рдирдЬрд╝рд░ рд░рдЦрдиреЗ рдХрд╛ рдмрд╛рдЬрд╛рд░ рдмрдврд╝рдиреЗ рдФрд░ рдмрдврд╝рдиреЗ рдХреА рдЙрдореНрдореАрдж рд╣реИ: 2020 рдореЗрдВ $ 560 рдорд┐рд▓рд┐рдпрди рд╕реЗ 2025 рдореЗрдВ $ 1,786 рдмрд┐рд▓рд┐рдпрди ред рддреЛ рдЕрдкреЗрдХреНрд╖рд╛рдХреГрдд рдорд╣рдВрдЧреЗ рдЙрдкрдХрд░рдгреЛрдВ рдХрд╛ рд╡рд┐рдХрд▓реНрдк рдХреНрдпрд╛ рд╣реИ? рдмреЗрд╢рдХ, рдПрдХ рд╕рд╛рдзрд╛рд░рдг рд╡реЗрдм рдХреИрдорд░рд╛! рджреВрд╕рд░реЛрдВ рдХреА рддрд░рд╣, рдпрд╣ рджреГрд╖реНрдЯрд┐рдХреЛрдг рдХрдИ рдХрдард┐рдирд╛рдЗрдпреЛрдВ рдХрд╛ рд╕рд╛рдордирд╛ рдХрд░рддрд╛ рд╣реИ, рдЪрд╛рд╣реЗ рд╡рд╣ рд╣реЛ: рдЙрдкрдХрд░рдгреЛрдВ рдХреА рдПрдХ рд╡рд┐рд╕реНрддреГрдд рд╡рд┐рд╡рд┐рдзрддрд╛ (рдЗрд╕рд▓рд┐рдП, рдРрд╕реА рд╕реЗрдЯрд┐рдВрдЧреНрд╕ рдЪреБрдирдирд╛ рдореБрд╢реНрдХрд┐рд▓ рд╣реИ рдЬреЛ рд╕рднреА рдХреИрдорд░реЛрдВ рдкрд░ рд╕рдорд╛рди рд░реВрдк рд╕реЗ рдХрд╛рдо рдХрд░реЗрдВрдЧреЗ), рдорд╛рдкрджрдВрдбреЛрдВ рдХреА рдордЬрдмреВрдд рдкрд░рд┐рд╡рд░реНрддрдирд╢реАрд▓рддрд╛ (рдкреНрд░рдХрд╛рд╢ рд╕реЗ рдХреИрдорд░рд╛ рдЭреБрдХрд╛рд╡ рдФрд░ рдЪреЗрд╣рд░реЗ рдХреЗ рд╕рд╛рдкреЗрдХреНрд╖ рдЗрд╕рдХреА рд╕реНрдерд┐рддрд┐, рд╕рднреНрдп рдХрдВрдкреНрдпреВрдЯрд┐рдВрдЧ) рд╢рдХреНрддрд┐ (рдХрдИ рдХреЛрдбрд╛-рдХреЛрд░ рдФрд░ рдПрдХреНрд╕реЛрди - рдпрд╣ рдмрд╛рдд рд╣реИ ...)
рд╣рд╛рд▓рд╛рдВрдХрд┐ рдПрдХ рдорд┐рдирдЯ рдкреНрд░рддреАрдХреНрд╖рд╛ рдХрд░реЗрдВ, рдХреНрдпрд╛ рд╡рд╛рд╕реНрддрд╡ рдореЗрдВ рдЯреЙрдк-рдПрдВрдб рд╣рд╛рд░реНрдбрд╡реЗрдпрд░ рдкрд░ рдкреИрд╕рд╛ рдЦрд░реНрдЪ рдХрд░рдирд╛ рдФрд░ рдпрд╣рд╛рдВ рддрдХ тАЛтАЛрдХрд┐ рд╡реАрдбрд┐рдпреЛ рдХрд╛рд░реНрдб рдЦрд░реАрджрдирд╛ рднреА рдЖрд╡рд╢реНрдпрдХ рд╣реИ? рд╢рд╛рдпрдж рд╕реАрдкреАрдпреВ рдкрд░ рд╕рднреА рдЧрдгрдирд╛рдУрдВ рдХреЛ рдлрд┐рдЯ рдХрд░рдиреЗ рдХрд╛ рдПрдХ рддрд░реАрдХрд╛ рд╣реИ рдФрд░ рдЧрддрд┐ рдореЗрдВ рдПрдХ рд╣реА рд╕рдордп рдореЗрдВ рдирд╣реАрдВ рдЦреЛрдирд╛ рд╣реИ?
(рдареАрдХ рд╣реИ, рдЕрдЧрд░ рдЗрд╕ рддрд░рд╣ рд╕реЗ рдирд╣реАрдВ рдерд╛, рддреЛ PyTch рдкрд░ рдПрдХ рдиреНрдпреВрд░реЙрди рдХреЛ рдкреНрд░рд╢рд┐рдХреНрд╖рд┐рдд рдХрд░рдиреЗ рдХреЗ рддрд░реАрдХреЗ рдкрд░ рдПрдХ рд▓реЗрдЦ рдирд╣реАрдВ рд╣реЛрдЧрд╛)
рдбреЗрдЯрд╛
рдбреЗрдЯрд╛ рд╡рд┐рдЬреНрдЮрд╛рди рдореЗрдВ рд╣рдореЗрд╢рд╛ рдХреА рддрд░рд╣, рд╕рдмрд╕реЗ рдорд╣рддреНрд╡рдкреВрд░реНрдг рд╕рд╡рд╛рд▓ред рдЦреЛрдЬ рдХреЗ рдХреБрдЫ рд╕рдордп рдмрд╛рдж, рдореБрдЭреЗ MPIIGaze рдбреЗрдЯрд╛рд╕реЗрдЯ рдорд┐рд▓рд╛ ред рд▓реЗрдЦ рдХреЗ рд▓реЗрдЦрдХреЛрдВ рдиреЗ рдЗрд╕реЗ рд╕рдВрд╕рд╛рдзрд┐рдд рдХрд░рдиреЗ рдХреЗ рд▓рд┐рдП рдмрд╣реБрдд рд╕рд╛рд░реЗ рд╢рд╛рдВрдд рддрд░реАрдХреЗ рд╕реБрдЭрд╛рдП (рдЙрджрд╛рд╣рд░рдг рдХреЗ рд▓рд┐рдП, рд╕рд┐рд░ рдХреА рд╕реНрдерд┐рддрд┐ рдХреЛ рд╕рд╛рдорд╛рдиреНрдп рдХрд░рдирд╛), рд▓реЗрдХрд┐рди рд╣рдо рд╕рд░рд▓ рддрд░реАрдХреЗ рд╕реЗ рдЬрд╛рдПрдВрдЧреЗред
рдЗрд╕рд▓рд┐рдП, рдХреЛрд▓рд╛рдм рд▓реЙрдиреНрдЪ рдХрд░реЗрдВ , рд▓реИрдкрдЯреЙрдк рд▓реЛрдб рдХрд░реЗрдВ рдФрд░ рд╢реБрд░реВ рдХрд░реЗрдВ:
import os
import numpy as np
import pandas as pd
import scipy
import scipy.io
from PIL import Image
import cv2
import seaborn as sns
import matplotlib
import matplotlib.pyplot as plt
рдХреЛрд▓рд╛рдм рдореЗрдВ, рдЖрдк рд╕реАрдзреЗ рдЕрдкрдиреЗ рд▓реИрдкрдЯреЙрдк рд╕реЗ тАЛтАЛрд╕рд┐рд╕реНрдЯрдо рдЙрдкрдпреЛрдЧрд┐рддрд╛рдУрдВ рдХрд╛ рдЙрдкрдпреЛрдЧ рдХрд░ рд╕рдХрддреЗ рд╣реИрдВ, рдбреЗрдЯрд╛ рдбрд╛рдЙрдирд▓реЛрдб рдХрд░ рд╕рдХрддреЗ рд╣реИрдВ рдФрд░ рдбреЗрдЯрд╛рд╕реЗрдЯ рдЕрдирдкреИрдХ рдХрд░ рд╕рдХрддреЗ рд╣реИрдВ:
!wget https://datasets.d2.mpi-inf.mpg.de/MPIIGaze/MPIIGaze.tar.gz
!tar xvzf MPIIGaze.tar.gz MPIIGaze
Data/Original . 15, . Annotation Subset , тАФ . header', , .
database_path = "/content/MPIIGaze"
def load_image_data(patient_name):
global database_path
annotation_path = os.path.join(database_path, "Annotation Subset", patient_name + ".txt")
data_folder = os.path.join(database_path, "Data", "Original", patient_name)
annotation = pd.read_csv(annotation_path, sep=" ", header=None)
points = np.array(annotation.loc[:, list(range(1, 17))])
filenames = np.array(annotation.loc[:, [0]]).reshape(-1)
images = [np.array(Image.open(os.path.join(data_folder, filename))) for filename in filenames]
return images, points
images, points = load_image_data("p00")
plt.imshow(images[0])
colors = ["r", "g", "b", "magenta", "y", "cyan", "brown", "lightcoral"]
for i in range(0, len(points[0]), 2):
x, y = points[0, i:i+2]
plt.scatter([x], [y], c=colors[i//2])
:
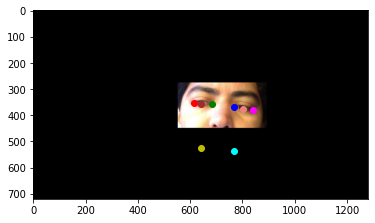
, : , , .
, . , : , (, , ), , 2:1. 2 1 , .
def distance(x1, y1, x2, y2):
return int(((x1 - x2) ** 2 + (y1 - y2) ** 2) ** 0.5)
image_shape = (16, 32)
def handle_eye(image, p1, p2, pupil):
global image_shape
line_len = distance(*p1, *p2)
p1 = p1[::-1]
p2 = p2[::-1]
pupil = pupil[::-1]
corner1 = p1 - np.array([line_len//4, 0])
corner2 = p2 + np.array([line_len//4, 0])
sub_image = image[corner1[0]:corner2[0]+1, corner1[1]:corner2[1]+1]
pupil_new = pupil - corner1
pupil_new = pupil_new / sub_image.shape[:2]
sub_image = cv2.resize(sub_image, image_shape[::-1], interpolation=cv2.INTER_AREA)
sub_image = cv2.cvtColor(sub_image, cv2.COLOR_RGB2GRAY)
return sub_image, pupil_new
2 , тАФ :
def image_to_train_data(image, points):
eye_right_p1 = points[0:2]
eye_right_p2 = points[2:4]
eye_right_pupil = points[12:14]
right_image, right_pupil = handle_eye(image, eye_right_p1, eye_right_p2, eye_right_pupil)
eye_left_p1 = points[4:6]
eye_left_p2 = points[6:8]
eye_left_pupil = points[14:16]
left_image, left_pupil = handle_eye(image, eye_left_p1, eye_left_p2, eye_left_pupil)
return right_image, right_pupil, left_image, left_pupil
( ):
right_image, right_pupil, left_image, left_pupil = image_to_train_data(images[10], points[10])
plt.imshow(right_image, cmap="gray")
r_p_x = int(right_pupil[1] * image_shape[1])
r_p_y = int(right_pupil[0] * image_shape[0])
plt.scatter([r_p_x], [r_p_y], c="red")

, - . :
images_left_conc = []
images_right_conc = []
pupils_left_conc = []
pupils_right_conc = []
patients_path = os.path.join(database_path, "Data", "Original")
for patient in os.listdir(patients_path):
print(patient)
images, points = load_image_data(patient)
for i in range(len(images)):
signle_image_data = image_to_train_data(images[i], points[i])
if any(stuff is None for stuff in signle_image_data):
continue
right_image, right_pupil, left_image, left_pupil = signle_image_data
if any(right_pupil < 0) or any(left_pupil < 0):
continue
images_right_conc.append(right_image)
images_left_conc.append(left_image)
pupils_right_conc.append(right_pupil)
pupils_left_conc.append(left_pupil)
images_left_conc = np.array(images_left_conc)
images_right_conc = np.array(images_right_conc)
pupils_left_conc = np.array(pupils_left_conc)
pupils_right_conc = np.array(pupils_right_conc)
:
images_left_conc = images_left_conc / 255
images_right_conc = images_right_conc / 255
, : :
pupils_conc = np.zeros_like(pupils_left_conc)
for i in range(2):
pupils_conc[:, i] = (pupils_left_conc[:, i] + pupils_right_conc[:, i]) / 2
:
viz_pupils = np.zeros(image_shape)
for y, x in pupils_conc:
y = int(y * image_shape[0])
x = int(x * image_shape[1])
viz_pupils[y, x] += 1
max_val = viz_pupils.max()
viz_pupils = viz_pupils / max_val
plt.imshow(viz_pupils, cmap="hot")
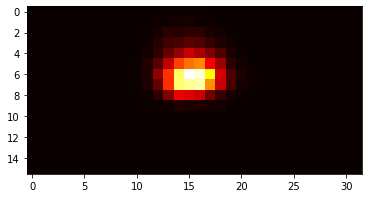
, .
from sklearn.model_selection import train_test_split
import torch
from torch.utils.data import DataLoader, TensorDataset
def make_2eyes_datasets(images_left, images_right, pupils, train_size=0.8):
n, height, width = images_left.shape
images_left = images_left.reshape(n, 1, height, width)
images_right = images_right.reshape(n, 1, height, width)
images_left_train, images_left_val, images_right_train, images_right_val, pupils_train, pupils_val = train_test_split(
images_left, images_right, pupils, train_size=train_size
)
def make_dataset(im_left, im_right, pups):
return TensorDataset(
torch.from_numpy(im_left.astype(np.float32)), torch.from_numpy(im_right.astype(np.float32)), torch.from_numpy(pups.astype(np.float32))
)
train_dataset = make_dataset(images_left_train, images_right_train, pupils_train)
val_dataset = make_dataset(images_left_val, images_right_val, pupils_val)
return train_dataset, val_dataset
def make_dataloaders(train_dataset, val_dataset, batch_size=256):
train_dataloader = DataLoader(train_dataset, batch_size=batch_size)
val_dataloader = DataLoader(val_dataset, batch_size=batch_size)
return train_dataloader, val_dataloader
batch_size = 256
eyes_datasets = make_2eyes_datasets(images_left_conc, images_right_conc, pupils_conc)
eyes_train_loader, eyes_val_loader = make_dataloaders(*eyes_datasets, batch_size=batch_size)
import torch
import torch.nn as nn
import torch.nn.functional as F
class Reshaper(nn.Module):
def __init__(self, target_shape):
super(Reshaper, self).__init__()
self.target_shape = target_shape
def forward(self, input):
return torch.reshape(input, (-1, *self.target_shape))
class EyesNet(nn.Module):
def __init__(self):
super(EyesNet, self).__init__()
self.features_left = nn.Sequential(
nn.Conv2d(in_channels=1, out_channels=32, kernel_size=5, stride=2, padding=2),
nn.LeakyReLU(),
nn.Conv2d(in_channels=32, out_channels=64, kernel_size=3, stride=2, padding=1),
nn.LeakyReLU(),
nn.Conv2d(in_channels=64, out_channels=64, kernel_size=3, stride=2, padding=1),
nn.LeakyReLU(),
nn.Conv2d(in_channels=64, out_channels=64, kernel_size=3, stride=2, padding=1),
nn.LeakyReLU(),
nn.Conv2d(in_channels=64, out_channels=64, kernel_size=3, stride=2, padding=1),
nn.LeakyReLU(),
Reshaper([64])
)
self.features_right = nn.Sequential(
nn.Conv2d(in_channels=1, out_channels=32, kernel_size=5, stride=2, padding=2),
nn.LeakyReLU(),
nn.Conv2d(in_channels=32, out_channels=64, kernel_size=3, stride=2, padding=1),
nn.LeakyReLU(),
nn.Conv2d(in_channels=64, out_channels=64, kernel_size=3, stride=2, padding=1),
nn.LeakyReLU(),
nn.Conv2d(in_channels=64, out_channels=64, kernel_size=3, stride=2, padding=1),
nn.LeakyReLU(),
nn.Conv2d(in_channels=64, out_channels=64, kernel_size=3, stride=2, padding=1),
nn.LeakyReLU(),
Reshaper([64])
)
self.fc = nn.Sequential(
nn.Linear(128, 64),
nn.LeakyReLU(),
nn.Linear(64, 16),
nn.LeakyReLU(),
nn.Linear(16, 2),
nn.Sigmoid()
)
def forward(self, x_left, x_right):
x_left = self.features_left(x_left)
x_right = self.features_right(x_right)
x = torch.cat((x_left, x_right), 1)
x = self.fc(x)
return x
, GPU ( CPU тЙИ ), 8 .
def train(model, train_loader, test_loader, epochs, lr, folder="gazenet"):
os.makedirs(folder, exist_ok=True)
optimizer = torch.optim.Adam(model.parameters(), lr=lr)
mse = nn.MSELoss()
for epoch in range(epochs):
running_loss = 0
error_mean = []
error_std = []
for i, (*xs_batch, y_batch) in enumerate(train_loader):
xs_batch = [x_batch.cuda() for x_batch in xs_batch]
y_batch = y_batch.cuda()
optimizer.zero_grad()
y_batch_pred = model(*xs_batch)
loss = mse(y_batch_pred, y_batch)
loss.backward()
optimizer.step()
running_loss += loss.item()
difference = (y_batch - y_batch_pred).detach().cpu().numpy().reshape(-1)
error_mean.append(np.mean(difference))
error_std.append(np.std(difference))
error_mean = np.mean(error_mean)
error_std = np.mean(error_std)
print(f"Epoch {epoch+1}/{epochs}, train loss: {running_loss}, error mean: {error_mean}, error std: {error_std}")
running_loss = 0
error_mean = []
error_std = []
for i, (*xs_batch, y_batch) in enumerate(train_loader):
xs_batch = [x_batch.cuda() for x_batch in xs_batch]
y_batch = y_batch.cuda()
y_batch_pred = model(*xs_batch)
loss = mse(y_batch_pred, y_batch)
loss.backward()
running_loss += loss.item()
difference = (y_batch - y_batch_pred).detach().cpu().numpy().reshape(-1)
error_mean.append(np.mean(difference))
error_std.append(np.std(difference))
error_mean = np.mean(error_mean)
error_std = np.mean(error_std)
print(f"Epoch {epoch+1}/{epochs}, val loss: {running_loss}, error mean: {error_mean}, error std: {error_std}")
epoch_path = os.path.join(folder, f"epoch_{epoch+1}.pth")
torch.save(model.state_dict(), epoch_path)
eyesnet = EyesNet().cuda()
train(eyesnet, eyes_train_loader, eyes_val_loader, 300, 1e-3, "eyes_net")
, 300 ( , ):
Epoch 1/300, train loss: 0.3125856015831232, error mean: -0.019309822469949722, error std: 0.08668763190507889
Epoch 1/300, val loss: 0.18365296721458435, error mean: -0.008721884340047836, error std: 0.07283741235733032
Epoch 2/300, train loss: 0.1700970521196723, error mean: 0.0001489206333644688, error std: 0.07033108174800873
Epoch 2/300, val loss: 0.1475073655601591, error mean: -0.001808341359719634, error std: 0.06572529673576355
...
Epoch 299/300, train loss: 0.003378463063199888, error mean: -8.133996743708849e-05, error std: 0.009488753043115139
Epoch 299/300, val loss: 0.004163481352406961, error mean: -0.001996406354010105, error std: 0.010547727346420288
Epoch 300/300, train loss: 0.003569353237253381, error mean: -9.1125002654735e-05, error std: 0.00977678969502449
Epoch 300/300, val loss: 0.004456713928448153, error mean: 0.0008482271223329008, error std: 0.010923181660473347
299 , .
:
import random
def show_output(model, data_loader, batch_num=0, samples=5, grid_shape=(5, 1), figsize=(10, 10)):
for i, (*xs, y) in enumerate(data_loader):
if i == batch_num:
break
xs = [x.cuda() for x in xs]
y_pred = model(*xs).detach().cpu().numpy().reshape(-1, 2)
xs = [x.detach().cpu().numpy().reshape(-1, 16, 32) for x in xs]
imgs_conc = np.hstack(xs)
y = y.cpu().numpy().reshape(-1, 2)
indices = random.sample(range(len(y_pred)), samples)
fig, axes = plt.subplots(*grid_shape, figsize=figsize)
for i, index in enumerate(indices):
row = i // grid_shape[1]
column = i % grid_shape[1]
axes[row, column].imshow(imgs_conc[index])
axes[row, column].scatter([y_pred[index, 1]*32, y_pred[index, 1]*32], [y_pred[index, 0]*16, (y_pred[index, 0]+1)*16], c="r")
axes[row, column].scatter([y[index, 1]*32, y[index, 1]*32], [y[index, 0]*16, (y[index, 0]+1)*16], c="g")
eyesnet.load_state_dict(torch.load("eyes_net/epoch_299.pth"))
show_output(eyesnet, eyes_val_loader, 103, 16, (4, 4))
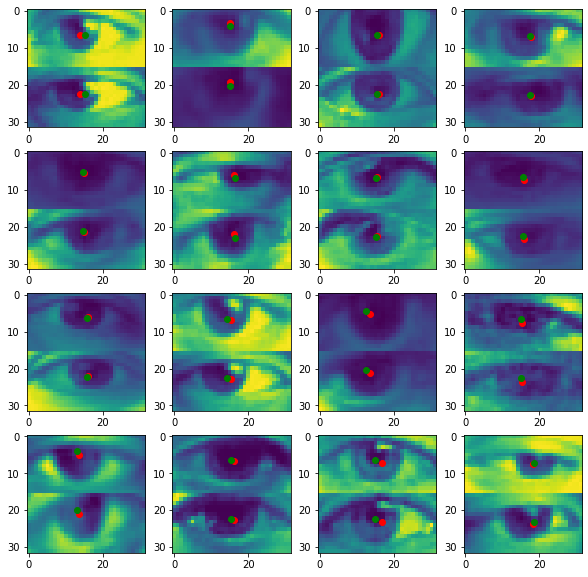
, , "" , . тАФ -, - , -, . , .
( X Y), :
def error_distribution(model, data_loader, image_shape=(16, 32), bins=32, digits=2, figsize=(10,10)):
ys_true = []
ys_pred = []
for *xs, y in data_loader:
xs = [x.cuda() for x in xs]
y_pred = model(*xs)
ys_true.append(y.detach().cpu().numpy())
ys_pred.append(y_pred.detach().cpu().numpy())
ys_true = np.concatenate(ys_true)
ys_pred = np.concatenate(ys_pred)
indices = np.arange(len(ys_true))
fig, axes = plt.subplots(2, figsize=figsize)
for ax_num in range(2):
ys_true_subset = ys_true[:, ax_num]
ys_pred_subset = ys_pred[:, ax_num]
counts, ranges = np.histogram(ys_true_subset, bins=bins)
errors = []
labels = []
for i in range(len(counts)):
begin, end = ranges[i], ranges[i + 1]
range_indices = indices[(ys_true_subset >= begin) & (ys_true_subset <= end)]
diffs = np.abs(ys_pred_subset[range_indices] - ys_true_subset[range_indices])
label = (begin + end) / 2
if image_shape:
diffs = diffs * image_shape[ax_num]
label = label * image_shape[ax_num]
else:
label = round(label, digits)
errors.append(diffs)
labels.append(str(label)[:2+digits])
axes[ax_num].boxplot(errors, labels=labels)
if image_shape:
y_label = "difference, px"
x_label = "true position, px"
else:
y_label = "difference"
x_label = "true position"
axes[ax_num].set_ylabel(y_label)
axes[ax_num].set_xlabel(x_label)
if ax_num == 0:
title = "Y"
else:
title = "X"
axes[ax_num].set_title(title)
error_distribution(eyesnet, eyes_val_loader, figsize=(20, 10))

,
-, . , . :
import time
def measure_time(model, data_loader, n_batches=5):
begin_time = time.time()
batch_num = 0
n_samples = 0
predicted = []
for *xs, y in data_loader:
xs = [x.cpu() for x in xs]
y_pred = model(*xs)
predicted.append(y_pred.detach().cpu().numpy().reshape(-1))
batch_num += 1
n_samples += len(y)
if batch_num >= n_batches:
break
end_time = time.time()
time_per_sample = (end_time - begin_time) / n_samples
return time_per_sample
eyesnet_cpu = EyesNet().cpu()
eyesnet_cpu.load_state_dict(torch.load("eyes_net/epoch_299.pth", map_location="cpu"))
_, eyes_val_loader_single = make_dataloaders(*eyes_datasets, batch_size=1)
tps = measure_time(eyesnet_cpu, eyes_val_loader_single)
print(f"{tps} seconds per sample")
>>> 0.003347921371459961 seconds per sample
, VGG16 ( , ):
import torchvision.models as models
class VGG16Based(nn.Module):
def __init__(self):
super(VGG16Based, self).__init__()
self.vgg = models.vgg16(pretrained=False)
self.vgg.classifier = nn.Sequential(
nn.Linear(25088, 256),
nn.LeakyReLU(),
nn.Linear(256, 2),
nn.Sigmoid()
)
def forward(self, x_left, x_right):
x_mid = (x_left + x_right) / 2
x = torch.cat((x_left, x_mid, x_right), dim=1)
x_pad = torch.zeros((x.shape[0], 3, 32, 32))
x_pad[:, :, :16, :] = x
x = self.vgg(x_pad)
return x
vgg16 = VGG16Based()
vgg16_tps = measure_time(vgg16, eyes_val_loader_single)
print(f"{vgg16_tps} seconds per sample")
>>> 0.023713159561157226 seconds per sample
, (AMD A10-4600M APU, 1500 MHz):
python benchmark.py
0.003980588912963867 seconds per sample, EyesNet
0.12246298789978027 seconds per sample, VGG16-based
, , , ( VGG16 80 , EyesNet тАФ 1 ; , , ). , , . , :
- ( ).
- . , float8 float32 ( , , ).
- PyTorch Mobile тАФ PyTorch . .
- . тАФ GazeCapture. , , тАФ :
- TFLite тАФ TensorFlow . !
, . Data science ( тАФ *^*) . тАФ FARADAY Lab. тАФ , .
c:
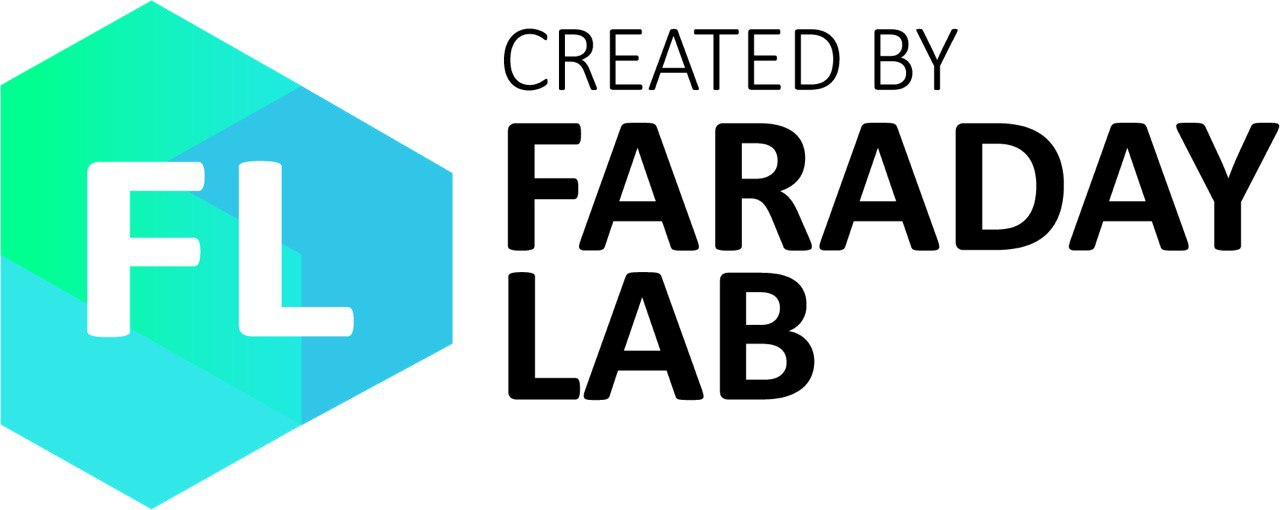
: