Jaringan saraf cukup populer. Keuntungan utama mereka adalah bahwa mereka dapat menggeneralisasi data yang agak rumit di mana algoritma lain menunjukkan kualitas rendah. Tetapi bagaimana jika kualitas jaringan saraf masih belum memuaskan?
Dan di sini ansambel datang untuk menyelamatkan ...
Apa itu ansambel
Ensembel algoritma pembelajaran mesin adalah penggunaan beberapa model (tidak harus berbeda), bukan satu. Artinya, pertama-tama kita melatih masing-masing model, dan kemudian menggabungkan prediksi mereka. Ternyata model kami bersama-sama membentuk satu model yang lebih kompleks (dalam hal kemampuan generalisasi - kemampuan untuk "memahami" data), yang sering disebut metamodel . Paling sering, metamodel dilatih bukan pada sampel data awal kami, tetapi pada prediksi model lain. Tampaknya memperhitungkan pengalaman semua model, dan ini membantu mengurangi kesalahan.
Rencana
- Pertama kita melihat satu model PyTorch sederhana dan mendapatkan kualitasnya.
- Kemudian kami akan mengumpulkan beberapa model menggunakan Scikit-belajar dan belajar bagaimana mencapai level yang lebih tinggi
Satu model tunggal
, Digits Sklearn, :
from sklearn.datasets import load_digits
import numpy as np
import matplotlib.pyplot as plt
x, y = load_digits(n_class=10, return_X_y=True)
, :
print(x.shape)
>>> (1797, 64)
print(np.unique(y))
>>> array([0, 1, 2, 3, 4, 5, 6, 7, 8, 9])
index = 0
print(y[index])
plt.imshow(x[index].reshape(8, 8), cmap="Greys")
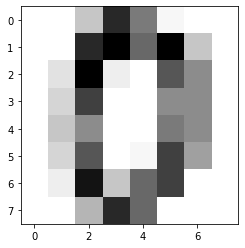
x[index]
>>> array([ 0., 0., 5., 13., 9., 1., 0., 0., 0., 0., 13., 15., 10.,
15., 5., 0., 0., 3., 15., 2., 0., 11., 8., 0., 0., 4.,
12., 0., 0., 8., 8., 0., 0., 5., 8., 0., 0., 9., 8.,
0., 0., 4., 11., 0., 1., 12., 7., 0., 0., 2., 14., 5.,
10., 12., 0., 0., 0., 0., 6., 13., 10., 0., 0., 0.])
, 0 15. , :
from sklearn.model_selection import train_test_split
from sklearn.preprocessing import StandardScaler
x_train, x_test, y_train, y_test = train_test_split(x, y, test_size=0.2,
shuffle=True, random_state=42)
print(x_train.shape)
>>> (1437, 64)
print(x_test.shape)
>>> (360, 64)
StandardScaler: -1 1. , β score score' :
scaler = StandardScaler()
scaler.fit(x_train)
:
x_train_scaled = scaler.transform(x_train)
x_test_scaled = scaler.transform(x_test)
Torch'!
import torch
from torch.utils.data import TensorDataset, DataLoader
import torch.nn as nn
import torch.nn.functional as F
from torch.optim import Adam
:
class SimpleCNN(nn.Module):
def __init__(self):
super(SimpleCNN, self).__init__()
self.conv1 = nn.Conv2d(in_channels=1, out_channels=3, kernel_size=5, stride=1, padding=2)
self.conv1_s = nn.Conv2d(in_channels=3, out_channels=3, kernel_size=5, stride=2, padding=2)
self.conv2 = nn.Conv2d(in_channels=3, out_channels=6, kernel_size=3, stride=1, padding=1)
self.conv2_s = nn.Conv2d(in_channels=6, out_channels=6, kernel_size=3, stride=2, padding=1)
self.conv3 = nn.Conv2d(in_channels=6, out_channels=10, kernel_size=3, stride=1, padding=1)
self.conv3_s = nn.Conv2d(in_channels=10, out_channels=10, kernel_size=3, stride=2, padding=1)
self.flatten = nn.Flatten()
self.fc1 = nn.Linear(10, 10)
def forward(self, x):
x = F.relu(self.conv1(x))
x = F.relu(self.conv1_s(x))
x = F.relu(self.conv2(x))
x = F.relu(self.conv2_s(x))
x = F.relu(self.conv3(x))
x = F.relu(self.conv3_s(x))
x = self.flatten(x)
x = self.fc1(x)
x = F.softmax(x)
return x
:
batch_size = 64
learning_rate = 1e-3
epochs = 200
, PyTorch:
x_train_tensor = torch.tensor(x_train_scaled.reshape(-1, 1, 8, 8).astype(np.float32))
x_test_tensor = torch.tensor(x_test_scaled.reshape(-1, 1, 8, 8).astype(np.float32))
y_train_tensor = torch.tensor(y_train.astype(np.long))
y_test_tensor = torch.tensor(y_test.astype(np.long))
PyTorch , β . β , X y ( ), DataLoader β , <X, y> 64 :
train_dataset = TensorDataset(x_train_tensor, y_train_tensor)
train_loader = DataLoader(train_dataset, batch_size=batch_size)
test_dataset = TensorDataset(x_test_tensor, y_test_tensor)
test_loader = DataLoader(test_dataset, batch_size=batch_size)
, :
simple_cnn = SimpleCNN().cuda()
criterion = nn.CrossEntropyLoss()
optimizer = Adam(simple_cnn.parameters(), lr=learning_rate)
:
for epoch in range(epochs):
simple_cnn.train()
train_samples_count = 0
true_train_samples_count = 0
running_loss = 0
for batch in train_loader:
x_data = batch[0].cuda()
y_data = batch[1].cuda()
y_pred = simple_cnn(x_data)
loss = criterion(y_pred, y_data)
optimizer.zero_grad()
loss.backward()
optimizer.step()
running_loss += loss.item()
y_pred = y_pred.argmax(dim=1, keepdim=False)
true_classified = (y_pred == y_data).sum().item()
true_train_samples_count += true_classified
train_samples_count += len(x_data)
train_accuracy = true_train_samples_count / train_samples_count
print(f"[{epoch}] train loss: {running_loss}, accuracy: {round(train_accuracy, 4)}")
simple_cnn.eval()
test_samples_count = 0
true_test_samples_count = 0
running_loss = 0
for batch in test_loader:
x_data = batch[0].cuda()
y_data = batch[1].cuda()
y_pred = simple_cnn(x_data)
loss = criterion(y_pred, y_data)
loss.backward()
running_loss += loss.item()
y_pred = y_pred.argmax(dim=1, keepdim=False)
true_classified = (y_pred == y_data).sum().item()
true_test_samples_count += true_classified
test_samples_count += len(x_data)
test_accuracy = true_test_samples_count / test_samples_count
print(f"[{epoch}] test loss: {running_loss}, accuracy: {round(test_accuracy, 4)}")
,- Torch' , . . 20 .
[180] train loss: 40.52328181266785, accuracy: 0.6966
[180] test loss: 10.813781499862671, accuracy: 0.6583
[181] train loss: 40.517325043678284, accuracy: 0.6966
[181] test loss: 10.811877608299255, accuracy: 0.6611
[182] train loss: 40.517088294029236, accuracy: 0.6966
[182] test loss: 10.814386487007141, accuracy: 0.6611
[183] train loss: 40.515315651893616, accuracy: 0.6966
[183] test loss: 10.812204122543335, accuracy: 0.6611
[184] train loss: 40.5108939409256, accuracy: 0.6966
[184] test loss: 10.808713555335999, accuracy: 0.6639
[185] train loss: 40.50885498523712, accuracy: 0.6966
[185] test loss: 10.80833113193512, accuracy: 0.6639
[186] train loss: 40.50892996788025, accuracy: 0.6966
[186] test loss: 10.809209108352661, accuracy: 0.6639
[187] train loss: 40.508036971092224, accuracy: 0.6966
[187] test loss: 10.806900978088379, accuracy: 0.6667
[188] train loss: 40.507275462150574, accuracy: 0.6966
[188] test loss: 10.79791784286499, accuracy: 0.6611
[189] train loss: 40.50368785858154, accuracy: 0.6966
[189] test loss: 10.799399137496948, accuracy: 0.6667
[190] train loss: 40.499858379364014, accuracy: 0.6966
[190] test loss: 10.795265793800354, accuracy: 0.6611
[191] train loss: 40.498780846595764, accuracy: 0.6966
[191] test loss: 10.796114206314087, accuracy: 0.6639
[192] train loss: 40.497228503227234, accuracy: 0.6966
[192] test loss: 10.790620803833008, accuracy: 0.6639
[193] train loss: 40.44325613975525, accuracy: 0.6973
[193] test loss: 10.657087206840515, accuracy: 0.7
[194] train loss: 39.62049174308777, accuracy: 0.7495
[194] test loss: 10.483307123184204, accuracy: 0.7222
[195] train loss: 39.24516046047211, accuracy: 0.7613
[195] test loss: 10.462445378303528, accuracy: 0.7278
[196] train loss: 39.16947162151337, accuracy: 0.762
[196] test loss: 10.488057255744934, accuracy: 0.7222
[197] train loss: 39.196797251701355, accuracy: 0.7634
[197] test loss: 10.502906918525696, accuracy: 0.7222
[198] train loss: 39.395434617996216, accuracy: 0.7537
[198] test loss: 10.354896545410156, accuracy: 0.7472
[199] train loss: 39.331292152404785, accuracy: 0.7509
[199] test loss: 10.367400050163269, accuracy: 0.7389
, : .
, : 74% . Not great, not terrible.
!
Bagging. : , , . : "" , - . .
sklearn ensembles
, . β , BaggingClassifier
:
import sklearn
from sklearn.ensemble import BaggingClassifier
. sklearn, β , sklearn.base.BaseEstimator
. , . fit
( ), predict_proba
, ( , ), predict
( ), .
, "" .
. , , .
-, ( 2 β get_params
set_params
), , . , net_type
, __init__
net_type
.
-, , , ( sklearn , ) copy
, deepcopy
. , , () , , .
class PytorchModel(sklearn.base.BaseEstimator):
def __init__(self, net_type, net_params, optim_type, optim_params, loss_fn,
input_shape, batch_size=32, accuracy_tol=0.02, tol_epochs=10,
cuda=True):
self.net_type = net_type
self.net_params = net_params
self.optim_type = optim_type
self.optim_params = optim_params
self.loss_fn = loss_fn
self.input_shape = input_shape
self.batch_size = batch_size
self.accuracy_tol = accuracy_tol
self.tol_epochs = tol_epochs
self.cuda = cuda
: fit
. , β , Loss, . : , ? . , 200 ( ). , . β , accuracy . , accuracy . , (tol_epochs
) ( accuracy , accuracy_tol
).
def fit(self, X, y):
self.net = self.net_type(**self.net_params)
if self.cuda:
self.net = self.net.cuda()
self.optim = self.optim_type(self.net.parameters(), **self.optim_params)
uniq_classes = np.sort(np.unique(y))
self.classes_ = uniq_classes
X = X.reshape(-1, *self.input_shape)
x_tensor = torch.tensor(X.astype(np.float32))
y_tensor = torch.tensor(y.astype(np.long))
train_dataset = TensorDataset(x_tensor, y_tensor)
train_loader = DataLoader(train_dataset, batch_size=self.batch_size,
shuffle=True, drop_last=False)
last_accuracies = []
epoch = 0
keep_training = True
while keep_training:
self.net.train()
train_samples_count = 0
true_train_samples_count = 0
for batch in train_loader:
x_data, y_data = batch[0], batch[1]
if self.cuda:
x_data = x_data.cuda()
y_data = y_data.cuda()
y_pred = self.net(x_data)
loss = self.loss_fn(y_pred, y_data)
self.optim.zero_grad()
loss.backward()
self.optim.step()
y_pred = y_pred.argmax(dim=1, keepdim=False)
true_classified = (y_pred == y_data).sum().item()
true_train_samples_count += true_classified
train_samples_count += len(x_data)
train_accuracy = true_train_samples_count / train_samples_count
last_accuracies.append(train_accuracy)
if len(last_accuracies) > self.tol_epochs:
last_accuracies.pop(0)
if len(last_accuracies) == self.tol_epochs:
accuracy_difference = max(last_accuracies) - min(last_accuracies)
if accuracy_difference <= self.accuracy_tol:
keep_training = False
. β , Loss, - :
def predict_proba(self, X, y=None):
X = X.reshape(-1, *self.input_shape)
x_tensor = torch.tensor(X.astype(np.float32))
if y:
y_tensor = torch.tensor(y.astype(np.long))
else:
y_tensor = torch.zeros(len(X), dtype=torch.long)
test_dataset = TensorDataset(x_tensor, y_tensor)
test_loader = DataLoader(test_dataset, batch_size=self.batch_size,
shuffle=False, drop_last=False)
self.net.eval()
predictions = []
for batch in test_loader:
x_data, y_data = batch[0], batch[1]
if self.cuda:
x_data = x_data.cuda()
y_data = y_data.cuda()
y_pred = self.net(x_data)
predictions.append(y_pred.detach().cpu().numpy())
predictions = np.concatenate(predictions)
return predictions
:
def predict(self, X, y=None):
predictions = self.predict_proba(X, y)
predictions = predictions.argmax(axis=1)
return predictions
class PytorchModel(sklearn.base.BaseEstimator):
def __init__(self, net_type, net_params, optim_type, optim_params, loss_fn,
input_shape, batch_size=32, accuracy_tol=0.02, tol_epochs=10,
cuda=True):
self.net_type = net_type
self.net_params = net_params
self.optim_type = optim_type
self.optim_params = optim_params
self.loss_fn = loss_fn
self.input_shape = input_shape
self.batch_size = batch_size
self.accuracy_tol = accuracy_tol
self.tol_epochs = tol_epochs
self.cuda = cuda
def fit(self, X, y):
self.net = self.net_type(**self.net_params)
if self.cuda:
self.net = self.net.cuda()
self.optim = self.optim_type(self.net.parameters(), **self.optim_params)
uniq_classes = np.sort(np.unique(y))
self.classes_ = uniq_classes
X = X.reshape(-1, *self.input_shape)
x_tensor = torch.tensor(X.astype(np.float32))
y_tensor = torch.tensor(y.astype(np.long))
train_dataset = TensorDataset(x_tensor, y_tensor)
train_loader = DataLoader(train_dataset, batch_size=self.batch_size,
shuffle=True, drop_last=False)
last_accuracies = []
epoch = 0
keep_training = True
while keep_training:
self.net.train()
train_samples_count = 0
true_train_samples_count = 0
for batch in train_loader:
x_data, y_data = batch[0], batch[1]
if self.cuda:
x_data = x_data.cuda()
y_data = y_data.cuda()
y_pred = self.net(x_data)
loss = self.loss_fn(y_pred, y_data)
self.optim.zero_grad()
loss.backward()
self.optim.step()
y_pred = y_pred.argmax(dim=1, keepdim=False)
true_classified = (y_pred == y_data).sum().item()
true_train_samples_count += true_classified
train_samples_count += len(x_data)
train_accuracy = true_train_samples_count / train_samples_count
last_accuracies.append(train_accuracy)
if len(last_accuracies) > self.tol_epochs:
last_accuracies.pop(0)
if len(last_accuracies) == self.tol_epochs:
accuracy_difference = max(last_accuracies) - min(last_accuracies)
if accuracy_difference <= self.accuracy_tol:
keep_training = False
def predict_proba(self, X, y=None):
X = X.reshape(-1, *self.input_shape)
x_tensor = torch.tensor(X.astype(np.float32))
if y:
y_tensor = torch.tensor(y.astype(np.long))
else:
y_tensor = torch.zeros(len(X), dtype=torch.long)
test_dataset = TensorDataset(x_tensor, y_tensor)
test_loader = DataLoader(test_dataset, batch_size=self.batch_size,
shuffle=False, drop_last=False)
self.net.eval()
predictions = []
for batch in test_loader:
x_data, y_data = batch[0], batch[1]
if self.cuda:
x_data = x_data.cuda()
y_data = y_data.cuda()
y_pred = self.net(x_data)
predictions.append(y_pred.detach().cpu().numpy())
predictions = np.concatenate(predictions)
return predictions
def predict(self, X, y=None):
predictions = self.predict_proba(X, y)
predictions = predictions.argmax(axis=1)
return predictions
, :
base_model = PytorchModel(net_type=SimpleCNN, net_params=dict(), optim_type=Adam,
optim_params={"lr": 1e-3}, loss_fn=nn.CrossEntropyLoss(),
input_shape=(1, 8, 8), batch_size=32, accuracy_tol=0.02,
tol_epochs=10, cuda=True)
base_model.fit(x_train_scaled, y_train)
preds = base_model.predict(x_test_scaled)
true_classified = (preds == y_test).sum()
test_accuracy = true_classified / len(y_test)
print(f"Test accuracy: {test_accuracy}")
>>> Test accuracy: 0.7361111111111112
.
meta_classifier = BaggingClassifier(base_estimator=base_model, n_estimators=10)
meta_classifier.fit(x_train_scaled.reshape(-1, 64), y_train)
>>> BaggingClassifier(
base_estimator=PytorchModel(accuracy_tol=0.02, batch_size=32,
cuda=True, input_shape=(1, 8, 8),
loss_fn=CrossEntropyLoss(),
net_params={},
net_type=<class '__main__.SimpleCNN'>,
optim_params={'lr': 0.001},
optim_type=<class 'torch.optim.adam.Adam'>,
tol_epochs=10),
bootstrap=True, bootstrap_features=False, max_features=1.0,
max_samples=1.0, n_estimators=10, n_jobs=None,
oob_score=False, random_state=None, verbose=0,
warm_start=False)
score
accuracy :
print(meta_classifier.score(x_test_scaled.reshape(-1, 64), y_test))
>>> 0.95
, . . . , , ( "" - ).
?
-, . , Loss' - .
-, Boosting. Bagging' , , . ( ) . sklearn, Loss - .
, . , "" . , .
, . β - , , , , , .
. , Data science' . Computer vision, . ( β !) β FARADAY Lab. β , .
c: