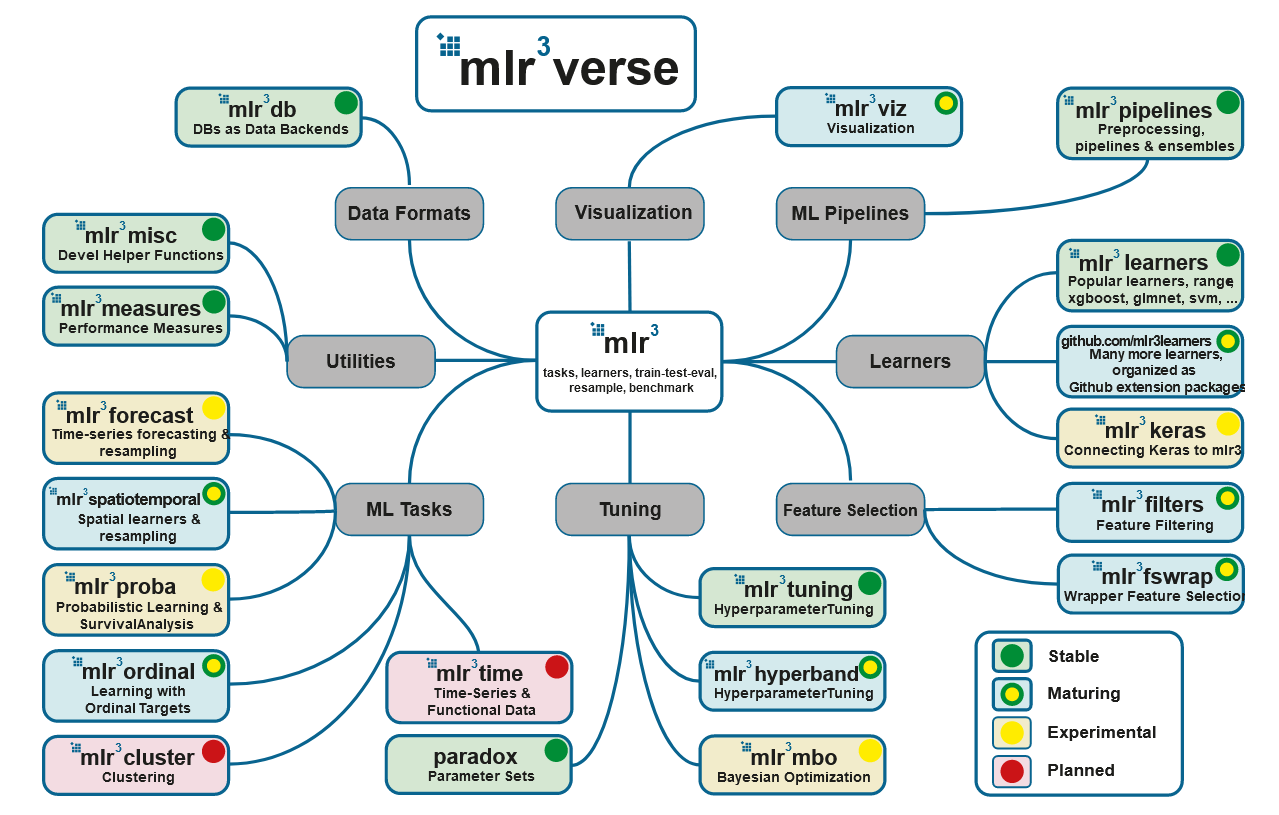
Sumber: https://mlr3book.mlr-org.com/
Halo, Habr!Dalam posting ini, kami akan mempertimbangkan pendekatan yang paling bijaksana untuk pembelajaran mesin dalam bahasa R hari ini - paket mlr3 dan ekosistem di sekitarnya. Pendekatan ini didasarkan pada OOP "normal" menggunakan kelas R6 dan mewakili semua operasi dengan data dan model dalam bentuk grafik perhitungan. Ini memungkinkan Anda membuat saluran pipa yang ramping dan fleksibel untuk tugas-tugas pembelajaran mesin, tetapi pada awalnya mungkin terlihat rumit dan membingungkan. Di bawah ini kami akan mencoba memberikan kejelasan dan memotivasi untuk menggunakan mlr3 dalam proyek Anda.Kandungan:
- Sedikit sejarah dan perbandingan dengan solusi yang bersaing
- Rincian teknis: paket kelas R6 dan data.table
- Komponen utama pipline ML dalam mlr3
- Pengaturan Hyperparameters
- Ikhtisar ekosistem Mlr3
- Pipa dan grafik perhitungan
1.
caret โ ,
caret R ( CRAN 2007 ). 2013 Applied Predictive Modeling, .
:
- ( - );
- (-), , , ;
- , caret- - ;
- โ , xgboost
nrounds
, max_depth
, eta
, gamma
, colsample_bytree
, min_child_weight
subsample
.
:
- โ , . ;
- : , . recipes , ;
- - (nested resampling), caretEnsemble.
tidyverse strikes back
tidymodels, recipes ( ยซยป , ), rsample ( ) tune ( ).
:
- ยซยป , ;
- , embed textrecipes;
- , . ( tune);
- workflows ยซยป .
:
- , tune . ยซยป , ,
apply
/map
-; - . , 200 ;
- - .
mlr3 vs
mlr3 mlr , caret tidymodels. mlr , mlr3.
:
- R6-, data.table;
- . , ;
- API ,
learner
โ ; - .
:
2. : R6- data.table
mlr3 ยซยป , R6-. R6- , . Advanced R, , .
R6- R6Class()
:
library(R6)
Accumulator <- R6Class("Accumulator", list(
sum = 0,
add = function(x = 1) {
self$sum <- self$sum + x
invisible(self)
})
)
โ "Accumulator"
.
new()
, (, , ) :
x <- Accumulator$new()
, , :
x$add(4)
x$sum
#> [1] 4
R6- :
y1 <- Accumulator$new()
y2 <- y1
y1$add(10)
c(y1 = y1$sum, y2 = y2$sum)
#> y1 y2
#> 10 10
clone()
( clone(deep = TRUE)
):
y1 <- Accumulator$new()
y2 <- y1$clone()
y1$add(10)
c(y1 = y1$sum, y2 = y2$sum)
#> y1 y2
#> 10 0
, R6 mlr3.
data.table ( , data.table data.table: R). - data.table
, :=
. , , 2 , . , , .
3. ML- mlr3

: https://mlr3book.mlr-org.com/
mlr3 :
library(mlr3)
#
task <- TaskClassif$new(id = "iris",
backend = iris,
target = "Species")
task
# <TaskClassif:iris> (150 x 5)
# * Target: Species
# * Properties: multiclass
# * Features (4):
# - dbl (4): Petal.Length, Petal.Width, Sepal.Length, Sepal.Width
#
# learner_rpart <- mlr_learners$get("classif.rpart")
learner_rpart <- lrn("classif.rpart",
predict_type = "prob",
minsplit = 50)
learner_rpart
# <LearnerClassifRpart:classif.rpart>
# * Model: -
# * Parameters: xval=0, minsplit=50
# * Packages: rpart
# * Predict Type: prob
# * Feature types: logical, integer, numeric, factor, ordered
# * Properties: importance, missings, multiclass, selected_features, twoclass, weights
#
learner_rpart$param_set
# ParamSet:
# id class lower upper levels default value
# 1: minsplit ParamInt 1 Inf 20 50
# 2: minbucket ParamInt 1 Inf <NoDefault>
# 3: cp ParamDbl 0 1 0.01
# 4: maxcompete ParamInt 0 Inf 4
# 5: maxsurrogate ParamInt 0 Inf 5
# 6: maxdepth ParamInt 1 30 30
# 7: usesurrogate ParamInt 0 2 2
# 8: surrogatestyle ParamInt 0 1 0
# 9: xval ParamInt 0 Inf 10 0
#
learner_rpart$train(task, row_ids = 1:120)
learner_rpart$model
# n= 120
#
# node), split, n, loss, yval, (yprob)
# * denotes terminal node
#
# 1) root 120 70 setosa (0.41666667 0.41666667 0.16666667)
# 2) Petal.Length< 2.45 50 0 setosa (1.00000000 0.00000000 0.00000000) *
# 3) Petal.Length>=2.45 70 20 versicolor (0.00000000 0.71428571 0.28571429)
# 6) Petal.Length< 4.95 49 1 versicolor (0.00000000 0.97959184 0.02040816) *
# 7) Petal.Length>=4.95 21 2 virginica (0.00000000 0.09523810 0.90476190) *
: (task) (learner).
(TaskClassif
, TaskRegr
..) new()
. id
, backend
target
; positive
. : mlr_tasks$get("iris")
tsk("iris")
.
mlr_learners
get()
train()
, task
, . : lrn("classif.rpart", predict_type = "prob", minsplit = 50)
. (predict_type = "prob"
) (minsplit = 50
). : learner_rpart$predict_type <- "prob"
, learner_rpart$param_set$values$minsplit = 50
.
predict_newdata()
:
#
preds <- learner_rpart$predict_newdata(newdata = iris[121:150, ])
preds
# <PredictionClassif> for 30 observations:
# row_id truth response prob.setosa prob.versicolor prob.virginica
# 1 virginica virginica 0 0.0952381 0.90476190
# 2 virginica versicolor 0 0.9795918 0.02040816
# 3 virginica virginica 0 0.0952381 0.90476190
# ---
# 28 virginica virginica 0 0.0952381 0.90476190
# 29 virginica virginica 0 0.0952381 0.90476190
# 30 virginica virginica 0 0.0952381 0.90476190
- 5 :
cv10 <- rsmp("cv", folds = 5)
resample_results <- resample(task, learner_rpart, cv10)
# INFO [09:37:05.993] Applying learner 'classif.rpart' on task 'iris' (iter 1/5)
# INFO [09:37:06.018] Applying learner 'classif.rpart' on task 'iris' (iter 2/5)
# INFO [09:37:06.042] Applying learner 'classif.rpart' on task 'iris' (iter 3/5)
# INFO [09:37:06.074] Applying learner 'classif.rpart' on task 'iris' (iter 4/5)
# INFO [09:37:06.098] Applying learner 'classif.rpart' on task 'iris' (iter 5/5)
resample_results
# <ResampleResult> of 5 iterations
# * Task: iris
# * Learner: classif.rpart
# * Warnings: 0 in 0 iterations
# * Errors: 0 in 0 iterations
# (-):
as.data.table(mlr_resamplings)
# key params iters
# 1: bootstrap repeats,ratio 30
# 2: custom 0
# 3: cv folds 10
# 4: holdout ratio 1
# 5: repeated_cv repeats,folds 100
# 6: subsampling repeats,ratio 30
. score()
resample_results
, โ accuracy "classif.acc"
classification error "classif.ce"
. , get()
: mlr_measures$get("classif.ce")
. msrs()
:
resample_results$score(msrs(c("classif.acc", "classif.ce")))[, 5:10]
#
# resampling resampling_id iteration prediction classif.acc classif.ce
# 1: <ResamplingCV> cv 1 <list> 0.8666667 0.13333333
# 2: <ResamplingCV> cv 2 <list> 0.9666667 0.03333333
# 3: <ResamplingCV> cv 3 <list> 0.9333333 0.06666667
# 4: <ResamplingCV> cv 4 <list> 0.9666667 0.03333333
# 5: <ResamplingCV> cv 5 <list> 0.9333333 0.06666667
4.
โ . , .
. paradox:
library("paradox")
searchspace <- ParamSet$new(list(
ParamDbl$new("cp", lower = 0.001, upper = 0.1),
ParamInt$new("minsplit", lower = 1, upper = 10)
))
searchspace
# ParamSet:
# id class lower upper levels default value
# 1: cp ParamDbl 0.001 0.1 <NoDefault>
# 2: minsplit ParamInt 1.000 10.0 <NoDefault>
ParamSet
, cp
minsplit
; rpart
.
, searchspace
. tune()
Tuner
. . resolution
, , param_resolutions
, . , , .
generate_design_grid()
, :
generate_design_grid(searchspace,
param_resolutions = c("cp" = 2, "minsplit" = 3))
# <Design> with 6 rows:
# cp minsplit
# 1: 0.001 1
# 2: 0.001 5
# 3: 0.001 10
# 4: 0.100 1
# 5: 0.100 5
# 6: 0.100 10
: generate_design_random()
generate_design_lhs()
.
, . Terminator
-, , ( ), . mlr3tuning:
library("mlr3tuning")
evals20 <- term("evals", n_evals = 20)
evals20
# <TerminatorEvals>
# * Parameters: n_evals=20
#
as.data.table(mlr_terminators)
# key
# 1: clock_time
# 2: combo
# 3: evals
# 4: model_time
# 5: none
# 6: perf_reached
# 7: stagnation
TuningInstance
:
tuning_instance <- TuningInstance$new(
task = TaskClassif$new(id = "iris",
backend = iris,
target = "Species"),
learner = lrn("classif.rpart",
predict_type = "prob"),
resampling = rsmp("cv", folds = 5),
measures = msr("classif.ce"),
param_set = ParamSet$new(
list(ParamDbl$new("cp", lower = 0.001, upper = 0.1),
ParamInt$new("minsplit", lower = 1, upper = 10)
)
),
terminator = term("evals", n_evals = 20)
)
tuning_instance
# <TuningInstance>
# * State: Not tuned
# * Task: <TaskClassif:iris>
# * Learner: <LearnerClassifRpart:classif.rpart>
# * Measures: classif.ce
# * Resampling: <ResamplingCV>
# * Terminator: <TerminatorEvals>
# * bm_args: list()
# * n_evals: 0
# ParamSet:
# id class lower upper levels default value
# 1: cp ParamDbl 0.001 0.1 <NoDefault>
# 2: minsplit ParamInt 1.000 10.0 <NoDefault>
โ Tuner
, :
tuner <- tnr("grid_search",
resolution = 5,
batch_size = 2)
#
# as.data.table(mlr_tuners)
# key
# 1: design_points
# 2: gensa
# 3: grid_search
# 4: random_search
resolution = 5
, 25 . 20 , terminator = term("evals", n_evals = 20)
. batch_size
โ , . mlr3 โ , .
tnr("design_points")
: , ( โ , mlr3 ).
, :
result <- tuner$tune(tuning_instance)
result
# NULL
, result
. , tuner$tune()
tuning_instance
:
tuning_instance$result
# $tune_x
# $tune_x$cp
# [1] 0.001
#
# $tune_x$minsplit
# [1] 5
#
#
# $params
# $params$xval
# [1] 0
#
# $params$cp
# [1] 0.001
#
# $params$minsplit
# [1] 5
#
#
# $perf
# classif.ce
# 0.04
result <- tuning_instance$archive(unnest = "params")
result[order(classif.ce), c("cp", "minsplit", "classif.ce")]
# cp minsplit classif.ce
# 1: 0.00100 5 0.04000000
# 2: 0.00100 3 0.04000000
# 3: 0.00100 8 0.04000000
# 4: 0.00100 1 0.04000000
# 5: 0.00100 10 0.04666667
# 6: 0.02575 10 0.06000000
# 7: 0.07525 5 0.06000000
# 8: 0.02575 8 0.06000000
# 9: 0.02575 3 0.06000000
# 10: 0.05050 1 0.06000000
# 11: 0.07525 3 0.06000000
# 12: 0.07525 1 0.06000000
# 13: 0.05050 3 0.06000000
# 14: 0.02575 5 0.06000000
# 15: 0.05050 5 0.06000000
# 16: 0.05050 8 0.06000000
# 17: 0.10000 3 0.06000000
# 18: 0.10000 8 0.06000000
# 19: 0.05050 10 0.06000000
# 20: 0.10000 1 0.06000000
library(ggplot2)
ggplot(result,
aes(x = cp, y = classif.ce, color = as.factor(minsplit))) +
geom_line() +
geom_point(size = 3)

, tune()
:
Tuner
( batch_size
);Learner
Task
. ResampleResult
( BenchmarkResult
);Terminator
, . , 1, , ;- ;
- , ( , ,
msr("classif.ce", aggregator = "median")
.
tuning_instance$bmr
, BenchmarkResult
, score()
as.data.table(tuning_instance$bmr)
. , , ResampleResult
tuning_instance$archive()
:
tuning_instance$archive()[1, resample_result][[1]]$score()[, 4:9]
# learner_id resampling resampling_id iteration prediction classif.ce
# 1: classif.rpart <ResamplingCV> cv 1 <list> 0.06666667
# 2: classif.rpart <ResamplingCV> cv 2 <list> 0.16666667
# 3: classif.rpart <ResamplingCV> cv 3 <list> 0.03333333
# 4: classif.rpart <ResamplingCV> cv 4 <list> 0.03333333
# 5: classif.rpart <ResamplingCV> cv 5 <list> 0.00000000
, :
res <- tuning_instance$archive(unnest = "params")
res[, ce_resemples := lapply(resample_result, function(x) x$score()[, classif.ce])]
ce_resemples <- res[, .(ce_resemples = unlist(ce_resemples)), by = nr]
res[ce_resemples, on = "nr"]
5. mlr3
: mlr3, mlr3tuning paradox. , - mlr3verse:
# install.packages("mlr3verse")
library(mlr3verse)
## Loading required package: mlr3
## Loading required package: mlr3db
## Loading required package: mlr3filters
## Loading required package: mlr3learners
## Loading required package: mlr3pipelines
## Loading required package: mlr3tuning
## Loading required package: mlr3viz
## Loading required package: paradox
- mlr3db dbplyr data.table.
- mlr3filters , ( ).
- mlr3learners (
regr.glmnet
, regr.kknn
, regr.km
, regr.lm
, regr.ranger
, regr.svm
, regr.xgboost
) (classif.glmnet
, classif.kknn
, classif.lda
, classif.log_reg
, classif.multinom
, classif.naive_bayes
, classif.qda
, classif.ranger
, classif.svm
, classif.xgboost
). . - mlr3pipelines (pipelines), . , , CRAN, :
remotes::install_github("https://github.com/mlr-org/mlr3pipelines")
. - mlr3tuning .
- mlr3viz , .
- mlr3measures โ ~40 . mlr3verse , .
, .
6.
(pipelines) , , .
โ , , โ . PipeOpLearner()
, โ PipeOpFilter()
, โ PipeOp()
. ( po()
) :
pca <- po("pca")
filter <- po("filter",
filter = mlr3filters::flt("variance"),
filter.frac = 0.5)
learner_po <- po("learner",
learner = lrn("classif.rpart"))
%>>%
:
graph <- pca %>>% filter %>>% learner_po
graph$plot()
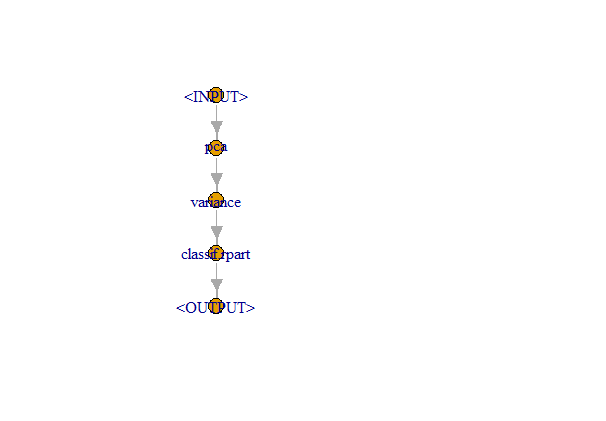
. , :
gr <- Graph$new()$
add_pipeop(mlr_pipeops$get("copy", outnum = 2))$
add_pipeop(mlr_pipeops$get("scale"))$
add_pipeop(mlr_pipeops$get("pca"))$
add_pipeop(mlr_pipeops$get("featureunion", innum = 2))
gr$
add_edge("copy", "scale", src_channel = 1)$
add_edge("copy", "pca", src_channel = "output2")$
add_edge("scale", "featureunion", dst_channel = 1)$
add_edge("pca", "featureunion", dst_channel = 2)
gr$plot(html = FALSE)

, (po("learner", learner = lrn("classif.rpart"))
). , :
glrn <- GraphLearner$new(graph)
glrn
# <GraphLearner:pca.variance.classif.rpart>
# * Model: -
# * Parameters: variance.filter.frac=0.5, variance.na.rm=TRUE, classif.rpart.xval=0
# * Packages: -
# * Predict Type: response
# * Feature types: logical, integer, numeric, character, factor, ordered, POSIXct
# * Properties: importance, missings, multiclass, oob_error, selected_features, twoclass,
# weights
GraphLearner
Learner
. , Learner
-, :
resample(tsk("iris"), glrn, rsmp("cv"))
# INFO [17:17:00.358] Applying learner 'pca.variance.classif.rpart' on task 'iris' (iter 1/10)
# INFO [17:17:00.615] Applying learner 'pca.variance.classif.rpart' on task 'iris' (iter 2/10)
# INFO [17:17:00.881] Applying learner 'pca.variance.classif.rpart' on task 'iris' (iter 3/10)
# INFO [17:17:01.087] Applying learner 'pca.variance.classif.rpart' on task 'iris' (iter 4/10)
# INFO [17:17:01.303] Applying learner 'pca.variance.classif.rpart' on task 'iris' (iter 5/10)
# INFO [17:17:01.518] Applying learner 'pca.variance.classif.rpart' on task 'iris' (iter 6/10)
# INFO [17:17:01.716] Applying learner 'pca.variance.classif.rpart' on task 'iris' (iter 7/10)
# INFO [17:17:01.927] Applying learner 'pca.variance.classif.rpart' on task 'iris' (iter 8/10)
# INFO [17:17:02.129] Applying learner 'pca.variance.classif.rpart' on task 'iris' (iter 9/10)
# INFO [17:17:02.337] Applying learner 'pca.variance.classif.rpart' on task 'iris' (iter 10/10)
# <ResampleResult> of 10 iterations
# * Task: iris
# * Learner: pca.variance.classif.rpart
# * Warnings: 0 in 0 iterations
# * Errors: 0 in 0 iterations
, issue How to deal with different preprocessing steps as hyperparameters:
gr <- pipeline_branch(list(pca = po("pca"), nothing = po("nop")))
gr$plot()

caret tidymodels !
, mlr3. mlr3 book .