Pelatihan jaringan saraf dalam pengenalan pola adalah proses yang panjang dan intensif sumber daya. Terutama ketika di tangan hanya ada laptop murah, dan bukan komputer dengan kartu grafis yang kuat. Dalam hal ini, Google Colaboratory akan datang untuk menyelamatkan , yang menawarkan untuk menggunakan GPU level Tesla K80 benar-benar gratis ( selengkapnya ).
Artikel ini menjelaskan proses mempersiapkan data, melatih model tensorflow di Google Colaboratory dan peluncurannya di perangkat android.
Persiapan data
Sebagai contoh, mari kita coba latih jaringan saraf untuk mengenali dadu putih dengan latar belakang hitam. Karenanya, sebagai permulaan, Anda perlu membuat kumpulan data yang cukup untuk pelatihan (untuk saat ini, mari berhenti di ~ 100 foto).

Untuk pelatihan, kami akan menggunakan Tensorflow Object Detection API . Kami akan menyiapkan semua data yang diperlukan untuk pelatihan di laptop. Kita membutuhkan manajer lingkungan dan manajemen ketergantungan . Petunjuk instalasi di sini .
Mari kita ciptakan lingkungan kerja:
conda create -n object_detection_prepare pip python=3.6
Dan aktifkan:
conda activate object_detection_prepare
Atur dependensi yang kita butuhkan:
pip install --ignore-installed --upgrade tensorflow==1.14
pip install --ignore-installed pandas
pip install --ignore-installed Pillow
pip install lxml
conda install pyqt=5
object_detection, object_detection/images.
Google Colab , , "tcmalloc: large alloc....".
object_detection/preprocessing .
:
python ./object_detection/preprocessing/image_resize.py -i ./object_detection/images --imageWidth=800 --imageHeight=600
, 800x600 object_detection/images/resized. object_detection/images.
labelImg.
labelImg object_detection
labelImg
cd [FULL_PATH]/object_detection/labelImg
:
pyrcc5 -o libs/resources.py resources.qrc
( ):
python labelImg.py

βOpen dirβ object_detection/images , . (1, 2, 3, 4, 5, 6). ( *.xml) .
object_detection/training_demo, Google Colab .
( ) 80/20 object_detection/training_demo/images/train object_detection/training_demo/images/test.
object_detection/training_demo/annotations, . label_map.pbtxt, . :
label_map.pbtxtitem {
id: 1
name: '1'
}
item {
id: 2
name: '2'
}
item {
id: 3
name: '3'
}
item {
id: 4
name: '4'
}
item {
id: 5
name: '5'
}
item {
id: 6
name: '6'
}
, ? , TFRecord. [1].
: xml -> csv csv -> record
preprocessing :
cd [FULL_PATH]\object_detection\preprocessing
1. xml csv
:
python xml_to_csv.py -i [FULL_PATH]/object_detection/training_demo/images/train -o [FULL_PATH]/object_detection/training_demo/annotations/train_labels.csv
:
python xml_to_csv.py -i [FULL_PATH]/object_detection/training_demo/images/test -o [FULL_PATH]/object_detection/training_demo/annotations/test_labels.csv
2. csv record
:
python generate_tfrecord.py --label_map_path=[FULL_PATH]\object_detection\training_demo\annotations\label_map.pbtxt --csv_input=[FULL_PATH]\object_detection\training_demo\annotations\train_labels.csv --output_path=[FULL_PATH]\object_detection\training_demo\annotations\train.record --img_path=[FULL_PATH]\object_detection\training_demo\images\train
:
python generate_tfrecord.py --label_map_path=[FULL_PATH]\object_detection\training_demo\annotations\label_map.pbtxt --csv_input=[FULL_PATH]\object_detection\training_demo\annotations\test_labels.csv --output_path=[FULL_PATH]\object_detection\training_demo\annotations\test.record --img_path=[FULL_PATH]\object_detection\training_demo\images\test
, , .
.
ssdlite_mobilenet_v2_coco, android .
object_detection/training_demo/pre-trained-model.
-
object_detection/training_demo/pre-trained-model/ssdlite_mobilenet_v2_coco_2018_05_09
pipeline.config object_detection/training_demo/training ssdlite_mobilenet_v2_coco.config.
, :
1.
model.ssd.num_classes: 6
2. ( ), ,
train_config.batch_size: 18
train_config.num_steps: 20000
train_config.fine_tune_checkpoint:"./training_demo/pre-trained-model/ssdlite_mobilenet_v2_coco_2018_05_09/model.ckpt"
3. (object_detection/training_demo/images/train)
eval_config.num_examples: 64
4.
train_input_reader.label_map_path: "./training_demo/annotations/label_map.pbtxt"
train_input_reader.tf_record_input_reader.input_path:"./training_demo/annotations/train.record"
5.
eval_input_reader.label_map_path: "./training_demo/annotations/label_map.pbtxt"
eval_input_reader.tf_record_input_reader.input_path:"./training_demo/annotations/test.record"
, .
training_demo training_demo.zip Google Drive.
google drive Google Colab,
, .
Google Drive training_demo.zip, Get shareable link id :
drive.google.com/open?id=[YOUR_FILE_ID_HERE]
Google Colab β Google Drive.

CPU. GPU, runtime.


.
:
1. TensorFlow Models:
!git clone https://github.com/tensorflow/models.git
2. protobuf object_detection:
!apt-get -qq install libprotobuf-java protobuf-compiler
%cd ./models/research/
!protoc object_detection/protos/*.proto --python_out=.
%cd ../..
3. PYTHONPATH:
import os
os.environ['PYTHONPATH'] += ":/content/models/research/"
os.environ['PYTHONPATH'] += ":/content/models/research/slim"
os.environ['PYTHONPATH'] += ":/content/models/research/object_detection"
os.environ['PYTHONPATH'] += ":/content/models/research/object_detection/utils"
4. Google Drive PyDrive :
!pip install -U -q PyDrive
from pydrive.auth import GoogleAuth
from pydrive.drive import GoogleDrive
from google.colab import auth
from oauth2client.client import GoogleCredentials
auth.authenticate_user()
gauth = GoogleAuth()
gauth.credentials = GoogleCredentials.get_application_default()
drive = GoogleDrive(gauth)
5. ( id ) :
drive_file_id="[YOUR_FILE_ID_HERE]"
training_demo_zip = drive.CreateFile({'id': drive_file_id})
training_demo_zip.GetContentFile('training_demo.zip')
!unzip training_demo.zip
!rm training_demo.zip
6. :
!python ./models/research/object_detection/legacy/train.py --logtostderr --train_dir=./training_demo/training --pipeline_config_path=./training_demo/training/ssdlite_mobilenet_v2_coco.config
--train_dir=./training_demo/training β ,
--pipeline_config_path=./training_demo/training/ssdlite_mobilenet_v2_coco.config β
7. frozen graph, :
!python /content/models/research/object_detection/export_inference_graph.py --input_type image_tensor --pipeline_config_path /content/training_demo/training/ssdlite_mobilenet_v2_coco.config --trained_checkpoint_prefix /content/training_demo/training/model.ckpt-[CHECKPOINT_NUMBER]
--output_directory /content/training_demo/training/output_inference_graph_v1.pb
--pipeline_config_path /content/training_demo/training/ssdlite_mobilenet_v2_coco.config β
--trained_checkpoint_prefix /content/training_demo/training/model.ckpt-[CHECKPOINT_NUMBER] β , .
--output_directory /content/training_demo/training/output_inference_graph_v1.pb β
[CHECKPOINT_NUMBER], content/training_demo/training/. model.ckpt-1440.index, model.ckpt-1440.meta. 1440 β [CHECKPOINT_NUMBER] .

. ~20000 .

8. tflite.
tensorflow lite tflite. frozen graph tflite ( export_inference_graph.py):
!python /content/models/research/object_detection/export_tflite_ssd_graph.py --pipeline_config_path /content/training_demo/training/ssdlite_mobilenet_v2_coco.config --trained_checkpoint_prefix /content/training_demo/training/model.ckpt-[CHECKPOINT_NUMBER] --output_directory /content/training_demo/training/output_inference_graph_tf_lite.pb
tflite , output_inference_graph_tf_lite.pb:

Netron. .
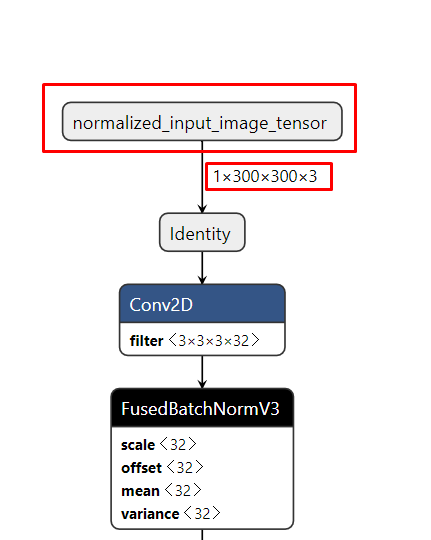

pb tflite :
!tflite_convert --output_file=/content/training_demo/training/model_q.tflite --graph_def_file=/content/training_demo/training/output_inference_graph_tf_lite_v1.pb/tflite_graph.pb --input_arrays=normalized_input_image_tensor --output_arrays='TFLite_Detection_PostProcess','TFLite_Detection_PostProcess:1','TFLite_Detection_PostProcess:2','TFLite_Detection_PostProcess:3' --input_shapes=1,300,300,3 --enable_select_tf_ops --allow_custom_ops --inference_input_type=QUANTIZED_UINT8 --inference_type=FLOAT --mean_values=128 --std_dev_values=128
--output_file=/content/training_demo/training/model_q.tflite β
--graph_def_file=/content/training_demo/training/output_inference_graph_tf_lite_v1.pb/tflite_graph.pb β frozen graph,
--input_arrays=normalized_input_image_tensor β ,
--output_arrays='TFLite_Detection_PostProcess','TFLite_Detection_PostProcess:1','TFLite_Detection_PostProcess:2','TFLite_Detection_PostProcess:3' β ,
--input_shapes=1,300,300,3 β ,
--enable_select_tf_ops β runtime TensorFlow Lite
--allow_custom_ops β TensorFlow Lite Optimizing Converter
--inference_type=FLOAT β
--inference_input_type=QUANTIZED_UINT8 β
--mean_values=128 --std_dev_values=128 β , QUANTIZED_UINT8
Google Drive:
!zip -r ./training_demo/training.zip ./training_demo/training/
training_result = drive.CreateFile({'title': 'training_result.zip'})
training_result.SetContentFile('training_demo/training.zip')
training_result.Upload()
Invalid client secrets file, google drive.
android
android object detection, kotlin CameraX. .
CameraX ImageAnalysis. ObjectDetectorAnalyzer.
:
1. YUV . RGB :
val rgbArray = convertYuvToRgb(image)
2. (, , , 300x300), Bitmap :
val rgbBitmap = getRgbBitmap(rgbArray, image.width, image.height)
val transformation = getTransformation(rotationDegrees, image.width, image.height)
Canvas(resizedBitmap).drawBitmap(rgbBitmap, transformation, null)
3. bitmap , :
ImageUtil.storePixels(resizedBitmap, inputArray)
val objects = detect(inputArray)
4. RecognitionResultOverlayView :
val scaleFactorX = measuredWidth / result.imageWidth.toFloat()
val scaleFactorY = measuredHeight / result.imageHeight.toFloat()
result.objects.forEach { obj ->
val left = obj.location.left * scaleFactorX
val top = obj.location.top * scaleFactorY
val right = obj.location.right * scaleFactorX
val bottom = obj.location.bottom * scaleFactorY
canvas.drawRect(left, top, right, bottom, boxPaint)
canvas.drawText(obj.text, left, top - 25f, textPaint)
}
, assets training_demo/training/model_q.tflite ( detect.tflite) labelmap.txt, :
SSD Mobilenet V1, 1, 0, labelOffset 1 0 collectDetectionResult ObjectDetector.
.
, Xiaomi Redmi 4X :
: