Dalam contoh kecil ini, saya ingin menunjukkan cara mem-parsing data dari situs dan bagaimana menggunakannya lebih lanjut untuk analisis. Untuk melakukan ini, saya mem-parsing tabel peringkat klan dari permainan World of Tanks dan melihat bagaimana peringkat klan dapat berkorelasi dengan data lainnya.
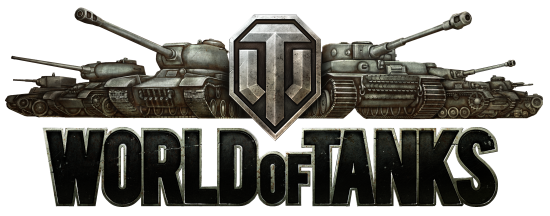
1. Parsing data
import numpy as np
import pandas as pd
from scrapy.selector import Selector
import matplotlib.pyplot as plt
import seaborn as sns
%matplotlib inline
sns.set(rc={'figure.figsize':(20, 5)})
javascript , - scrapy html , ( selenium) scrapy Selector
.
with open(' _ Wargaming.net.html', 'r') as f:
html_text = f.read()
selector = Selector(text=html_text)
xpath
- youtube.com, . , .. (. ), , text
, ( ), , , .
df = pd.DataFrame()
table = selector.xpath('//div[@class="js-widget-content"]/div[2]/div[2]/div') + selector.xpath('//div[@class="js-widget-content"]/div[2]/div[4]/div')
for row in table:
text = row.xpath('*//text()').extract()
clan = text[-10]
cr = int(text[-7].replace(' ',''))
wpr = int(text[-6].replace(' ',''))
abd = float(text[-5].replace(',', '.'))
avl_10 = int(text[-4])
fsh = int(text[-3].replace(' ',''))
wgm = int(text[-2].replace(' ',''))
wsh = int(text[-1].replace(' ',''))
df = df.append({'Clan' : clan,
'CR' : cr,
'wPR' : wpr,
'aB_D' : abd,
'aVL10' : avl_10,
'fSH' : fsh,
'wGM' : wgm,
'wSH' : wsh},
ignore_index=True)
df
, 25, 5 .
print('- :', len(df))
- : 25
df.head()
| CR | Clan | aB_D | aVL10 | fSH | wGM | wPR | wSH |
---|
0 | 15486.0 | [CM-1] | 18.20 | 34.0 | 4083.0 | 2253.0 | 10325.0 | 2294.0 |
---|
1 | 15148.0 | [R-BOY] | 18.86 | 37.0 | 3745.0 | 1943.0 | 10267.0 | 2066.0 |
---|
2 | 15041.0 | [CYS] | 17.47 | 32.0 | 3649.0 | 2300.0 | 10251.0 | 1857.0 |
---|
3 | 14984.0 | [I-YAN] | 16.85 | 28.0 | 4080.0 | 2468.0 | 8992.0 | 2290.0 |
---|
4 | 14952.0 | [YETT1] | 17.41 | 29.0 | 4222.0 | 2159.0 | 8387.0 | 2474.0 |
---|
2.
, - , . .
plt.xticks(rotation=45, ha="right")
ax = sns.lineplot(x='Clan', y='CR', data=df, marker='o', color='r', sort=False)
ax.set(xlabel='', ylabel=' ')
ax.set(xticks=df['Clan'].values);

. , ?
def draw_corr(df, y1, y1_label, y2='CR', y2_label=' '):
fig, ax = plt.subplots()
plt.xticks(rotation=45, ha="right")
sns.lineplot(x='Clan', y=y1, data=df, marker='o', color='b', label=y1_label, sort=False)
ax.set(xlabel='', ylabel=y1_label)
plt.legend(bbox_to_anchor=(0.01, 0.95), loc='upper left')
ax2 = ax.twinx()
sns.lineplot(x='Clan', y=y2, data=df, marker='o', color='r', label=y2_label, sort=False)
ax2.set(ylabel=y2_label)
plt.legend(bbox_to_anchor=(0.01, 0.85), loc='upper left');
draw_corr(df, 'wPR', ' ')

draw_corr(df, 'aVL10', ' ')

3.
Artikel ini ditulis khusus hanya untuk menunjukkan bahwa tidak ada yang rumit dalam penguraian dan analisis data. Dari data yang diperoleh, Anda dapat membuat lebih banyak grafik korelasi, membuat histogram, jika perlu, membuat beberapa prediksi, dll.
→ Kode Sumber