorFlow Lite Optimizing Converter
--inference_type=FLOAT —
--inference_input_type=QUANTIZED_UINT8 —
--mean_values=128 --std_dev_values=128 — , QUANTIZED_UINT8
Google Drive:
!zip -r ./training_demo/training.zip ./training_demo/training/
training_result = drive.CreateFile({'title': 'training_result.zip'})
training_result.SetContentFile('training_demo/training.zip')
training_result.Upload()
Invalid client secrets file, google drive.
android
android object detection, kotlin CameraX. .
CameraX ImageAnalysis. ObjectDetectorAnalyzer.
:
1. YUV . RGB :
val rgbArray = convertYuvToRgb(image)
2. (, , , 300x300), Bitmap :
val rgbBitmap = getRgbBitmap(rgbArray, image.width, image.height)
val transformation = getTransformation(rotationDegrees, image.width, image.height)
Canvas(resizedBitmap).drawBitmap(rgbBitmap, transformation, null)
3. bitmap , :
ImageUtil.storePixels(resizedBitmap, inputArray)
val objects = detect(inputArray)
4. RecognitionResultOverlayView :
val scaleFactorX = measuredWidth / result.imageWidth.toFloat()
val scaleFactorY = measuredHeight / result.imageHeight.toFloat()
result.objects.forEach { obj ->
val left = obj.location.left * scaleFactorX
val top = obj.location.top * scaleFactorY
val right = obj.location.right * scaleFactorX
val bottom = obj.location.bottom * scaleFactorY
canvas.drawRect(left, top, right, bottom, boxPaint)
canvas.drawText(obj.text, left, top - 25f, textPaint)
}
, assets training_demo/training/model_q.tflite ( detect.tflite) labelmap.txt, :
SSD Mobilenet V1, 1, 0, labelOffset 1 0 collectDetectionResult ObjectDetector.
.
, Xiaomi Redmi 4X :
: