Klasifikasi dokumen atau teks adalah salah satu tugas paling penting dalam pemrosesan bahasa alami (NLP).
Ini memiliki banyak kegunaan, seperti mengelompokkan berita, memfilter spam, mencari komentar yang tidak pantas, dll.
Perusahaan besar tidak memiliki masalah mengumpulkan kumpulan data besar, sehingga mempelajari model klasifikasi teks dari awal adalah tugas yang layak.
Namun, untuk sebagian besar tugas di dunia nyata, kumpulan data besar jarang terjadi, dan Anda harus cerdas untuk membangun model.
Dalam artikel ini saya akan berbicara tentang pendekatan praktis untuk transformasi teks yang akan memungkinkan untuk mengklasifikasikan dokumen, bahkan jika kumpulan data kecil.
Pengantar Klasifikasi Dokumen
Proses klasifikasi dokumen dimulai dengan pembersihan dan persiapan tubuh.
Kemudian badan ini dikodekan dengan semua jenis representasi teks, setelah itu Anda dapat memulai pemodelan.
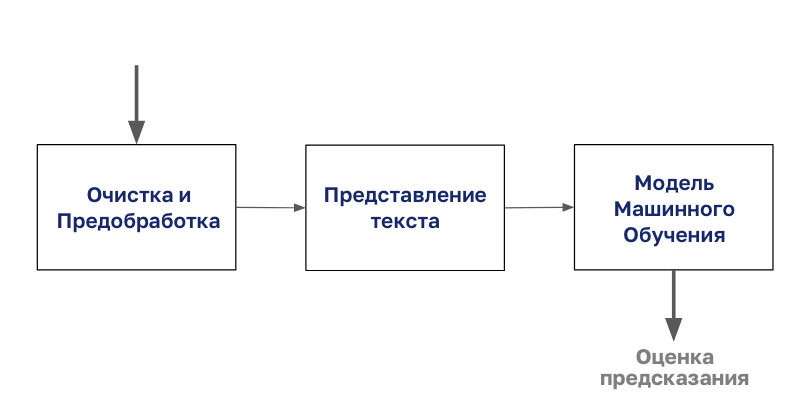
Dalam artikel ini, kita akan fokus pada langkah "Teks Penyajian" dari diagram ini.
Dataset uji untuk klasifikasi
Kami akan menggunakan data dari kompetisi. Benar atau tidak? NLP dengan tweet bencana Kaggle .
Tantangannya adalah untuk memprediksi tweet mana yang tentang bencana nyata dan mana yang bukan.
Jika Anda ingin mengulang artikel langkah demi langkah, jangan lupa untuk menginstal perpustakaan yang digunakan di dalamnya.
:
import pandas as pd
tweet= pd.read_csv('../input/nlp-getting-started/train.csv')
test=pd.read_csv('../input/nlp-getting-started/test.csv')
tweet.head(3)

, , , .
.
print('There are {} rows and {} columns in train'.format(tweet.shape[0],tweet.shape[1]))
print('There are {} rows and {} columns in test'.format(test.shape[0],test.shape[1]))
8000 .
, 280 .
, NLP, .
, , , .
, :
- — .
- - — «a» «the».
- — («studies», «studing» → «study»).
def preprocess_news(df):
'''Function to preprocess and create corpus'''
new_corpus=[]
lem=WordNetLemmatizer()
for text in df["question_text"]:
words=[w for w in word_tokenize(text) if (w not in stop)]
words=[lem.lemmatize(w) for w in words]
new_corpus.append(words)
return new_corpus
corpus=preprocess_news(df)
, , .
, .
.
CountVectorizer
CountVectorizer — .
, , .
:
text = ["She sells seashells in the seashore"]
vectorizer = CountVectorizer()
vectorizer.fit(text)
print(vectorizer.vocabulary_)
vector = vectorizer.transform(text)
print(vector.shape)
print(type(vector))
print(vector.toarray())

, CountVectorizer Numpy, .
, , .
vector=vectorizer.transform(["I sell seashells in the seashore"])
vector.toarray()

, :
, — «sells» «she».
CountVectorizer, .
vec=CountVectorizer(max_df=10,max_features=10000)
vec.fit(df.question_text.values)
vector=vec.transform(df.question_text.values)
, CountVectorizer , :
- max_features — n , .
- min_df — , .
- max_df — , .
( ).
TfidfVectorizer
Countvectorizer , , "the" ( ) .
— TfidfVectorizer.
— Term frequency-inverse document frequency ( — ).
