Deteksi anomali adalah tugas pembelajaran mesin yang menarik. Tidak ada cara khusus untuk menyelesaikannya, karena setiap kumpulan data memiliki karakteristiknya sendiri. Tetapi pada saat yang sama, ada beberapa pendekatan yang membantu untuk berhasil. Saya ingin berbicara tentang salah satu pendekatan ini - autoencoder.
Dataset mana yang harus dipilih?
Masalah paling mendesak dalam kehidupan ilmuwan data mana pun. Untuk menyederhanakan cerita, saya akan menggunakan dataset sederhana dalam struktur, yang akan kami hasilkan di sini.
import os
import numpy as np
from sklearn.model_selection import train_test_split
import matplotlib.pyplot as plt
from matplotlib.colors import Normalize
def gen_normal_distribution(mu, sigma, size, range=(0, 1), max_val=1):
bins = np.linspace(*range, size)
result = 1 / (sigma * np.sqrt(2*np.pi)) * np.exp(-(bins - mu)**2 / (2*sigma**2))
cur_max_val = result.max()
k = max_val / cur_max_val
result *= k
return result
Pertimbangkan contoh fungsi. Buat distribusi normal dengan μ = 0,3 dan σ = 0,05:
dist = gen_normal_distribution(0.3, 0.05, 256, max_val=1)
print(dist.max())
>>> 1.0
plt.plot(np.linspace(0, 1, 256), dist)
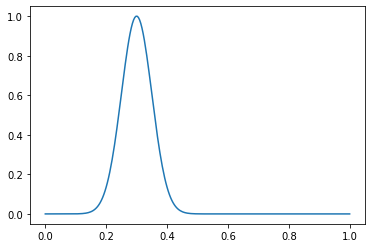
Nyatakan parameter dataset kami:
in_distribution_size = 2000
out_distribution_size = 200
val_size = 100
sample_size = 256
random_generator = np.random.RandomState(seed=42)
Dan fungsi untuk menghasilkan contoh normal dan tidak normal. Distribusi dengan satu maksimum akan dianggap normal, abnormal - dengan dua:
def generate_in_samples(size, sample_size):
global random_generator
in_samples = np.zeros((size, sample_size))
in_mus = random_generator.uniform(0.1, 0.9, size)
in_sigmas = random_generator.uniform(0.05, 0.5, size)
for i in range(size):
in_samples[i] = gen_normal_distribution(in_mus[i], in_sigmas[i], sample_size, max_val=1)
return in_samples
def generate_out_samples(size, sample_size):
global random_generator
out_samples = generate_in_samples(size, sample_size)
out_additional_mus = random_generator.uniform(0.1, 0.9, size)
out_additional_sigmas = random_generator.uniform(0.01, 0.05, size)
for i in range(size):
anomaly = gen_normal_distribution(out_additional_mus[i], out_additional_sigmas[i], sample_size, max_val=0.12)
out_samples[i] += anomaly
return out_samples
Ini adalah contoh yang normal:
in_samples = generate_in_samples(in_distribution_size, sample_size)
plt.plot(np.linspace(0, 1, sample_size), in_samples[42])

Dan abnormal - seperti ini:
out_samples = generate_out_samples(out_distribution_size, sample_size)
plt.plot(np.linspace(0, 1, sample_size), out_samples[42])
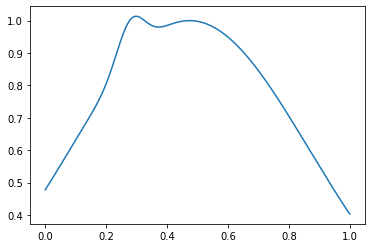
Buat array dengan tag dan tag:
x = np.concatenate((in_samples, out_samples))
y = np.concatenate((np.zeros(in_distribution_size), np.ones(out_distribution_size)))
x_train, x_test, y_train, y_test = train_test_split(x, y, test_size=0.2, shuffle=True, random_state=42)
. , , 2 1 (). , ( ), :
x_val_out = generate_out_samples(val_size, sample_size)
x_val_in = generate_in_samples(val_size, sample_size)
x_val = np.concatenate((x_val_out, x_val_in))
y_val = np.concatenate((np.ones(val_size), np.zeros(val_size)))
, , Sklearn: SVM . , , .
from sklearn.metrics import classification_report
from sklearn.metrics import f1_score
One class SVM
from sklearn.svm import OneClassSVM
OneClassSVM nu
— .
out_dist_part = out_distribution_size / (out_distribution_size + in_distribution_size)
svm = OneClassSVM(nu=out_dist_part)
svm.fit(x_train, y_train)
>>> OneClassSVM(cache_size=200, coef0=0.0, degree=3, gamma='scale', kernel='rbf',
max_iter=-1, nu=0.09090909090909091, shrinking=True, tol=0.001,
verbose=False)
:
svm_prediction = svm.predict(x_val)
svm_prediction[svm_prediction == 1] = 0
svm_prediction[svm_prediction == -1] = 1
sklearn — classification_report
, Anomaly detection , precision recall, :
print(classification_report(y_val, svm_prediction))
>>> precision recall f1-score support
0.0 0.57 0.93 0.70 100
1.0 0.81 0.29 0.43 100
accuracy 0.61 200
macro avg 0.69 0.61 0.57 200
weighted avg 0.69 0.61 0.57 200
, . f1-score , .
Isolation forest
, , - ?
from sklearn.ensemble import IsolationForest
, . :
out_dist_part = out_distribution_size / (out_distribution_size + in_distribution_size)
iso_forest = IsolationForest(n_estimators=100, contamination=out_dist_part, max_features=100, n_jobs=-1)
iso_forest.fit(x_train)
>>> IsolationForest(behaviour='deprecated', bootstrap=False,
contamination=0.09090909090909091, max_features=100,
max_samples='auto', n_estimators=100, n_jobs=-1,
random_state=None, verbose=0, warm_start=False)
Classification report? — Classification report!
iso_forest_prediction = iso_forest.predict(x_val)
iso_forest_prediction[iso_forest_prediction == 1] = 0
iso_forest_prediction[iso_forest_prediction == -1] = 1
print(classification_report(y_val, iso_forest_prediction))
>>> precision recall f1-score support
0.0 0.50 0.91 0.65 100
1.0 0.53 0.10 0.17 100
accuracy 0.51 200
macro avg 0.51 0.51 0.41 200
weighted avg 0.51 0.51 0.41 200
RandomForestClassifier
, - " ?" , :
from sklearn.ensemble import RandomForestClassifier
random_forest = RandomForestClassifier(n_estimators=100, max_features=100, n_jobs=-1)
random_forest.fit(x_train, y_train)
>>> RandomForestClassifier(bootstrap=True, ccp_alpha=0.0, class_weight=None,
criterion='gini', max_depth=None, max_features=100,
max_leaf_nodes=None, max_samples=None,
min_impurity_decrease=0.0, min_impurity_split=None,
min_samples_leaf=1, min_samples_split=2,
min_weight_fraction_leaf=0.0, n_estimators=100,
n_jobs=-1, oob_score=False, random_state=None, verbose=0,
warm_start=False)
random_forest_prediction = random_forest.predict(x_val)
print(classification_report(y_val, random_forest_prediction))
>>> precision recall f1-score support
0.0 0.57 0.99 0.72 100
1.0 0.96 0.25 0.40 100
accuracy 0.62 200
macro avg 0.77 0.62 0.56 200
weighted avg 0.77 0.62 0.56 200
Autoencoder
, : . .
, "" , . 2 : Encoder' Decoder', .
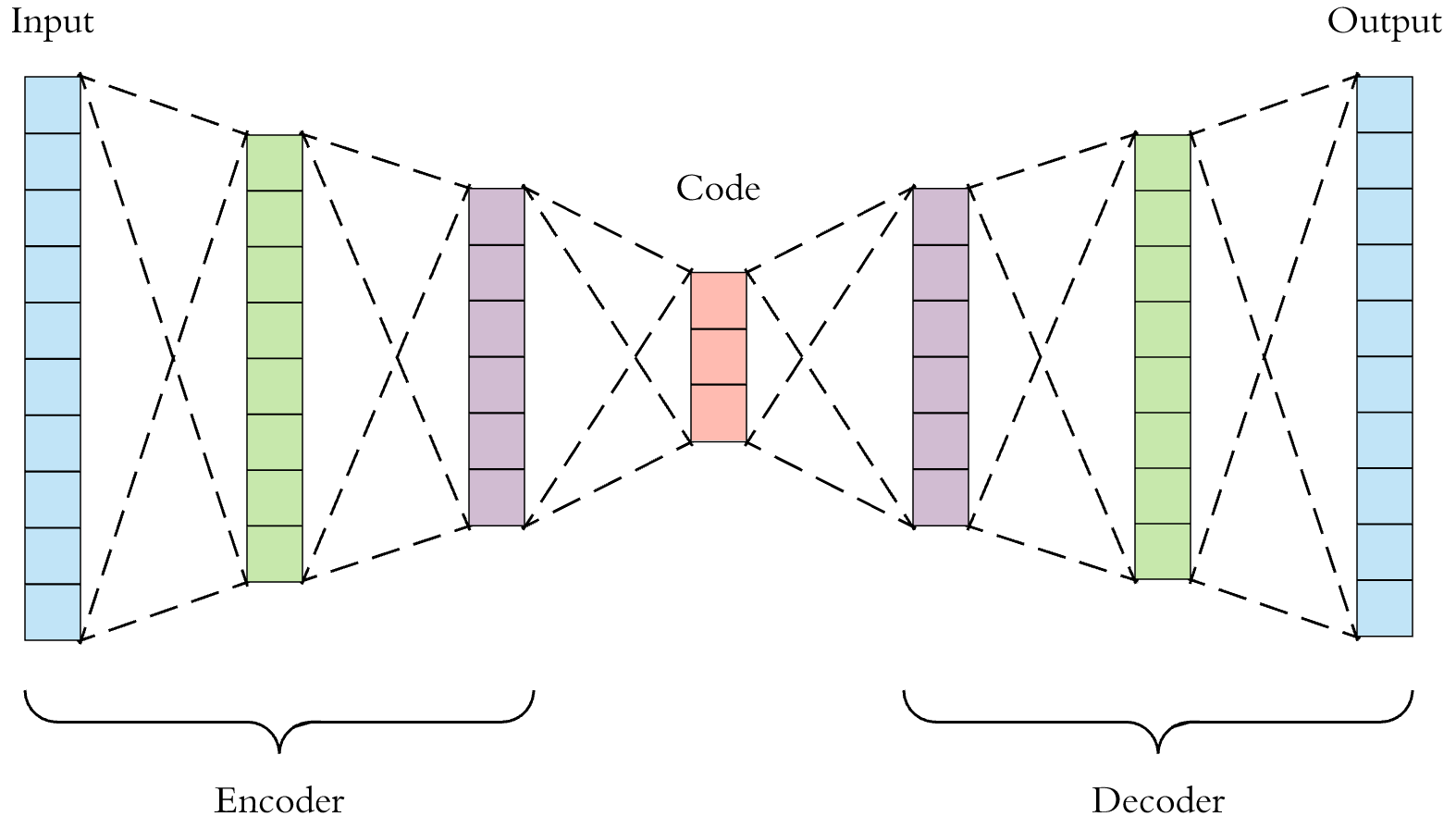
"" , , .
? , , , . , 9% 91% . , . , , : .
.
PyTorch, :
import torch
from torch import nn
from torch.utils.data import TensorDataset, DataLoader
from torch.optim import Adam
:
batch_size = 32
lr = 1e-3
. , , "" . , ( , learning rate, ) , , .
train_in_distribution = x_train[y_train == 0]
train_in_distribution = torch.tensor(train_in_distribution.astype(np.float32))
train_in_dataset = TensorDataset(train_in_distribution)
train_in_loader = DataLoader(train_in_dataset, batch_size=batch_size, shuffle=True)
test_dataset = TensorDataset(
torch.tensor(x_test.astype(np.float32)),
torch.tensor(y_test.astype(np.long))
)
test_loader = DataLoader(test_dataset, batch_size=batch_size, shuffle=False)
val_dataset = TensorDataset(torch.tensor(x_val.astype(np.float32)))
val_loader = DataLoader(val_dataset, batch_size=batch_size, shuffle=False)
. 4 , ( 2 : μ, — σ, ; 4 , ).
class Autoencoder(nn.Module):
def __init__(self, input_size):
super(Autoencoder, self).__init__()
self.encoder = nn.Sequential(
nn.Linear(input_size, 128),
nn.LeakyReLU(0.2),
nn.Linear(128, 64),
nn.LeakyReLU(0.2),
nn.Linear(64, 16),
nn.LeakyReLU(0.2),
nn.Linear(16, 4),
nn.LeakyReLU(0.2),
)
self.decoder = nn.Sequential(
nn.Linear(4, 16),
nn.LeakyReLU(0.2),
nn.Linear(16, 64),
nn.LeakyReLU(0.2),
nn.Linear(64, 128),
nn.LeakyReLU(0.2),
nn.Linear(128, 256),
nn.LeakyReLU(0.2),
)
def forward(self, x):
x = self.encoder(x)
x = self.decoder(x)
return x
model = Autoencoder(sample_size).cuda()
criterion = nn.MSELoss()
per_sample_criterion = nn.MSELoss(reduction="none")
optimizer = Adam(model.parameters(), lr=lr, weight_decay=1e-5)
- loss' , , boxplot' :
def save_score_distribution(model, data_loader, criterion, save_to, figsize=(8, 6)):
losses = []
labels = []
for (x_batch, y_batch) in data_loader:
x_batch = x_batch.cuda()
output = model(x_batch)
loss = criterion(output, x_batch)
loss = torch.mean(loss, dim=1)
loss = loss.detach().cpu().numpy().flatten()
losses.append(loss)
labels.append(y_batch.detach().cpu().numpy().flatten())
losses = np.concatenate(losses)
labels = np.concatenate(labels)
losses_0 = losses[labels == 0]
losses_1 = losses[labels == 1]
fig, ax = plt.subplots(1, figsize=figsize)
ax.boxplot([losses_0, losses_1])
ax.set_xticklabels(['normal', 'anomaly'])
plt.savefig(save_to)
plt.close(fig)
:
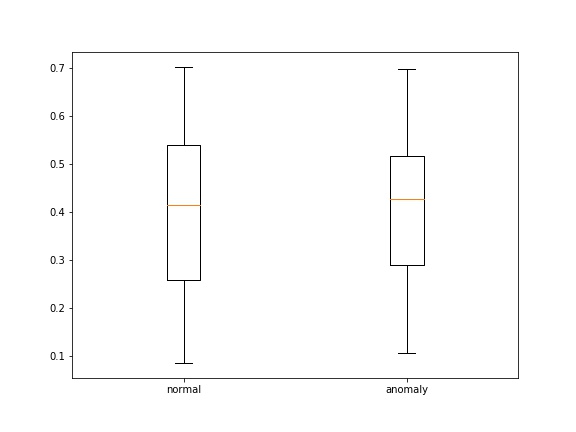
:
experiment_path = "ood_detection"
!rm -rf $experiment_path
os.makedirs(experiment_path, exist_ok=True)
epochs = 100
for epoch in range(epochs):
running_loss = 0
for (x_batch, ) in train_in_loader:
x_batch = x_batch.cuda()
output = model(x_batch)
loss = criterion(output, x_batch)
optimizer.zero_grad()
loss.backward()
optimizer.step()
running_loss += loss.item()
print("epoch [{}/{}], train loss:{:.4f}".format(epoch+1, epochs, running_loss))
plot_path = os.path.join(experiment_path, "{}.jpg".format(epoch+1))
save_score_distribution(model, test_loader, per_sample_criterion, plot_path)
>>>
epoch [1/100], train loss:8.5728
epoch [2/100], train loss:4.2405
epoch [3/100], train loss:4.0852
epoch [4/100], train loss:1.7578
epoch [5/100], train loss:0.8543
...
epoch [96/100], train loss:0.0147
epoch [97/100], train loss:0.0154
epoch [98/100], train loss:0.0117
epoch [99/100], train loss:0.0105
epoch [100/100], train loss:0.0097

50

100
, . , :
def get_prediction(model, x):
global batch_size
dataset = TensorDataset(torch.tensor(x.astype(np.float32)))
data_loader = DataLoader(dataset, batch_size=batch_size, shuffle=False)
predictions = []
for batch in data_loader:
x_batch = batch[0].cuda()
pred = model(x_batch)
predictions.append(pred.detach().cpu().numpy())
predictions = np.concatenate(predictions)
return predictions
def compare_data(xs, sample_num, data_range=(0, 1), labels=None):
fig, axes = plt.subplots(len(xs))
sample_size = len(xs[0][sample_num])
for i in range(len(xs)):
axes[i].plot(np.linspace(*data_range, sample_size), xs[i][sample_num])
if labels:
for i, label in enumerate(labels):
axes[i].set_ylabel(label)
:
x_test_pred = get_prediction(model, x_test)
compare_data([x_test[y_test == 0], x_test_pred[y_test == 0]], 10, labels=["real", "encoded"])
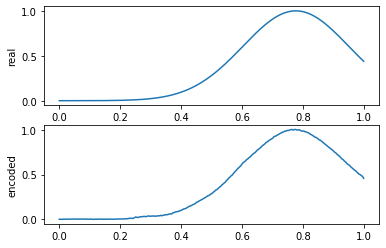
:

X
? .
Difference score
— . , , , . .
def get_difference_score(model, x):
global batch_size
dataset = TensorDataset(torch.tensor(x.astype(np.float32)))
data_loader = DataLoader(dataset, batch_size=batch_size, shuffle=False)
predictions = []
for (x_batch, ) in data_loader:
x_batch = x_batch.cuda()
preds = model(x_batch)
predictions.append(preds.detach().cpu().numpy())
predictions = np.concatenate(predictions)
return (x - predictions)
from sklearn.ensemble import RandomForestClassifier
test_score = get_difference_score(model, x_test)
score_forest = RandomForestClassifier(max_features=100)
score_forest.fit(test_score, y_test)
>>> RandomForestClassifier(bootstrap=True, ccp_alpha=0.0, class_weight=None,
criterion='gini', max_depth=None, max_features=100,
max_leaf_nodes=None, max_samples=None,
min_impurity_decrease=0.0, min_impurity_split=None,
min_samples_leaf=1, min_samples_split=2,
min_weight_fraction_leaf=0.0, n_estimators=100,
n_jobs=None, oob_score=False, random_state=None,
verbose=0, warm_start=False)
, : 2 — . , 2 : , — difference_score
, — . , , .
? . difference_score
, ( ) , , . , , difference_score
, ( ). .
:
val_score = get_difference_score(model, x_val)
prediction = score_forest.predict(val_score)
print(classification_report(y_val, prediction))
>>> precision recall f1-score support
0.0 0.76 1.00 0.87 100
1.0 1.00 0.69 0.82 100
accuracy 0.84 200
macro avg 0.88 0.84 0.84 200
weighted avg 0.88 0.84 0.84 200
. :
indices = np.arange(len(prediction))
wrong_indices = indices[(prediction == 0) & (y_val == 1)]
x_val_pred = get_prediction(model, x_val)
compare_data([x_val, x_val_pred], wrong_indices[0])
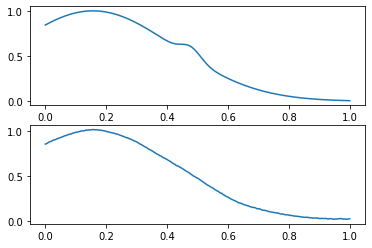
? :
plt.imshow(val_score[wrong_indices], norm=Normalize(0, 1, clip=True))
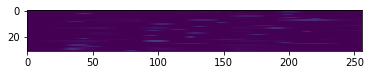
:
plt.imshow(val_score[(prediction == 1) & (y_val == 1)], norm=Normalize(0, 1, clip=True))
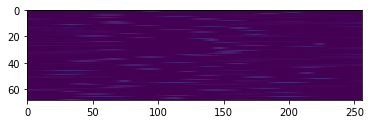
:
plt.imshow(val_score[(prediction == 0) & (y_val == 0)], norm=Normalize(0, 1, clip=True))
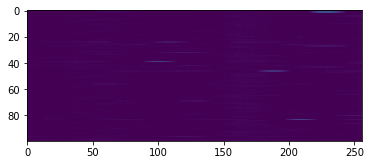
, : .
Difference histograms
. . — , "", .
, difference score
print("test score: [{}; {}]".format(test_score.min(), test_score.max()))
>>> test score: [-0.2260764424351479; 0.26339245919832344]
, :
def score_to_histograms(scores, bins=10, data_range=(-0.3, 0.3)):
result_histograms = np.zeros((len(scores), bins))
for i in range(len(scores)):
hist, bins = np.histogram(scores[i], bins=bins, range=data_range)
result_histograms[i] = hist
return result_histograms
test_histogram = score_to_histograms(test_score, bins=10, data_range=(-0.3, 0.3))
val_histogram = score_to_histograms(val_score, bins=10, data_range=(-0.3, 0.3))
plt.title("normal histogram")
plt.bar(np.linspace(-0.3, 0.3, 10), test_histogram[y_test == 0][0])
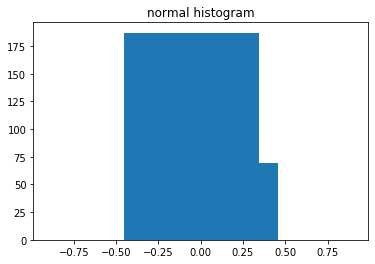
plt.title("anomaly histogram")
plt.bar(np.linspace(-0.3, 0.3, 10), test_histogram[y_test == 1][0])
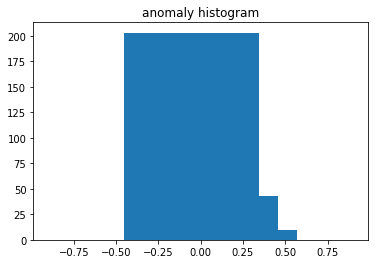
, "", .
histogram_forest = RandomForestClassifier(n_estimators=10)
histogram_forest.fit(test_histogram, y_test)
>>> RandomForestClassifier(bootstrap=True, ccp_alpha=0.0, class_weight=None,
criterion='gini', max_depth=None, max_features='auto',
max_leaf_nodes=None, max_samples=None,
min_impurity_decrease=0.0, min_impurity_split=None,
min_samples_leaf=1, min_samples_split=2,
min_weight_fraction_leaf=0.0, n_estimators=10,
n_jobs=None, oob_score=False, random_state=None,
verbose=0, warm_start=False)
val_prediction = histogram_forest.predict(val_histogram)
print(classification_report(y_val, val_prediction))
>>> precision recall f1-score support
0.0 0.83 0.99 0.90 100
1.0 0.99 0.80 0.88 100
accuracy 0.90 200
macro avg 0.91 0.90 0.89 200
weighted avg 0.91 0.90 0.89 200
— . , , , , . () .
