Halo, Habr! Kami tiba-tiba menyadari bahwa artikel terakhir kami tentang Elbrus keluar setahun yang lalu. Karena itu, kami memutuskan untuk memperbaiki pengawasan yang menjengkelkan ini, karena kami tidak meninggalkan topik ini!
Sulit membayangkan pengenalan tanpa jaringan saraf, jadi kita akan berbicara tentang bagaimana kami meluncurkan grid 8-bit pada Elbrus dan apa yang terjadi. Secara umum, model dengan koefisien dan input 8-bit dan perhitungan menengah 32-bit sangat populer. Sebagai contoh, Google [1] dan Facebook [2] meluncurkan implementasinya sendiri yang mengoptimalkan akses ke memori, menggunakan SIMD dan memungkinkan Anda untuk mempercepat perhitungan hingga 25% atau lebih tanpa penurunan akurasi yang nyata (ini tentu saja tergantung pada arsitektur jaringan saraf dan kalkulator, tetapi Anda perlu dijelaskan betapa kerennya itu?).

Gagasan mengganti bilangan real dengan bilangan bulat untuk mempercepat perhitungan ada di udara:
- . , ββ , float , ;
- . - , , β¦ , . , (SIMD). c 128- SIMD 4 floatβ 16 uint8. 4 , ;
- , . β !
8- [3] . , , .
8- , 16-, . 32- . , 8- . , .
, β . (. . 1) :
β , β , β , β .
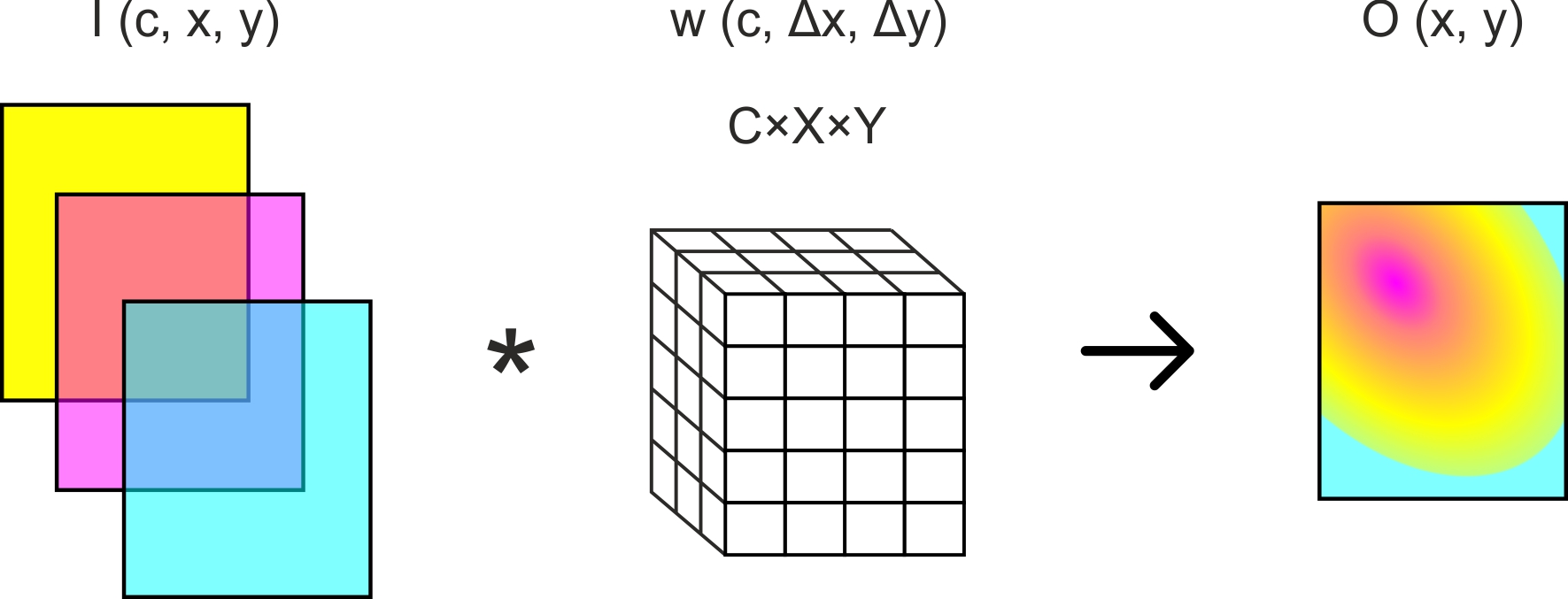
. 1. C x X x Y.
β [4]. , , . , "" β .. , . , .. (1). "" , .. ( ), ..
8- 32- .
- , , .
β , . , , β . . , , , [5-7].
6 ( ), . : - , . , , , , , . , .
. 1 (). 32 .
1. . "/" , , .
, - APB (array prefetch buffer). APB n- , . -, , APB , . APB :
, APB :
, , . , . Goto [8] . , , , . .
, , (. . 2-3). β . , .. . , APB - : , 8 ( 64- ) 2 .
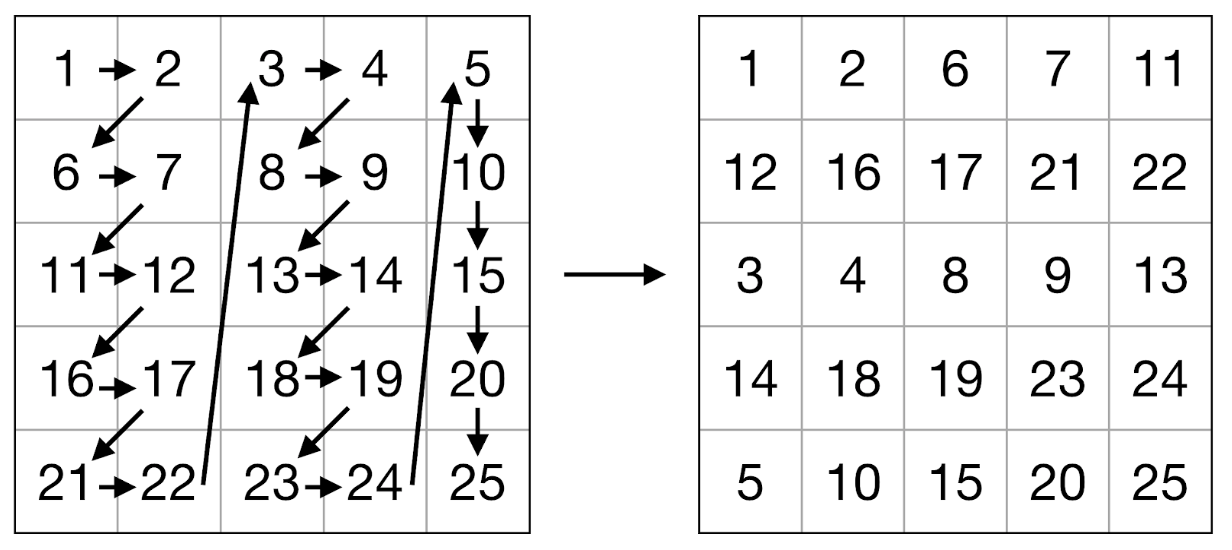
. 2. .

3. .
:
for bl_r in block_r(rhs):
packed_r <- pack_rhs(bl_r)
for bl_l in block_l(lhs):
packed_l <- pack_lhs(bl_l)
packed_res <- pack_res(bl_res)
kernel(packed_res, packed_l, packed_r)
bl_res <- unpack_res(packed_res)
lhs
rhs
β , block_l(.)
, block_r(.)
β , lhs
rhs
. pack_rhs
pack_lhs
, pack_res
β , unpack_res
β . kernel
.
kernel
:
for j in {0, ..., cols / nr}
{dst0, dst1} <-
for i in {0, ..., rows / mr}
for k in {0, ..., depth / 2}
bl_r <-
bl_l <-
lhs <- pshufb(zero, bl_l, 0x0901080009010800LL)
rhs0 <- punpcklbh(zero, bl_r)
rhs1 <- punpckhbh(zero, bl_r)
dst0 <- dst0 + pmaddh(rhs0, lhs)
dst1 <- dst1 + pmaddh(rhs1, lhs);
//
pshufb
β , ( ), punpckhbh
β , 16- , punpcklbh
β , 16- , pmaddh
β , 16- .
. nr mr = 12 8.
, 8 48 . 8 3, 14 , β 48 +, 8 .
2 8- -4, EML. N = 10^6
.
2. EML .
, -4 . , .
8- , x86 ARM, . , , , . 8- . , , ( , x86 ARM), : 6 64- , (64 128 ) 2 6 .
, 8- 32- . 3 (-4), 4 5 (-8 -8).
? , . , ββ , . - , . , , / .
- 8- ? , , , - ( ).
P.S.
Limonova E. E., Neyman-Zade M. I., Arlazarov V. L. Special aspects of matrix operation implementations for low-precision neural network model on the Elbrus platform // . . β 2020. β . 13. β β 1. β . 118-128. β DOI: 10.14529/mmp200109.
- https://github.com/google/gemmlowp
- https://engineering.fb.com/ml-applications/qnnpack/
- Vanhoucke, Vincent, Andrew Senior, and Mark Z. Mao. "Improving the speed of neural networks on CPUs." (2011).
- K. Chellapilla, S. Puri, P. Simard. High Performance Convolutional Neural Networks for Document Processing // Tenth International Workshop on Frontiers in Handwriting Recognition. β Universite de Rennes, 1 Oct 2006. β La Baule (France). β 2006.
- .., . ., . . ββ. β .: , β 2013. β 272 c.
- , .. / .. , .. , .. // - . β .: - ββ . . .. . β 2015. β No4 (8). β cc. 64-68.
- Limonova E. E., Skoryukina N. S., Neyman-Zade M. I. Fast Hamming Distance Computation for 2D Art Recognition on VLIW-Architecture in Case of Elbrus Platform // ICMV 2018 / SPIE. 2019. . 11041. ISSN 0277-786X. ISBN 978-15-10627-48-2. 2019. . 11041. 110411N. DOI: 10.1117/12.2523101
- Goto, K. Anatomy of high-performance matrix multiplication / K. Goto, R.A. Geijn // ACM Transactions on Mathematical Software (TOMS) β 2008. β 34(3). β p.12.