:
from sklearn.feature_extraction.text import TfidfVectorizer
text = ["She sells seashells by the seashore","The sea.","The seashore"]
vectorizer = TfidfVectorizer()
vectorizer.fit(text)
print(vectorizer.vocabulary_)
print(vectorizer.idf_)
vector = vectorizer.transform([text[0]])
print(vector.shape)
print(vector.toarray())
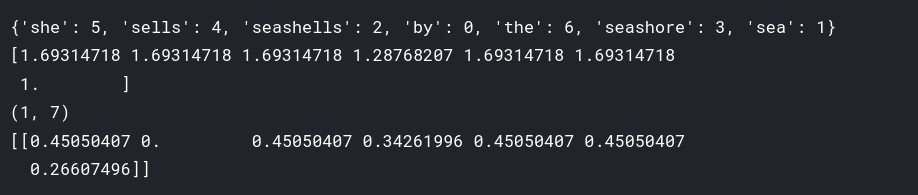
6 , «the», 4 .
0 1, - .
Word2vec
, .
(embeddings) .
n- .
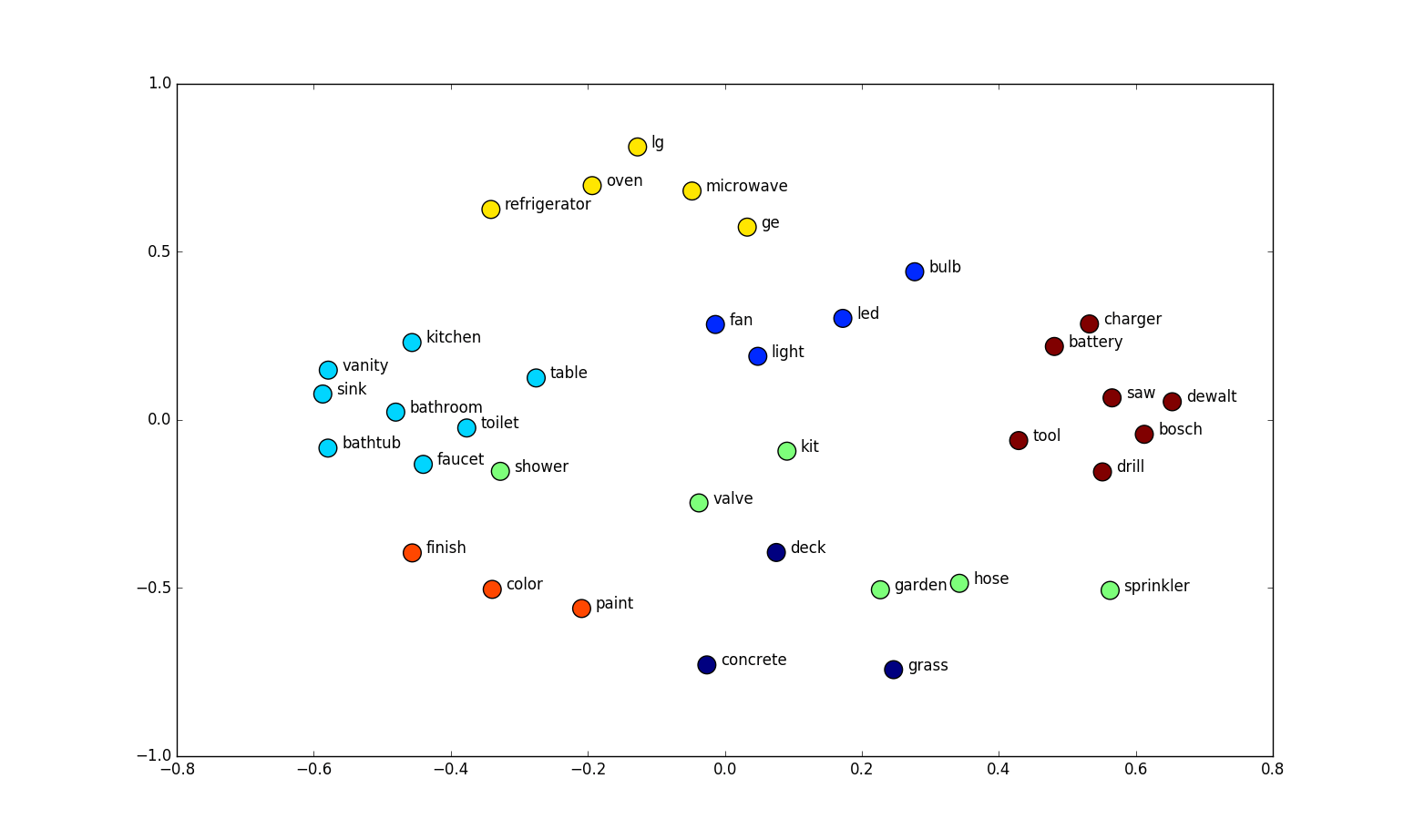
Word2Vec Google .
, .
, , .
«The cat sat on the mat».
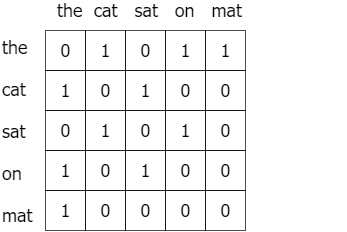
Word2vec :
, , , . word2vec python:
import gensim
from gensim.models import Word2Vec
model = gensim.models.Word2Vec(corpus,
min_count = 1, size = 100, window = 5)
, word2vec.
:
- size — .
- min_count — .
- window — , . .
.
.
.
, gensim.
from gensim.models.KeyedVectors import load_word2vec_format
def load_word2vec():
word2vecDict = load_word2vec_format(
'../input/word2vec-google/GoogleNews-vectors-negative300.bin',
binary=True, unicode_errors='ignore')
embeddings_index = dict()
for word in word2vecDict.wv.vocab:
embeddings_index[word] = word2vecDict.word_vec(word)
return embeddings_index
:
w2v_model=load_word2vec()
w2v_model['London'].shape

, 300- .
( — , . , , . . )
, .
FastText
Genism — FastText.
Facebook .
Continuous Bag of Words Skip-Gram.
FastText , n-.
, , «orange».
«ora», «ran», «ang», «nge» ( ).
( ) «orange» n-.
, n- .
, «stupedofantabulouslyfantastic», , , , genism , .
FastText , , .
«fantastic» «fantabulous».
, .
.
n- .
.
from gensim.models import FastText
def load_fasttext():
print('loading word embeddings...')
embeddings_index = {}
f = open('../input/fasttext/wiki.simple.vec',encoding='utf-8')
for line in tqdm(f):
values = line.strip().rsplit(' ')
word = values[0]
coefs = np.asarray(values[1:], dtype='float32')
embeddings_index[word] = coefs
f.close()
print('found %s word vectors' % len(embeddings_index))
return embeddings_index
embeddings_index=load_fastext()
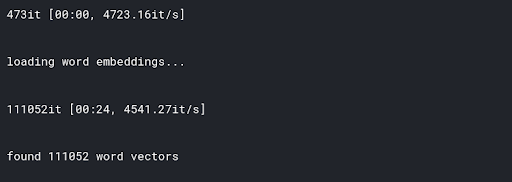
:
embeddings_index['london'].shape

GloVe
GloVe (global vectors for word representation) « ».
, .
, .
word2vec, .
.
.
.
.
, .
n- .
:

.
.
:
def load_glove():
embedding_dict = {}
path = '../input/glove-global-vectors-for-word-representation/glove.6B.100d.txt'
with open(path, 'r') as f:
for line in f:
values = line.split()
word = values[0]
vectors = np.asarray(values[1:], 'float32')
embedding_dict[word] = vectors
f.close()
return embedding_dict
embeddings_index = load_glove()
, , GloVe.
- .
embeddings_index['london'].shape
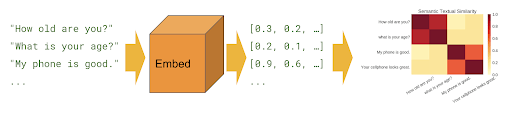
.
.
.
, .
:
, .
.
.
Tensorflow.
module_url = "../input/universalsentenceencoderlarge4"
embed = hub.load(module_url)
.
sentence_list=df.question_text.values.tolist()
sentence_emb=embed(sentence_list)['outputs'].numpy()
.
Elmo, BERT
, .
.
«stick», «», «» , , .
NLP BERT , . .
, Keras .
, .
, Keras Tokenizer pad_sequences.
MAX_LEN=50
tokenizer_obj=Tokenizer()
tokenizer_obj.fit_on_texts(corpus)
sequences=tokenizer_obj.texts_to_sequences(corpus)
tweet_pad=pad_sequences(sequences,
maxlen=MAX_LEN,
truncating='post',
padding='post')
.
word_index=tokenizer_obj.word_index
print('Number of unique words:',len(word_index))
, .
.
def prepare_matrix(embedding_dict, emb_size=300):
num_words = len(word_index)
embedding_matrix = np.zeros((num_words, emb_size))
for word, i in tqdm(word_index.items()):
if i > num_words:
continue
emb_vec = embedding_dict.get(word)
if emb_vec is not None:
embedding_matrix[i] = emb_vec
return embedding_matrix
.
trainable=False, .
def new_model(embedding_matrix):
inp = Input(shape=(MAX_LEN,))
x = Embedding(num_words, embedding_matrix.shape[1], weights=[embedding_matrix],
trainable=False)(inp)
x = Bidirectional(
LSTM(60, return_sequences=True, name='lstm_layer',
dropout=0.1, recurrent_dropout=0.1))(x)
x = GlobalAveragePool1D()(x)
x = Dense(1, activation="sigmoid")(x)
model = Model(inputs=inp, outputs=x)
model.compile(loss='binary_crossentropy',
optimizer='adam',
metrics=['accuracy'])
return model
, , word2vec:
embeddings_index=load_word2vec()
embedding_matrix=prepare_matrix(embeddings_index)
model=new_model(embedding_matrix)
history=model.fit(X_train,y_train,
batch_size=8,
epochs=5,
validation_data=(X_test,y_test),
verbose=2)
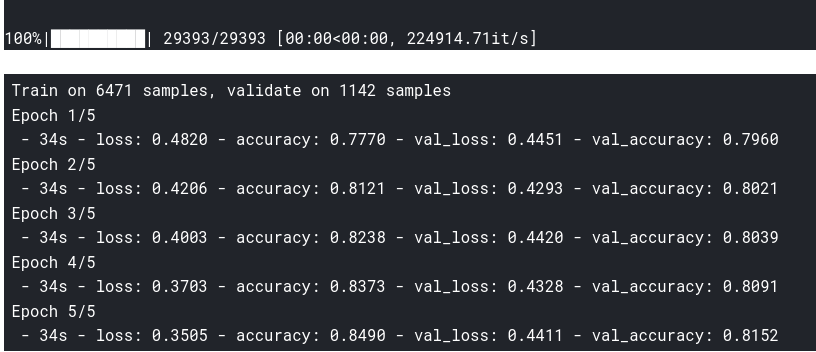
, .
?
Neptune .
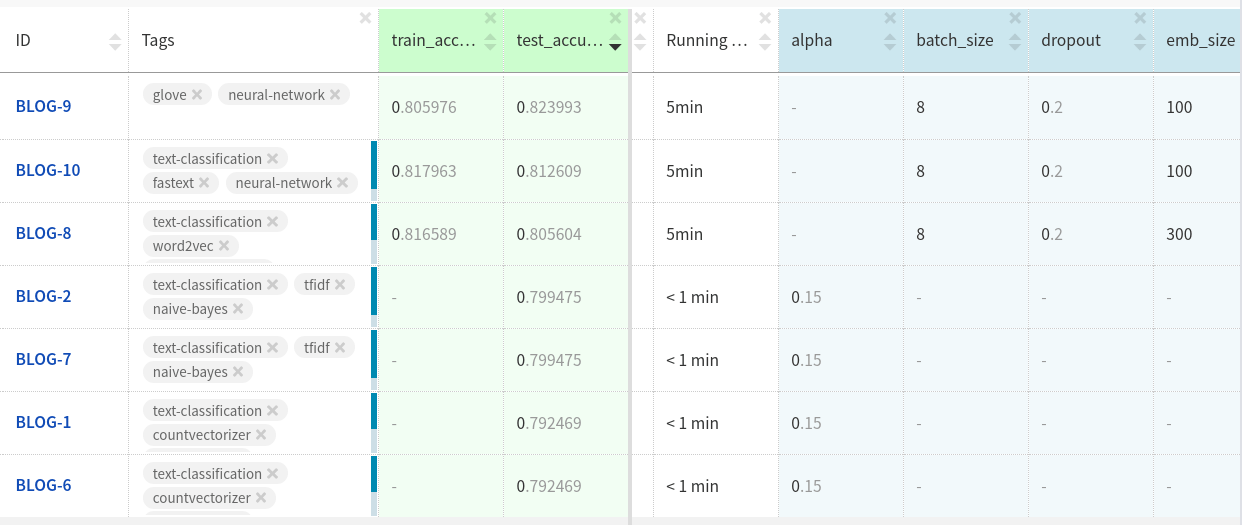
Lampiran GloVe dalam dataset uji memiliki kinerja yang sedikit lebih baik daripada dua lampiran lainnya.
Mungkin Anda akan mencapai lebih banyak jika Anda lebih lanjut menyesuaikan model dan membersihkan data.
Anda dapat melihat eksperimen di sini .
Kesimpulan
Dalam artikel ini, kami memeriksa dan menerapkan berbagai metode untuk menyajikan atribut untuk klasifikasi teks yang digunakan saat bekerja dengan set data kecil.
Saya harap mereka berguna dalam proyek Anda.