Le marché de l'eye-tracking devrait croître et croître: de 560 millions de dollars en 2020 à 1786 milliards de dollars en 2025 . Alors, quelle est l'alternative aux appareils relativement chers? Bien sûr, une simple webcam! Comme d'autres, cette approche rencontre de nombreuses difficultés, que ce soit: une grande variété d'appareils (par conséquent, il est difficile de choisir des paramètres qui fonctionneront également sur toutes les caméras), une forte variabilité des paramètres (de l'éclairage à l'inclinaison de la caméra et sa position par rapport au visage), une informatique décente puissance (plusieurs cuda-cores et Xeon - c'est tout) ...
Bien qu'attendez une minute, est-il vraiment nécessaire de dépenser de l'argent sur du matériel haut de gamme et même d'acheter une carte vidéo? Peut-être qu'il existe un moyen d'adapter tous les calculs sur le processeur et de ne pas perdre en même temps de vitesse?
(Eh bien, s'il n'y avait pas une telle façon, il n'y aurait pas d'article sur la façon d'entraîner un neurone sur PyTorch)
Les données
Comme toujours en science des données, la question la plus importante. Après un certain temps de recherche, j'ai trouvé le jeu de données MPIIGaze . Les auteurs de l' article ont suggéré de nombreuses façons intéressantes de le traiter (par exemple, normaliser la position de la tête), mais nous allons suivre la voie simple.
Alors, lancez Colab , chargez l'ordinateur portable et lancez:
import os
import numpy as np
import pandas as pd
import scipy
import scipy.io
from PIL import Image
import cv2
import seaborn as sns
import matplotlib
import matplotlib.pyplot as plt
Dans Colab, vous pouvez utiliser les utilitaires système directement depuis votre ordinateur portable, semer, télécharger et décompresser l'ensemble de données:
!wget https://datasets.d2.mpi-inf.mpg.de/MPIIGaze/MPIIGaze.tar.gz
!tar xvzf MPIIGaze.tar.gz MPIIGaze
Data/Original . 15, . Annotation Subset , — . header', , .
database_path = "/content/MPIIGaze"
def load_image_data(patient_name):
global database_path
annotation_path = os.path.join(database_path, "Annotation Subset", patient_name + ".txt")
data_folder = os.path.join(database_path, "Data", "Original", patient_name)
annotation = pd.read_csv(annotation_path, sep=" ", header=None)
points = np.array(annotation.loc[:, list(range(1, 17))])
filenames = np.array(annotation.loc[:, [0]]).reshape(-1)
images = [np.array(Image.open(os.path.join(data_folder, filename))) for filename in filenames]
return images, points
images, points = load_image_data("p00")
plt.imshow(images[0])
colors = ["r", "g", "b", "magenta", "y", "cyan", "brown", "lightcoral"]
for i in range(0, len(points[0]), 2):
x, y = points[0, i:i+2]
plt.scatter([x], [y], c=colors[i//2])
:
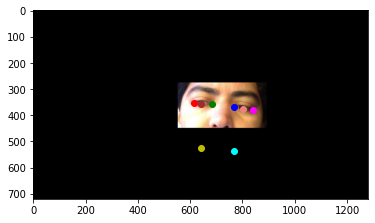
, : , , .
, . , : , (, , ), , 2:1. 2 1 , .
def distance(x1, y1, x2, y2):
return int(((x1 - x2) ** 2 + (y1 - y2) ** 2) ** 0.5)
image_shape = (16, 32)
def handle_eye(image, p1, p2, pupil):
global image_shape
line_len = distance(*p1, *p2)
p1 = p1[::-1]
p2 = p2[::-1]
pupil = pupil[::-1]
corner1 = p1 - np.array([line_len//4, 0])
corner2 = p2 + np.array([line_len//4, 0])
sub_image = image[corner1[0]:corner2[0]+1, corner1[1]:corner2[1]+1]
pupil_new = pupil - corner1
pupil_new = pupil_new / sub_image.shape[:2]
sub_image = cv2.resize(sub_image, image_shape[::-1], interpolation=cv2.INTER_AREA)
sub_image = cv2.cvtColor(sub_image, cv2.COLOR_RGB2GRAY)
return sub_image, pupil_new
2 , — :
def image_to_train_data(image, points):
eye_right_p1 = points[0:2]
eye_right_p2 = points[2:4]
eye_right_pupil = points[12:14]
right_image, right_pupil = handle_eye(image, eye_right_p1, eye_right_p2, eye_right_pupil)
eye_left_p1 = points[4:6]
eye_left_p2 = points[6:8]
eye_left_pupil = points[14:16]
left_image, left_pupil = handle_eye(image, eye_left_p1, eye_left_p2, eye_left_pupil)
return right_image, right_pupil, left_image, left_pupil
( ):
right_image, right_pupil, left_image, left_pupil = image_to_train_data(images[10], points[10])
plt.imshow(right_image, cmap="gray")
r_p_x = int(right_pupil[1] * image_shape[1])
r_p_y = int(right_pupil[0] * image_shape[0])
plt.scatter([r_p_x], [r_p_y], c="red")

, - . :
images_left_conc = []
images_right_conc = []
pupils_left_conc = []
pupils_right_conc = []
patients_path = os.path.join(database_path, "Data", "Original")
for patient in os.listdir(patients_path):
print(patient)
images, points = load_image_data(patient)
for i in range(len(images)):
signle_image_data = image_to_train_data(images[i], points[i])
if any(stuff is None for stuff in signle_image_data):
continue
right_image, right_pupil, left_image, left_pupil = signle_image_data
if any(right_pupil < 0) or any(left_pupil < 0):
continue
images_right_conc.append(right_image)
images_left_conc.append(left_image)
pupils_right_conc.append(right_pupil)
pupils_left_conc.append(left_pupil)
images_left_conc = np.array(images_left_conc)
images_right_conc = np.array(images_right_conc)
pupils_left_conc = np.array(pupils_left_conc)
pupils_right_conc = np.array(pupils_right_conc)
:
images_left_conc = images_left_conc / 255
images_right_conc = images_right_conc / 255
, : :
pupils_conc = np.zeros_like(pupils_left_conc)
for i in range(2):
pupils_conc[:, i] = (pupils_left_conc[:, i] + pupils_right_conc[:, i]) / 2
:
viz_pupils = np.zeros(image_shape)
for y, x in pupils_conc:
y = int(y * image_shape[0])
x = int(x * image_shape[1])
viz_pupils[y, x] += 1
max_val = viz_pupils.max()
viz_pupils = viz_pupils / max_val
plt.imshow(viz_pupils, cmap="hot")
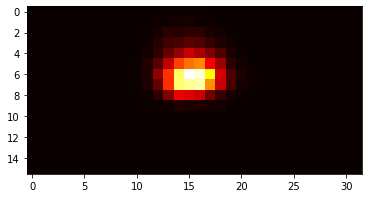
, .
from sklearn.model_selection import train_test_split
import torch
from torch.utils.data import DataLoader, TensorDataset
def make_2eyes_datasets(images_left, images_right, pupils, train_size=0.8):
n, height, width = images_left.shape
images_left = images_left.reshape(n, 1, height, width)
images_right = images_right.reshape(n, 1, height, width)
images_left_train, images_left_val, images_right_train, images_right_val, pupils_train, pupils_val = train_test_split(
images_left, images_right, pupils, train_size=train_size
)
def make_dataset(im_left, im_right, pups):
return TensorDataset(
torch.from_numpy(im_left.astype(np.float32)), torch.from_numpy(im_right.astype(np.float32)), torch.from_numpy(pups.astype(np.float32))
)
train_dataset = make_dataset(images_left_train, images_right_train, pupils_train)
val_dataset = make_dataset(images_left_val, images_right_val, pupils_val)
return train_dataset, val_dataset
def make_dataloaders(train_dataset, val_dataset, batch_size=256):
train_dataloader = DataLoader(train_dataset, batch_size=batch_size)
val_dataloader = DataLoader(val_dataset, batch_size=batch_size)
return train_dataloader, val_dataloader
batch_size = 256
eyes_datasets = make_2eyes_datasets(images_left_conc, images_right_conc, pupils_conc)
eyes_train_loader, eyes_val_loader = make_dataloaders(*eyes_datasets, batch_size=batch_size)
import torch
import torch.nn as nn
import torch.nn.functional as F
class Reshaper(nn.Module):
def __init__(self, target_shape):
super(Reshaper, self).__init__()
self.target_shape = target_shape
def forward(self, input):
return torch.reshape(input, (-1, *self.target_shape))
class EyesNet(nn.Module):
def __init__(self):
super(EyesNet, self).__init__()
self.features_left = nn.Sequential(
nn.Conv2d(in_channels=1, out_channels=32, kernel_size=5, stride=2, padding=2),
nn.LeakyReLU(),
nn.Conv2d(in_channels=32, out_channels=64, kernel_size=3, stride=2, padding=1),
nn.LeakyReLU(),
nn.Conv2d(in_channels=64, out_channels=64, kernel_size=3, stride=2, padding=1),
nn.LeakyReLU(),
nn.Conv2d(in_channels=64, out_channels=64, kernel_size=3, stride=2, padding=1),
nn.LeakyReLU(),
nn.Conv2d(in_channels=64, out_channels=64, kernel_size=3, stride=2, padding=1),
nn.LeakyReLU(),
Reshaper([64])
)
self.features_right = nn.Sequential(
nn.Conv2d(in_channels=1, out_channels=32, kernel_size=5, stride=2, padding=2),
nn.LeakyReLU(),
nn.Conv2d(in_channels=32, out_channels=64, kernel_size=3, stride=2, padding=1),
nn.LeakyReLU(),
nn.Conv2d(in_channels=64, out_channels=64, kernel_size=3, stride=2, padding=1),
nn.LeakyReLU(),
nn.Conv2d(in_channels=64, out_channels=64, kernel_size=3, stride=2, padding=1),
nn.LeakyReLU(),
nn.Conv2d(in_channels=64, out_channels=64, kernel_size=3, stride=2, padding=1),
nn.LeakyReLU(),
Reshaper([64])
)
self.fc = nn.Sequential(
nn.Linear(128, 64),
nn.LeakyReLU(),
nn.Linear(64, 16),
nn.LeakyReLU(),
nn.Linear(16, 2),
nn.Sigmoid()
)
def forward(self, x_left, x_right):
x_left = self.features_left(x_left)
x_right = self.features_right(x_right)
x = torch.cat((x_left, x_right), 1)
x = self.fc(x)
return x
, GPU ( CPU ≈ ), 8 .
def train(model, train_loader, test_loader, epochs, lr, folder="gazenet"):
os.makedirs(folder, exist_ok=True)
optimizer = torch.optim.Adam(model.parameters(), lr=lr)
mse = nn.MSELoss()
for epoch in range(epochs):
running_loss = 0
error_mean = []
error_std = []
for i, (*xs_batch, y_batch) in enumerate(train_loader):
xs_batch = [x_batch.cuda() for x_batch in xs_batch]
y_batch = y_batch.cuda()
optimizer.zero_grad()
y_batch_pred = model(*xs_batch)
loss = mse(y_batch_pred, y_batch)
loss.backward()
optimizer.step()
running_loss += loss.item()
difference = (y_batch - y_batch_pred).detach().cpu().numpy().reshape(-1)
error_mean.append(np.mean(difference))
error_std.append(np.std(difference))
error_mean = np.mean(error_mean)
error_std = np.mean(error_std)
print(f"Epoch {epoch+1}/{epochs}, train loss: {running_loss}, error mean: {error_mean}, error std: {error_std}")
running_loss = 0
error_mean = []
error_std = []
for i, (*xs_batch, y_batch) in enumerate(train_loader):
xs_batch = [x_batch.cuda() for x_batch in xs_batch]
y_batch = y_batch.cuda()
y_batch_pred = model(*xs_batch)
loss = mse(y_batch_pred, y_batch)
loss.backward()
running_loss += loss.item()
difference = (y_batch - y_batch_pred).detach().cpu().numpy().reshape(-1)
error_mean.append(np.mean(difference))
error_std.append(np.std(difference))
error_mean = np.mean(error_mean)
error_std = np.mean(error_std)
print(f"Epoch {epoch+1}/{epochs}, val loss: {running_loss}, error mean: {error_mean}, error std: {error_std}")
epoch_path = os.path.join(folder, f"epoch_{epoch+1}.pth")
torch.save(model.state_dict(), epoch_path)
eyesnet = EyesNet().cuda()
train(eyesnet, eyes_train_loader, eyes_val_loader, 300, 1e-3, "eyes_net")
, 300 ( , ):
Epoch 1/300, train loss: 0.3125856015831232, error mean: -0.019309822469949722, error std: 0.08668763190507889
Epoch 1/300, val loss: 0.18365296721458435, error mean: -0.008721884340047836, error std: 0.07283741235733032
Epoch 2/300, train loss: 0.1700970521196723, error mean: 0.0001489206333644688, error std: 0.07033108174800873
Epoch 2/300, val loss: 0.1475073655601591, error mean: -0.001808341359719634, error std: 0.06572529673576355
...
Epoch 299/300, train loss: 0.003378463063199888, error mean: -8.133996743708849e-05, error std: 0.009488753043115139
Epoch 299/300, val loss: 0.004163481352406961, error mean: -0.001996406354010105, error std: 0.010547727346420288
Epoch 300/300, train loss: 0.003569353237253381, error mean: -9.1125002654735e-05, error std: 0.00977678969502449
Epoch 300/300, val loss: 0.004456713928448153, error mean: 0.0008482271223329008, error std: 0.010923181660473347
299 , .
:
import random
def show_output(model, data_loader, batch_num=0, samples=5, grid_shape=(5, 1), figsize=(10, 10)):
for i, (*xs, y) in enumerate(data_loader):
if i == batch_num:
break
xs = [x.cuda() for x in xs]
y_pred = model(*xs).detach().cpu().numpy().reshape(-1, 2)
xs = [x.detach().cpu().numpy().reshape(-1, 16, 32) for x in xs]
imgs_conc = np.hstack(xs)
y = y.cpu().numpy().reshape(-1, 2)
indices = random.sample(range(len(y_pred)), samples)
fig, axes = plt.subplots(*grid_shape, figsize=figsize)
for i, index in enumerate(indices):
row = i // grid_shape[1]
column = i % grid_shape[1]
axes[row, column].imshow(imgs_conc[index])
axes[row, column].scatter([y_pred[index, 1]*32, y_pred[index, 1]*32], [y_pred[index, 0]*16, (y_pred[index, 0]+1)*16], c="r")
axes[row, column].scatter([y[index, 1]*32, y[index, 1]*32], [y[index, 0]*16, (y[index, 0]+1)*16], c="g")
eyesnet.load_state_dict(torch.load("eyes_net/epoch_299.pth"))
show_output(eyesnet, eyes_val_loader, 103, 16, (4, 4))
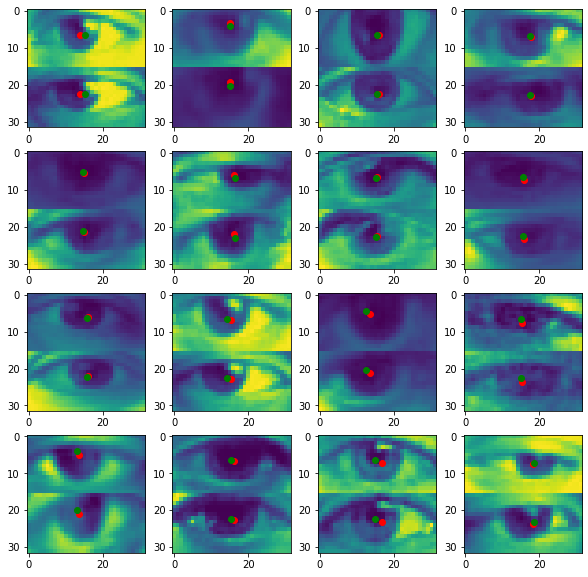
, , "" , . — -, - , -, . , .
( X Y), :
def error_distribution(model, data_loader, image_shape=(16, 32), bins=32, digits=2, figsize=(10,10)):
ys_true = []
ys_pred = []
for *xs, y in data_loader:
xs = [x.cuda() for x in xs]
y_pred = model(*xs)
ys_true.append(y.detach().cpu().numpy())
ys_pred.append(y_pred.detach().cpu().numpy())
ys_true = np.concatenate(ys_true)
ys_pred = np.concatenate(ys_pred)
indices = np.arange(len(ys_true))
fig, axes = plt.subplots(2, figsize=figsize)
for ax_num in range(2):
ys_true_subset = ys_true[:, ax_num]
ys_pred_subset = ys_pred[:, ax_num]
counts, ranges = np.histogram(ys_true_subset, bins=bins)
errors = []
labels = []
for i in range(len(counts)):
begin, end = ranges[i], ranges[i + 1]
range_indices = indices[(ys_true_subset >= begin) & (ys_true_subset <= end)]
diffs = np.abs(ys_pred_subset[range_indices] - ys_true_subset[range_indices])
label = (begin + end) / 2
if image_shape:
diffs = diffs * image_shape[ax_num]
label = label * image_shape[ax_num]
else:
label = round(label, digits)
errors.append(diffs)
labels.append(str(label)[:2+digits])
axes[ax_num].boxplot(errors, labels=labels)
if image_shape:
y_label = "difference, px"
x_label = "true position, px"
else:
y_label = "difference"
x_label = "true position"
axes[ax_num].set_ylabel(y_label)
axes[ax_num].set_xlabel(x_label)
if ax_num == 0:
title = "Y"
else:
title = "X"
axes[ax_num].set_title(title)
error_distribution(eyesnet, eyes_val_loader, figsize=(20, 10))

,
-, . , . :
import time
def measure_time(model, data_loader, n_batches=5):
begin_time = time.time()
batch_num = 0
n_samples = 0
predicted = []
for *xs, y in data_loader:
xs = [x.cpu() for x in xs]
y_pred = model(*xs)
predicted.append(y_pred.detach().cpu().numpy().reshape(-1))
batch_num += 1
n_samples += len(y)
if batch_num >= n_batches:
break
end_time = time.time()
time_per_sample = (end_time - begin_time) / n_samples
return time_per_sample
eyesnet_cpu = EyesNet().cpu()
eyesnet_cpu.load_state_dict(torch.load("eyes_net/epoch_299.pth", map_location="cpu"))
_, eyes_val_loader_single = make_dataloaders(*eyes_datasets, batch_size=1)
tps = measure_time(eyesnet_cpu, eyes_val_loader_single)
print(f"{tps} seconds per sample")
>>> 0.003347921371459961 seconds per sample
, VGG16 ( , ):
import torchvision.models as models
class VGG16Based(nn.Module):
def __init__(self):
super(VGG16Based, self).__init__()
self.vgg = models.vgg16(pretrained=False)
self.vgg.classifier = nn.Sequential(
nn.Linear(25088, 256),
nn.LeakyReLU(),
nn.Linear(256, 2),
nn.Sigmoid()
)
def forward(self, x_left, x_right):
x_mid = (x_left + x_right) / 2
x = torch.cat((x_left, x_mid, x_right), dim=1)
x_pad = torch.zeros((x.shape[0], 3, 32, 32))
x_pad[:, :, :16, :] = x
x = self.vgg(x_pad)
return x
vgg16 = VGG16Based()
vgg16_tps = measure_time(vgg16, eyes_val_loader_single)
print(f"{vgg16_tps} seconds per sample")
>>> 0.023713159561157226 seconds per sample
, (AMD A10-4600M APU, 1500 MHz):
python benchmark.py
0.003980588912963867 seconds per sample, EyesNet
0.12246298789978027 seconds per sample, VGG16-based
, , , ( VGG16 80 , EyesNet — 1 ; , , ). , , . , :
- ( ).
- . , float8 float32 ( , , ).
- PyTorch Mobile — PyTorch . .
- . — GazeCapture. , , — :
- TFLite — TensorFlow . !
, . Data science ( — *^*) . — FARADAY Lab. — , .
c:
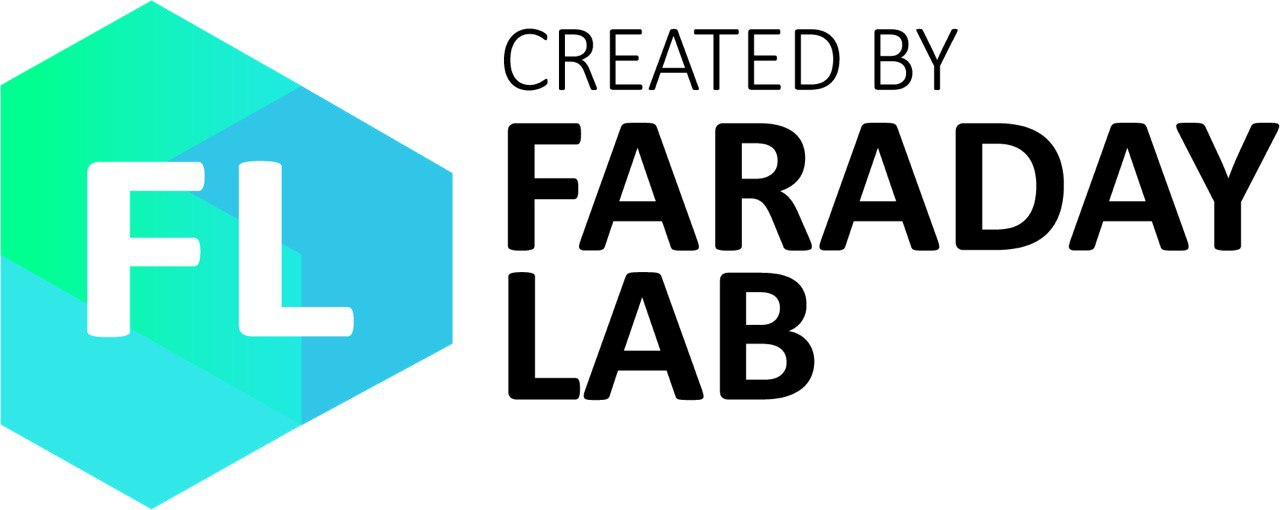
: