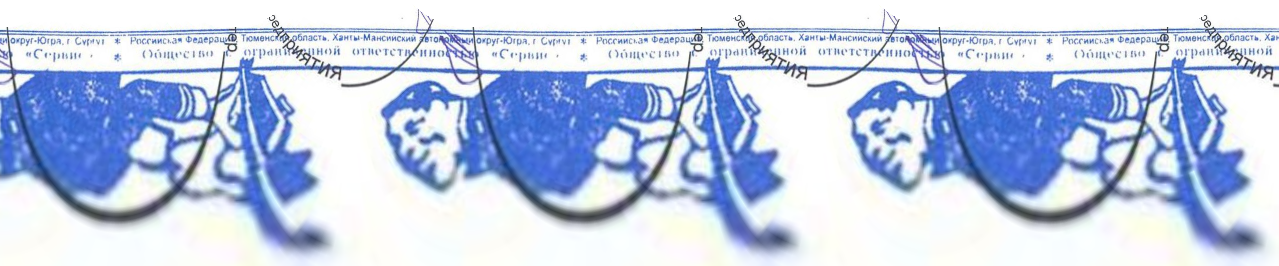
Belum lama ini, kami dihadapkan dengan tugas menemukan dan mengambil prangko dari dokumen. Untuk apa? Misalnya, untuk memverifikasi keberadaan segel dalam kontrak dari dua pihak (pihak dalam kontrak). Kami di dalam nampan sudah memiliki prototipe untuk menemukannya, ditulis dalam OpenCV, tetapi basah. Kami memutuskan untuk menggali peninggalan ini, menyingkirkan debu dari sana dan membuat solusi yang berfungsi atas dasar itu.
Sebagian besar teknik yang dijelaskan di sini dapat diterapkan di luar pencarian prangko. Contohnya:
- segmentasi warna;
- mencari benda bulat / lingkaran;
- konversi gambar ke sistem koordinat polar;
- persimpangan objek, Persimpangan over Union (IoU, koefisien Jacquard).
Sebagai hasilnya, kami memiliki dua opsi: untuk menyelesaikan menggunakan jaringan saraf atau untuk menghidupkan kembali prototipe pada OpenCV. Mengapa kami memutuskan untuk menggunakan OpenCV? Jawabannya ada di akhir artikel.
Contoh kode akan disajikan dalam Python dan C #. Untuk Python, Anda memerlukan OpenCV-python dan numpy paket , untuk C # Anda perlu OpenCvSharp dan OpenCV .
Semoga Anda terbiasa dengan algoritma OpenCV dasar. Saya tidak akan terjun jauh ke dalamnya, karena kebanyakan dari mereka layak mendapatkan artikel yang terpisah dan terperinci, tetapi saya akan meninggalkan tautan dengan gelaran. bagian dari semua haus.
, ( HLS-), . - , , .
. β .
, ? .
. / , , -, , .
, , python . ( , , ). ( Intersection over Union), .
:
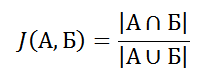
:
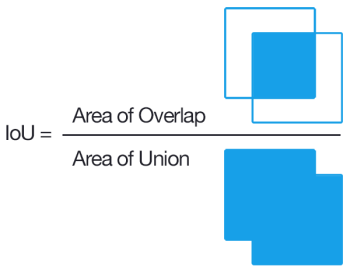
, , , , - . , , , :
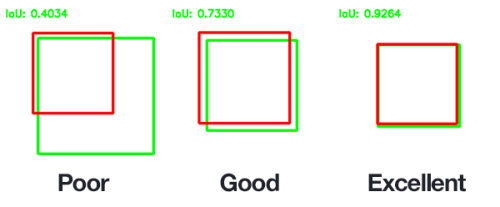
( 0.6) , F1- ( β ).
IoUimport sys
from collections import namedtuple
Metrics = namedtuple("metrics", ["tp", "fp", "fn"])
def bb_intersection_over_union(a, b):
x_a = max(a[0], b[0])
y_a = max(a[1], b[1])
x_b = min(a[2], b[2])
y_b = min(a[3], b[3])
inter_area = max(0, x_b - x_a + 1) * max(0, y_b - y_a + 1)
a_area = (a[2] - a[0] + 1) * (a[3] - a[1] + 1)
b_area = (b[2] - b[0] + 1) * (b[3] - b[1] + 1)
iou = inter_area / float(a_area + b_area - inter_area)
return iou
def same_stamp(a, b):
""", """
iou = bb_intersection_over_union(a, b)
print(f'iou: {iou}')
return iou > 0.6
def compare_stamps(extracted, mapped):
"""
:param extracted: , .
:param mapped: () .
"""
tp = []
fp = []
fn = list(mapped)
for stamp in extracted:
for check in fn:
if same_stamp(stamp, check):
tp.append(check)
fn.remove(check)
break
else:
fp.append(stamp)
return Metrics(len(tp), len(fp), len(fn))
def compare(file, sectors):
"""
.
:param file: .
:param sectors: .
"""
print(f'file: {file}')
try:
stamps = extract_stamps(file)
metrics = compare_stamps(stamps, [ss for ss in sectors if 'stamp' in ss['tags']])
return file, metrics
except:
print(sys.exc_info())
return file, Metrics(0, 0, 0)
if __name__ == '__main__':
file_metrics = {}
for file, sectors in dataset.items():
file_metrics[file] = compare(file, sectors)
total_metrics = Metrics(*(sum(x) for x in zip(*file_metrics.values())))
precision = total_metrics.tp / (total_metrics.tp + total_metrics.fp) if total_metrics.tp > 0 else 0
recall = total_metrics.tp / (total_metrics.tp + total_metrics.fn) if total_metrics.tp > 0 else 0
f1 = 2 * precision * recall / (precision + recall) if (precision + recall) > 0 else 0
print('precision\trecall\tf1')
print('{:.4f}\t{:.4f}\t{:.4f}'.format(precision * 100, recall * 100, f1 * 100).replace('.', ','))
print('tp\tfp\tfn')
print('{}\t{}\t{}'.format(total_metrics.tp, total_metrics.fp, total_metrics.fn))
print('tp\tfp\tfn')
for file in dataset.keys():
metric = file_metrics.get(file)
print(f'{metric.tp}\t{metric.fp}\t{metric.fn}')
print(f'precision: {precision}, recall: {recall}, f1: {f1}, {total_metrics}')
HLS-
() - . , . ( ):
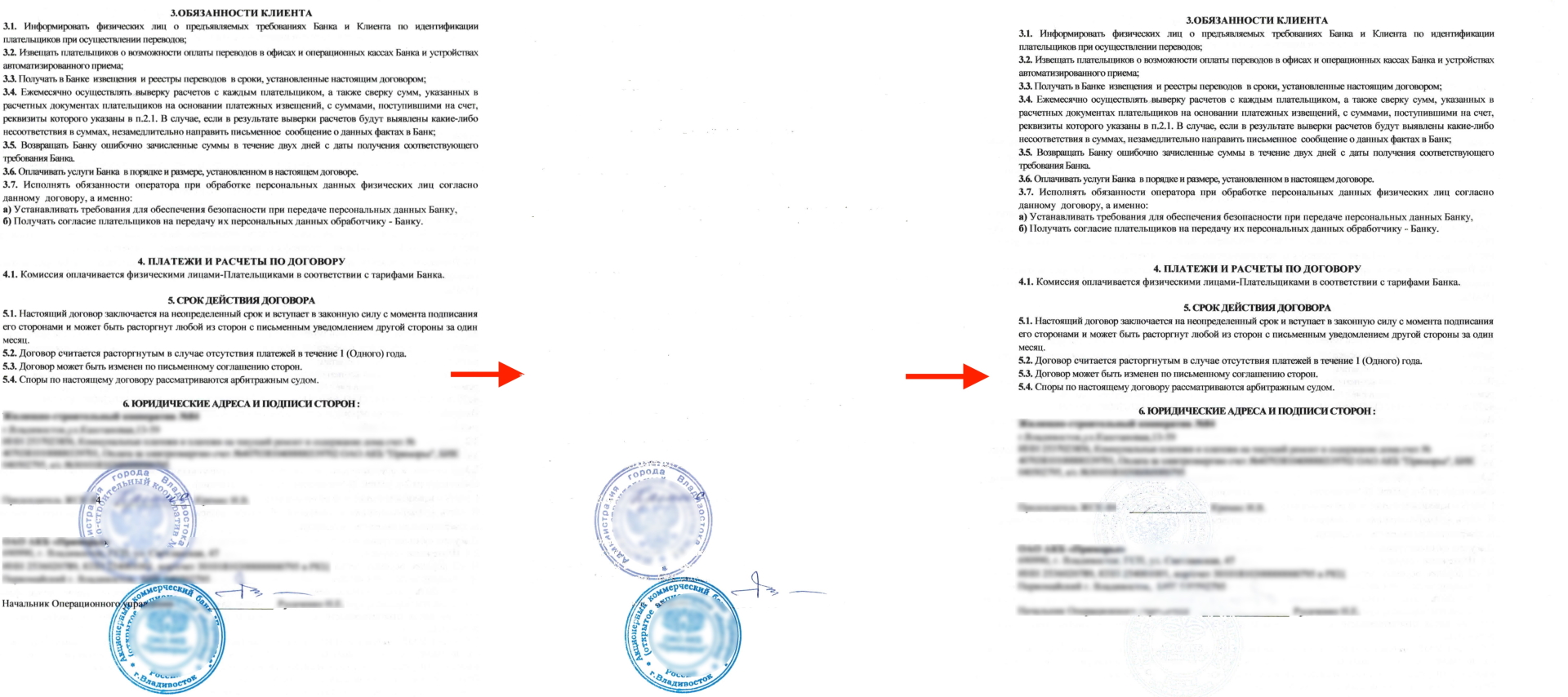
.
HLS . Hue, Lightness, Saturation, , , . :
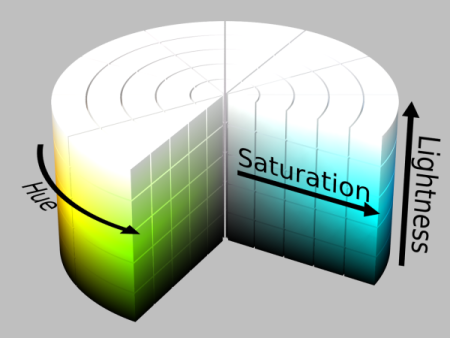
? - . , ~180 ~280.
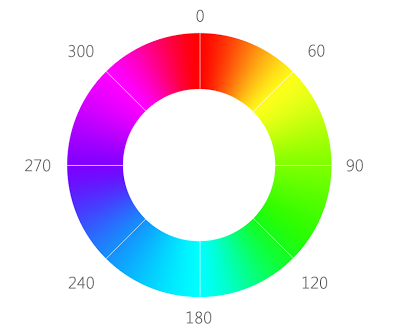
, - , , .
(Python)def colored_mask(img, threshold = -1):
denoised = cv2.medianBlur(img, 3)
cv2.imwrite('denoised.bmp', denoised)
gray = cv2.cvtColor(denoised, cv2.COLOR_BGR2GRAY)
cv2.imwrite('gray.bmp', gray)
adaptiveThreshold = threshold if threshold >= 0 else cv2.mean(img)[0]
color = cv2.cvtColor(denoised, cv2.COLOR_BGR2HLS)
mask = cv2.inRange(color, (0, int(adaptiveThreshold / 6), 60), (180, adaptiveThreshold, 255))
dst = cv2.bitwise_and(gray, gray, mask=mask)
cv2.imwrite('colors_mask.bmp', dst)
return dst
(C#)private void ColoredMask(Mat src, Mat dst, double threshold = -1)
{
using (var gray = new Mat())
using (var color = new Mat())
using (var mask = new Mat())
using (var denoised = new Mat())
{
Cv2.MedianBlur(src, denoised, 3);
denoised.Save("colors_denoised.bmp");
Cv2.CvtColor(denoised, gray, ColorConversionCodes.BGR2GRAY);
gray.Save("colors_gray.bmp");
var adaptiveThreshold = threshold < 0 ? src.Mean()[0] : threshold;
Cv2.CvtColor(denoised, color, ColorConversionCodes.BGR2HLS);
Cv2.InRange(color, new Scalar(0, adaptiveThreshold / 6, 60), new Scalar(180, adaptiveThreshold, 255), mask);
Cv2.BitwiseAnd(gray, gray, dst, mask);
dst.Save("colors_mask.bmp");
}
}
:
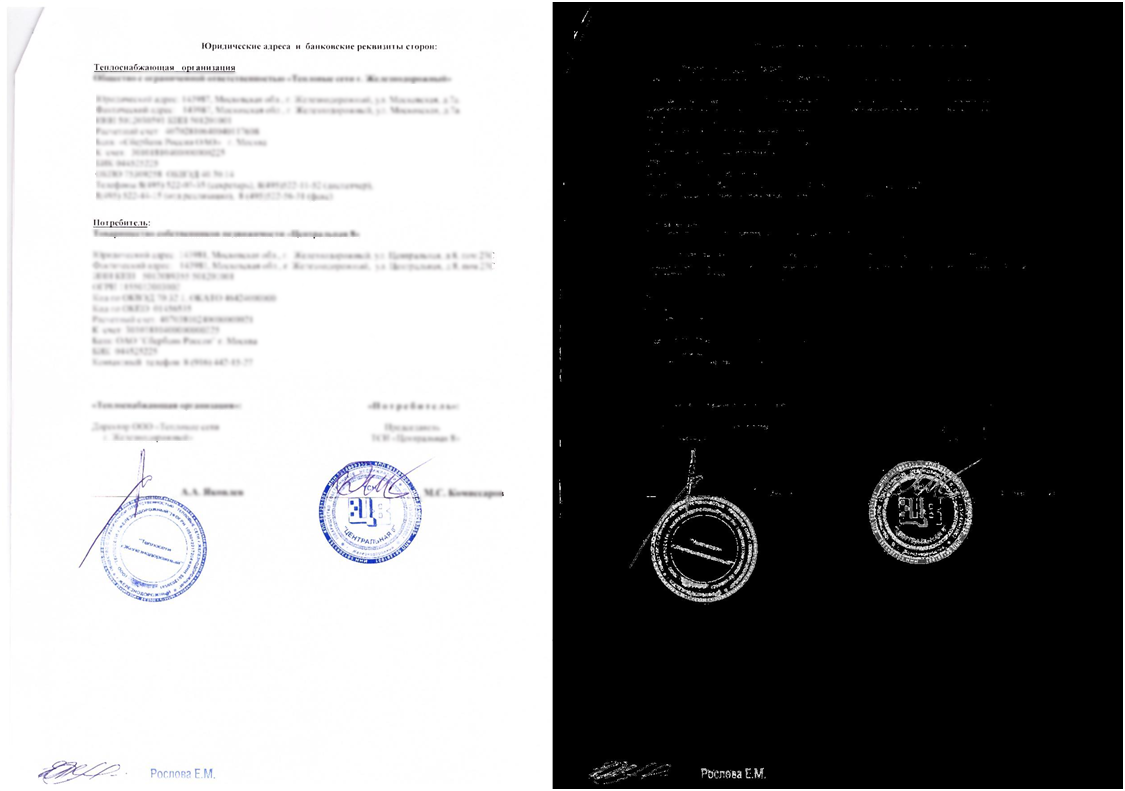
, HLS OpenCV 0 360, 0 180. β uint8/uchar/byte, 0 255, 360 2.
, , ? , , β β.
, . , ( ) , , β . , .
, , . OpenCV , , . , , .
(Python)
circles = cv2.HoughCircles(mask, cv2.HOUGH_GRADIENT, 1, 20, param1, param2, minRadius, maxRadius)
circles = np.uint16(np.around(circles))
cimg = cv2.cvtColor(img,cv2.COLOR_GRAY2BGR)
for i in circles[0,:]:
cv2.circle(cimg,(i[0],i[1]),i[2],(165,25,165),2)
(C#)
var houghCircles = Cv2.HoughCircles(morphed, HoughMethods.Gradient, 1, max, cannyEdgeThreshold, houghThreshold, min, max);
foreach (var circle in houghCircles)
Cv2.Circle(morphed, circle.Center, (int)circle.Radius, new Scalar(165, 25, 165), 2);
:
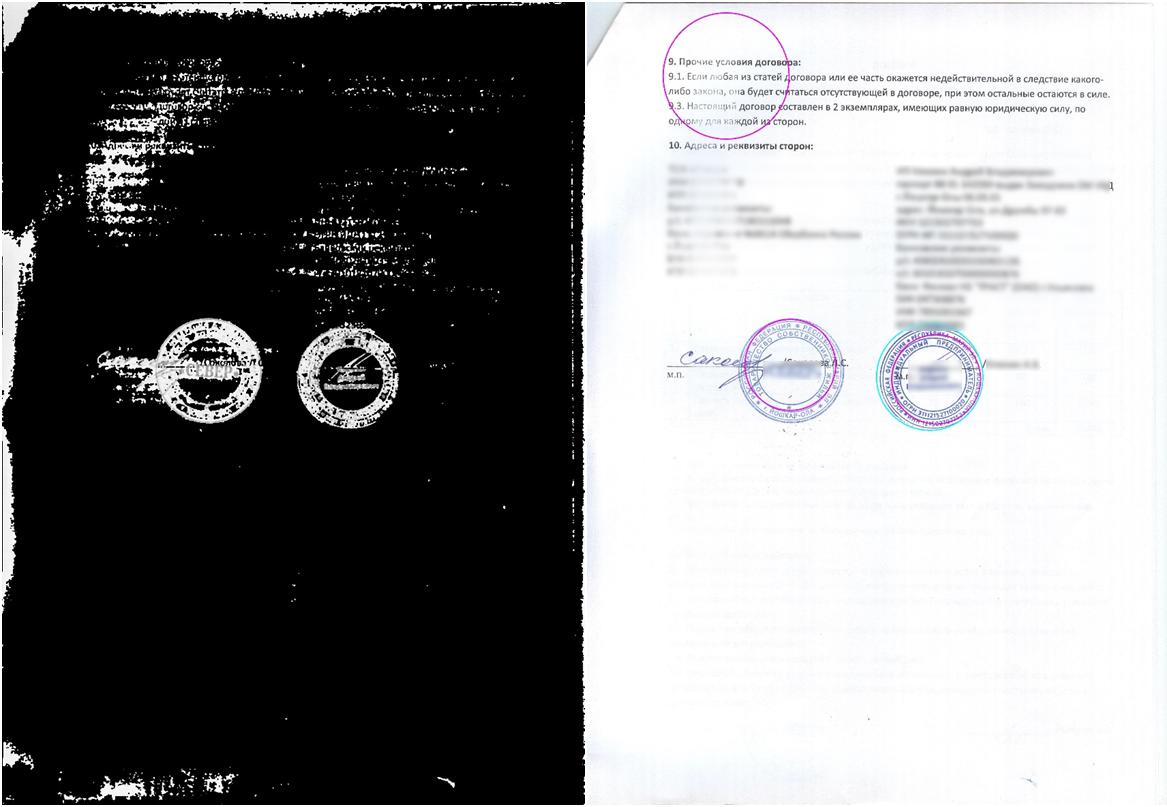
, , . , , . , , , . (- ββ - , - ).
- . .
, , . ββ , , . β , .
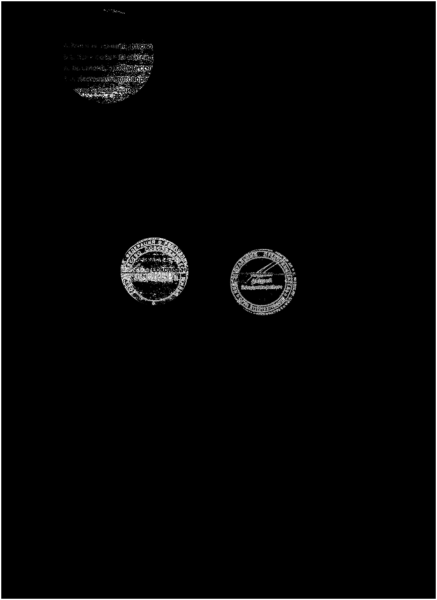
, , , . . , . ββ .
(Python)def equals(first, second, epsilon):
diff = cv2.subtract(first, second)
nonZero = cv2.countNonZero(diff)
area = first.size * epsilon
return nonZero <= area
for i in circles[0, :]:
empty = np.zeros((256, 256, 1), dtype="uint8")
cv2.circle(empty, (i[0], i[1]), i[2], (255, 255, 255), -1)
crop = img * (empty.astype(img.dtype))
cv2.imwrite('crop.bmp', crop)
if not equals(crop, empty, threshold):
result.append(i)
(C#)foreach (var circle in circles)
{
var x = (int) circle.Center.X;
var y = (int) circle.Center.Y;
var radius = (int) Math.Floor(circle.Radius);
using (var empty = Mat.Zeros(src.Rows, src.Cols, MatType.CV_8UC1))
using (var mask = empty.ToMat())
using (var crop = new Mat())
{
Cv2.Circle(mask, x, y, radius, Scalar.White, -1);
src.CopyTo(crop, mask);
crop.Save("crop.bmp");
if (!MatEquals(crop, empty, threshold))
result.Add(circle);
}
}
private bool MatEquals(Mat a, Mat b, double epsilon = 0.0)
{
var notEquals = a.NotEquals(b);
var nonZero = Cv2.CountNonZero(notEquals);
var area = a.Rows * epsilon * a.Cols;
return nonZero <= area;
}
. , , , - ?
, β , β (minor) (major) ( , , ).
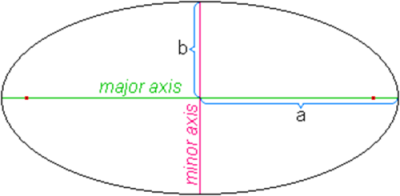
: , , , .
() , , voila β .
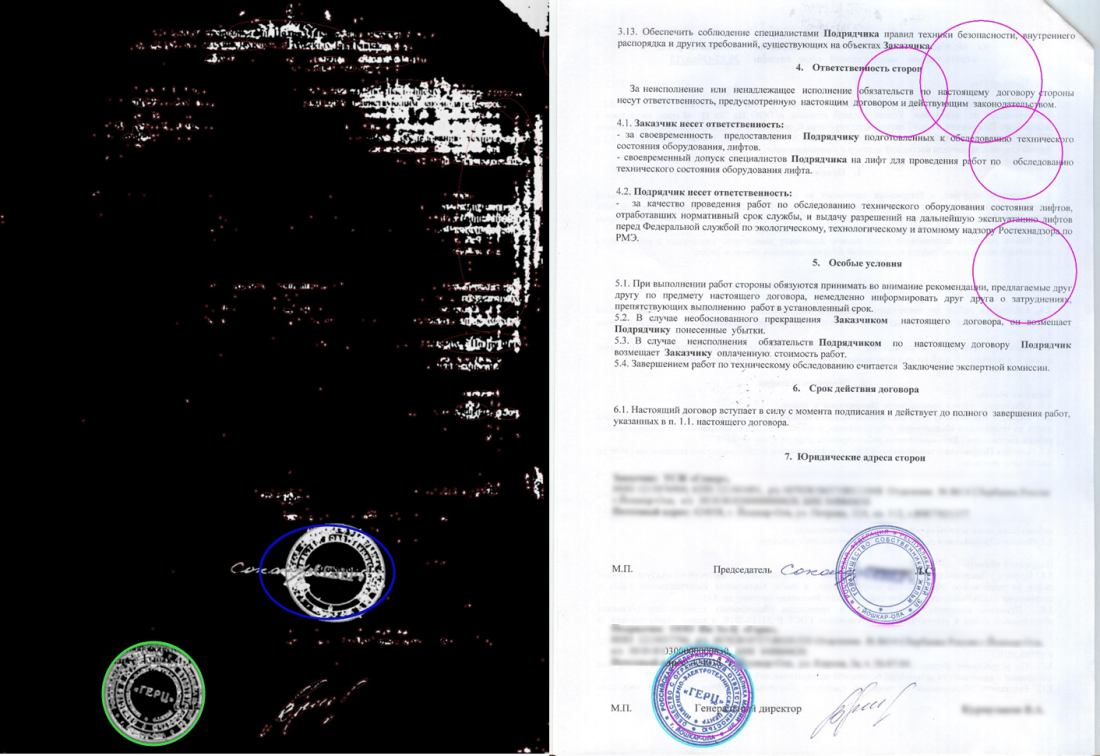
β , β , β , β , .
(Python)segments = []
contours, hierarchy = cv2.findContours(img, cv2.RETR_EXTERNAL, cv2.CHAIN_APPROX_SIMPLE)
for contour in contours:
if contour.shape[0] < 5:
continue
ellipse = cv2.fitEllipse(contour)
width = ellipse[1][0]
height = ellipse[1][1]
minor = min(width, height)
major = max(width, height)
if minor / 2 > minorMin and major / 2 < majorMax:
r1 = math.fabs(1 - math.fabs(major - minor) / max(minor, major))
cv2.ellipse(src, ellipse, (255, 0, 0), 3)
if r1 > roundness:
segments.append((ellipse[0], major / 2))
cv2.ellipse(src, ellipse, (0, 255, 0), 3)
else: cv2.ellipse(src, ellipse, (0, 0, 255), 1)
cv2.imwrite('test_res.bmp', src)
(Python)def distance(p1, p2):
return math.sqrt(math.pow(p2[0] - p1[0], 2) + math.pow(p2[1] - p1[1], 2))
def isNested(inner, outer, epsilon):
distance = distance(inner[0], outer[0])
radius = outer[1] * epsilon
return distance < radius and inner[1] < radius - distance
nested = []
for i in inner:
for o in outer:
if (isNested(i, o, 1.3)):
if (distance(i[0], i[1]) < 30 and i[1] / o[1] > 0.75):
nested.append(i)
else: nested.append(o)
(C#)Cv2.FindContours(src, out var contours, hierarchy, RetrievalModes.External, ContourApproximationModes.ApproxSimple);
foreach (var contour in contours)
{
if (contour.Height < 5)
continue;
var ellipse = Cv2.FitEllipse(contour);
var minor = Math.Min(ellipse.Size.Width, ellipse.Size.Height);
var major = Math.Max(ellipse.Size.Width, ellipse.Size.Height);
if (minor / 2 > minorSize && major / 2 < majorSize)
{
var r1 = Math.Abs(1 - Math.Abs(major - minor) / Math.Max(minor, major));
if (r1 > roundness)
{
var circle = new CircleSegment(ellipse.Center, major / 2);
segments.Add(circle);
}
}
contour.Dispose();
}
}
(C#)private bool IsCirclesNested(CircleSegment inner, CircleSegment outer, double epsilon)
{
var distance = inner.Center.DistanceTo(outer.Center);
var secondRadius = outer.Radius * epsilon;
return distance < secondRadius && inner.Radius < secondRadius - distance;
}
var nested = new List<CircleSegment>();
foreach (var i in inner)
foreach (var o in outer)
{
if (IsCirclesNested(i, o, 1.3))
{
if (i.Center.DistanceTo(o.Center) < 30 &&
i.Radius / o.Radius > 0.75)
nested.Add(i);
else nested.Add(o);
}
}
return outer.Union(inner).Except(nested).ToList();
, , , . . β , .
β β, , - . β β? ββ . ? , - . :
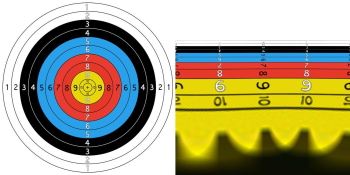
, , : , , . :
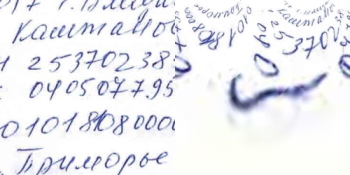
β X Y , X, . , , , .
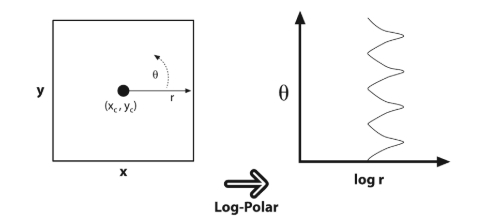
β , , ( ). , , ( , , , ).
, , . ( ) 90 .
(Python)maxRadius = math.sqrt(math.pow(src.shape[0], 2) + math.pow(src.shape[1], 2)) / 2
magnitude = src.shape[0] / math.log(maxRadius)
center = (src.shape[0] / 2, src.shape[1] / 2)
polar = cv2.logPolar(src, center, magnitude, cv2.INTER_AREA)
(C#)var maxRadius = Math.Sqrt(Math.Pow(stampImage.Width, 2) + Math.Pow(stampImage.Height, 2)) / 2;
var magnitude = stampImage.Width / Math.Log(maxRadius);
var center = new Point2f(stampImage.Width / 2, stampImage.Height / 2);
Cv2.LogPolar(stampImage, cartesianImage, center, magnitude, InterpolationFlags.Area);
, , OpenCVβ . , , , , OpenCV. , .
:
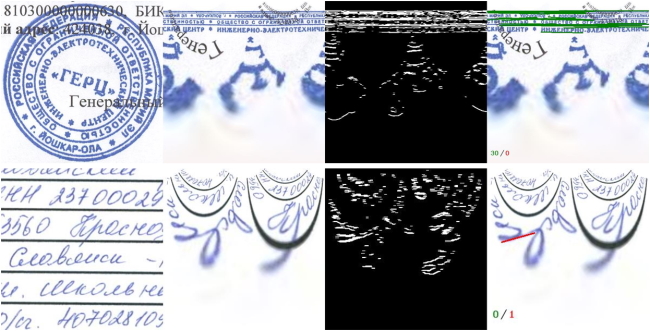
, , 90 , .
(Python)
sobel = cv2.Sobel(polar, cv2.CV_16S, 1, 0)
kernel = cv2.getStructuringElement(shape=cv2.MORPH_RECT, ksize=(1, 5))
img = cv2.convertScaleAbs(sobel)
img = cv2.cvtColor(img, cv2.COLOR_BGR2GRAY)
img = cv2.threshold(img, 0, 255, cv2.THRESH_BINARY + cv2.THRESH_OTSU)[1]
img = cv2.morphologyEx(img, cv2.MORPH_OPEN, kernel)
img = cv2.morphologyEx(img, cv2.MORPH_CLOSE, kernel)
def is_vertical(img_src, line):
tolerance = 10
coords = line[0]
angle = math.atan2(coords[3] - coords[1], coords[2] - coords[0]) * 180.0 / math.pi
edge = img_src.shape[0] * 0.66
out_of_bounds = coords[0] < edge and coords[2] < edge
return math.fabs(90 - math.fabs(angle)) <= tolerance and not out_of_bounds
lines = cv2.HoughLinesP(img, 1, math.pi / 180, 15, img.shape[0] / 5, 10)
vertical = [line for line in lines if is_vertical(img, line)]
correct_lines = len(vertical)
(C#)
using (var sobel = new Mat())
using (var kernel = Cv2.GetStructuringElement(MorphShapes.Rect, new Size(1, 5)))
{
Cv2.Sobel(img, sobel, MatType.CV_16S, 1, 0);
Cv2.ConvertScaleAbs(sobel, img);
Cv2.Threshold(img, img, 0, 255, ThresholdTypes.Binary | ThresholdTypes.Otsu);
Cv2.MorphologyEx(img, img, MorphTypes.Open, kernel);
Cv2.MorphologyEx(img, img, MorphTypes.Close, kernel);
}
bool AlmostVerticalLine(LineSegmentPoint line)
{
const int tolerance = 10;
var angle = Math.Atan2(line.P2.Y - line.P1.Y, line.P2.X - line.P1.X) * 180.0 / Math.PI;
var edge = edges.Width * 0.66;
var outOfBounds = line.P1.X < edge && line.P2.X < edge;
return Math.Abs(90 - Math.Abs(angle)) <= tolerance && !outOfBounds;
}
var lines = Cv2.HoughLinesP(img, 1, Math.PI / 180, 15, img.Width / 5, 10);
var correctLinesCount = lines.Count(AlmostVerticalLine);
, 85%. :
, β β ?β. :
, , , . OpenCV , . U-Net, OpenCV , .