— . ? VAE, ( 4 ) . . CVAE, - . , , , ..
GAN', (), . ( ).
, , .
Parametrik statistik
- GMM - pemodelan campuran Gaussian + Akaike atau Kriteria Informasi Bayesian
- HMM — Hidden Markov models
- MRF — Markov random fields
- CRF — conditional random fields
Robust statistic
- minimum volume estimation
- PCA
- estimation maximisation (EM) + deterministic annealing
- K-means
Non-parametric statistics
- histogram analysis with density estimation on KNN
- local kernel models (Parzen windowing)
- vector of feature matching with similarity distance (between train and test)
- wavelets + MMRF
- histogram-based measures features
- texture features
- shape features
- features from VGG-16
- HOG
Neural networks
- self organisation maps (SOM) or Kohonen's
- Radial Basis Functions (RBF) (Minhas, 2005)
- LearningVector Quantisation (LVQ)
- ProbabilisticNeural Networks (PNN)
- Hopfieldnetworks
- SupportVector Machines (SVM)
- AdaptiveResonance Theory (ART)
- Relevance vector machine (RVM)
, Data science' . Computer vision, . ( — !) — FARADAY Lab. — , .
c:
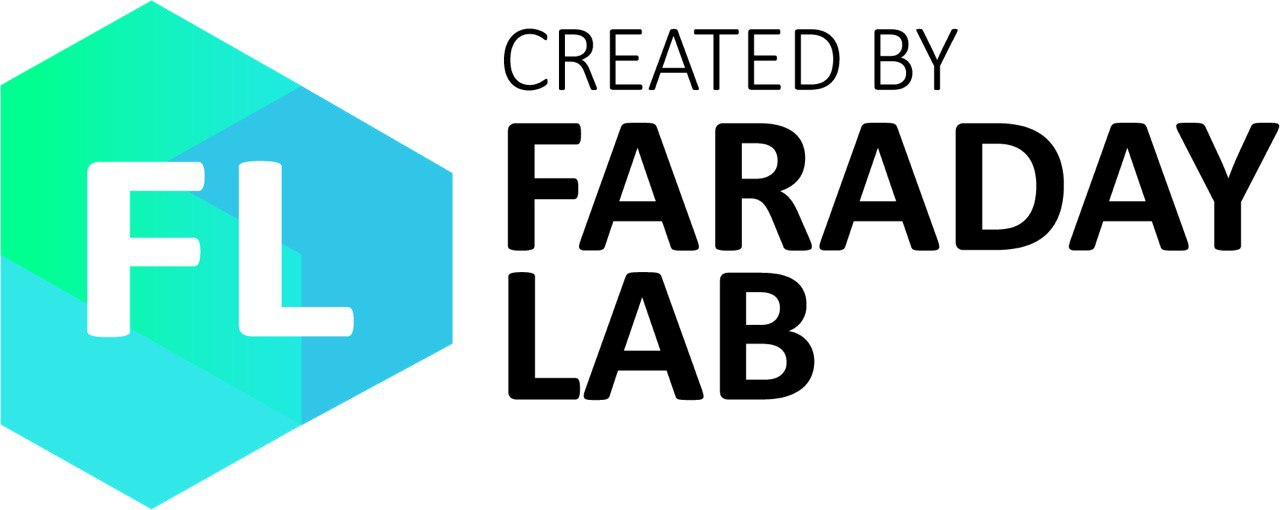