L'apprentissage en profondeur ressemble parfois à de la pure magie, surtout lorsque l'ordinateur apprend à faire quelque chose de vraiment créatif, par exemple, pour peindre des images! La technologie utilisée pour cela s'appelle GAN, un réseau génératif compétitif, et dans cet article, nous verrons comment ces réseaux sont organisés et comment les former à générer des images à l'aide d'Azure Machine Learning.
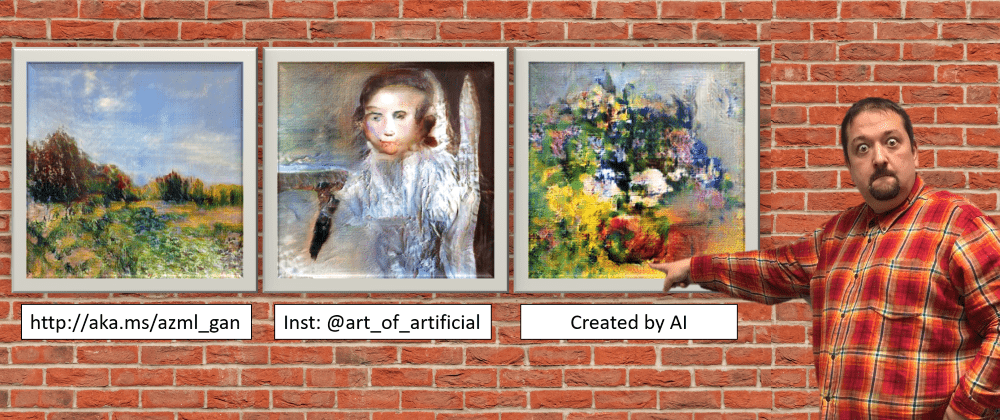
Ce message fait partie de l'initiative AI April . Chaque jour d'avril, mes collÚgues de Microsoft écrivent des articles intéressants sur l'IA et l'apprentissage automatique. Regardez le calendrier - tout à coup, vous y trouverez d'autres sujets qui vous intéressent. Les articles sont principalement en anglais.
Azure ML VS Code ), , Azure ML. , "" MNIST. Azure ML â , , :
WikiArt. , , , WikiArt Retriever, WikiArt Dataset GANGogh.
, dataset
. , , :
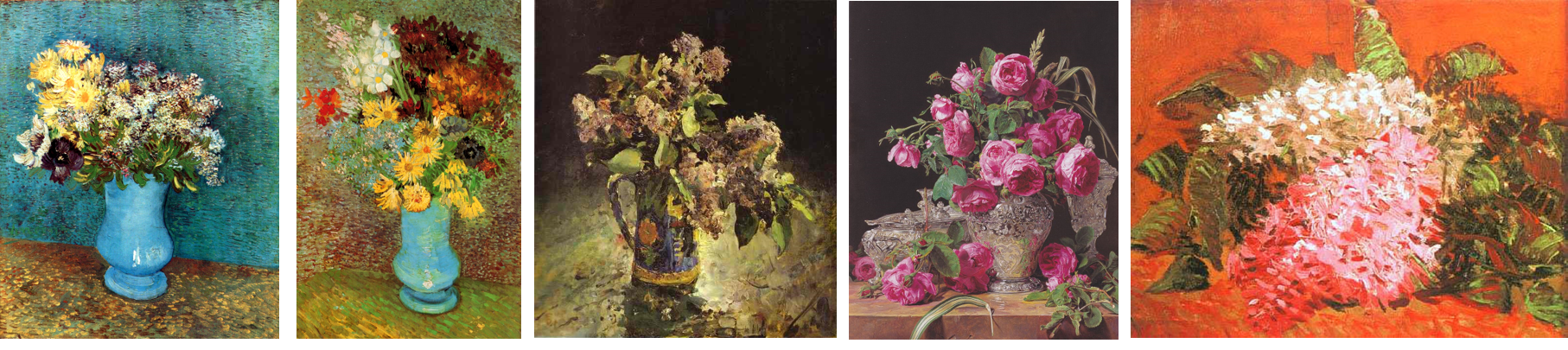
, , ( , ), (, ) .
-
- (GAN).
GAN keragan Keras, .
GAN :
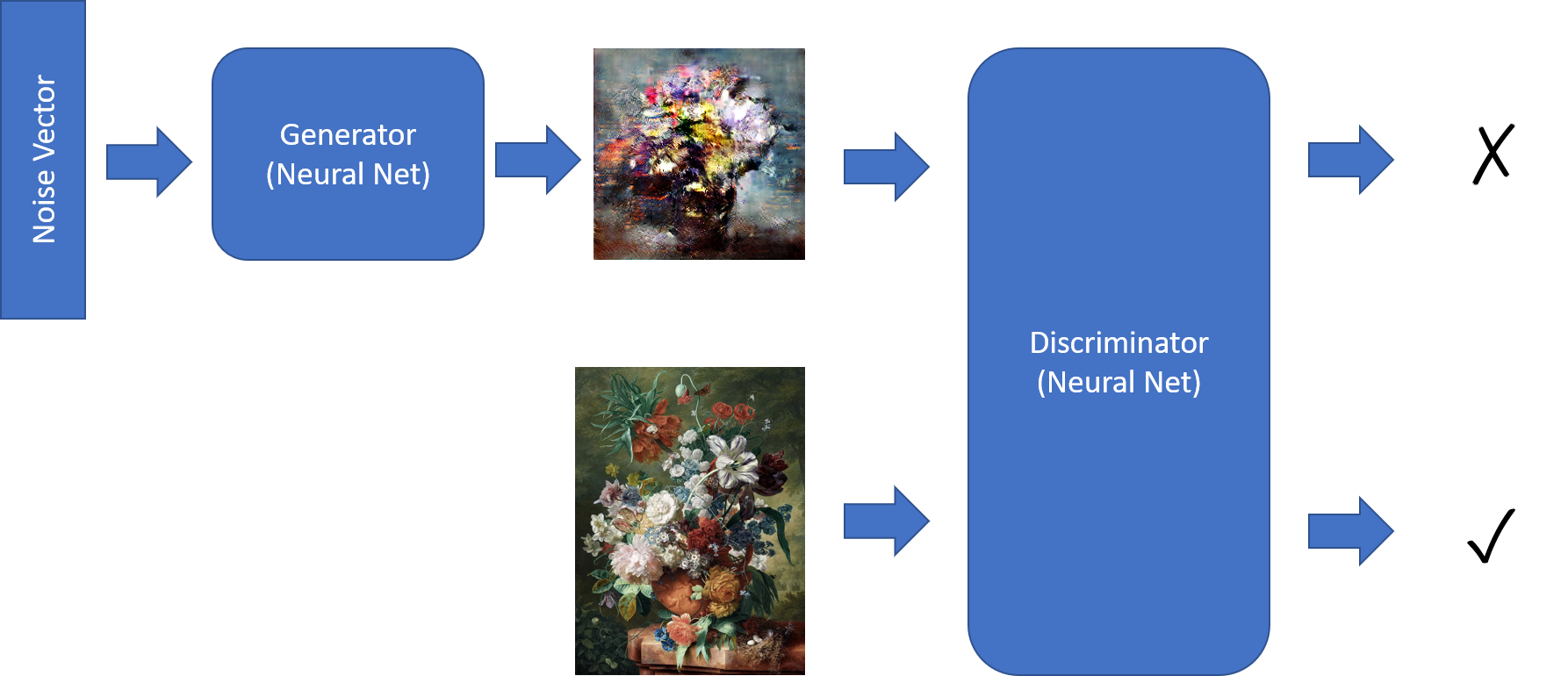
GAN :
- :
noise = np.random.normal(0, 1, (batch_size, latent_dim))
gen_imgs = generator.predict(noise)
imgs = get_batch(batch_size)
- .
ones
zeros
:
d_loss_r = discriminator.train_on_batch(imgs, ones)
d_loss_f = discriminator.train_on_batch(gen_imgs, zeros)
d_loss = np.add(d_loss_r , d_loss_f)*0.5
- , , :
g_loss = combined.train_on_batch(noise, ones)
, â :
discriminator = create_discriminator()
generator = create_generator()
discriminator.compile(loss='binary_crossentropy',optimizer=optimizer,
metrics=['accuracy'])
discriminator.trainable = False
z = keras.models.Input(shape=(latent_dim,))
img = generator(z)
valid = discriminator(img)
combined = keras.models.Model(z, valid)
combined.compile(loss='binary_crossentropy', optimizer=optimizer)
, (CNN). 64x64 :
discriminator = Sequential()
for x in [16,32,64]:
discriminator.add(Conv2D(x, (3,3), strides=1, padding="same"))
discriminator.add(AveragePooling2D())
discriminator.addBatchNormalization(momentum=0.8))
discriminator.add(LeakyReLU(alpha=0.2))
discriminator.add(Dropout(0.3))
discriminator.add(Flatten())
discriminator.add(Dense(1, activation='sigmoid'))
3 :
- 64x64x3 16- , (
AveragePooling2D
) 32x32x16. - 32x32x16 16x16x32
- 8x8x64.
, ( â Dense
).
. , , â latent_dim=100
. , , 100..
â 100 . . UpSampling2D
, :
generator = Sequential()
generator.add(Dense(8 * 8 * 2 * size, activation="relu",
input_dim=latent_dim))
generator.add(Reshape((8, 8, 2 * size)))
for x in [64;32;16]:
generator.add(UpSampling2D())
generator.add(Conv2D(x, kernel_size=(3,3),strides=1,padding="same"))
generator.add(BatchNormalization(momentum=0.8))
generator.add(Activation("relu"))
generator.add(Conv2D(3, kernel_size=3, padding="same"))
generator.add(Activation("tanh"))
64x64x3, . tanh
[-1;1] â , . , , ImageDataset
, .
Azure ML
- , Azure ML !
, , . Azure ML , (accuracy) (loss). run.log
, , Azure ML.
, , ( ) . , , .. - , .
, Azure ML , . log_image
, numpy-, , matplotlib
. , , . callbk
, keragan
:
def callbk(tr):
if tr.gan.epoch % 20 == 0:
res = tr.gan.sample_images(n=3)
fig,ax = plt.subplots(1,len(res))
for i,v in enumerate(res):
ax[i].imshow(v[0])
run.log_image("Sample",plot=plt)
:
gan = keragan.DCGAN(args)
imsrc = keragan.ImageDataset(args)
imsrc.load()
train = keragan.GANTrainer(image_dataset=imsrc,gan=gan,args=args)
train.train(callbk)
, keragan
, args
, , , , learning rate ..
Azure ML VS Code, , SDK, Azure ML. submit_gan.ipynb, :
- :
ws = Workspace.from_config()
- :
cluster = ComputeTarget(workspace=ws, name='My Cluster')
. GPU, [NC6][AzureVMNC]. - :
ds.upload(...)
.
, , :
exp = Experiment(workspace=ws, name='KeraGAN')
script_params = {
'--path': ws.get_default_datastore(),
'--dataset' : 'faces',
'--model_path' : './outputs/models',
'--samples_path' : './outputs/samples',
'--batch_size' : 32,
'--size' : 512,
'--learning_rate': 0.0001,
'--epochs' : 10000
}
est = TensorFlow(source_directory='.',
script_params=script_params,
compute_target=cluster,
entry_script='train_gan.py',
use_gpu = True,
conda_packages=['keras','tensorflow','opencv','tqdm','matplotlib'],
pip_packages=['git+https://github.com/shwars/keragan@v0.0.1']
run = exp.submit(est)
model_path=./outputs/models
samples_path=./outputs/samples
, ( ) outputs
. , , Azure ML.
Estimator
, GPU, Tensorflow
. Estimator
, " " . Estimator
- .
â , keragan
GitHub. PyPI pip
-, , GitHub , commit ID. , PyPI.
, Azure ML Portal:
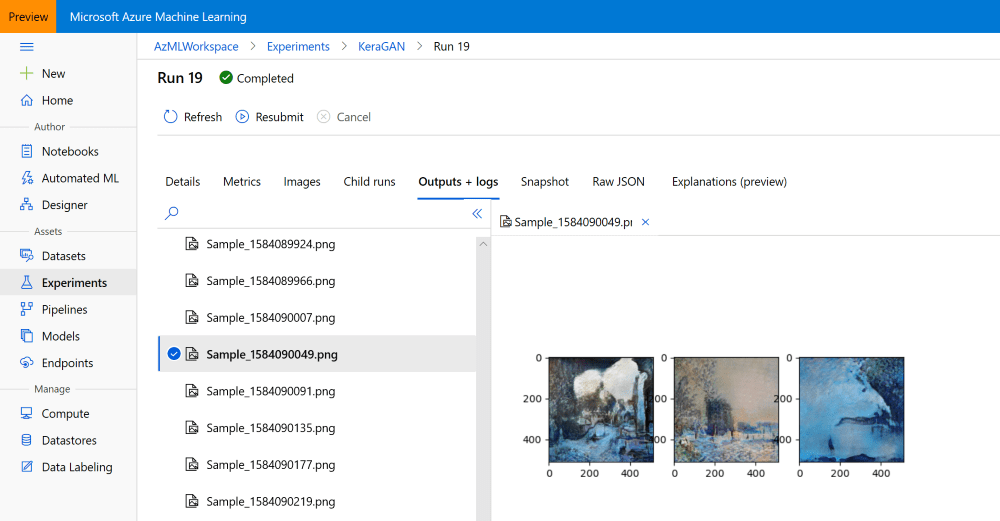
GAN , . -, learning rate: , â . .
:
--size
, . (64 128) , ( 1024) . 1024 , , progressive growing--learning_rate
. , .--dateset
. , , Azure ML datastore, .
, for
, . , . .
, , . outputs/models
, â outputs/samples
. Azure ML Portal :
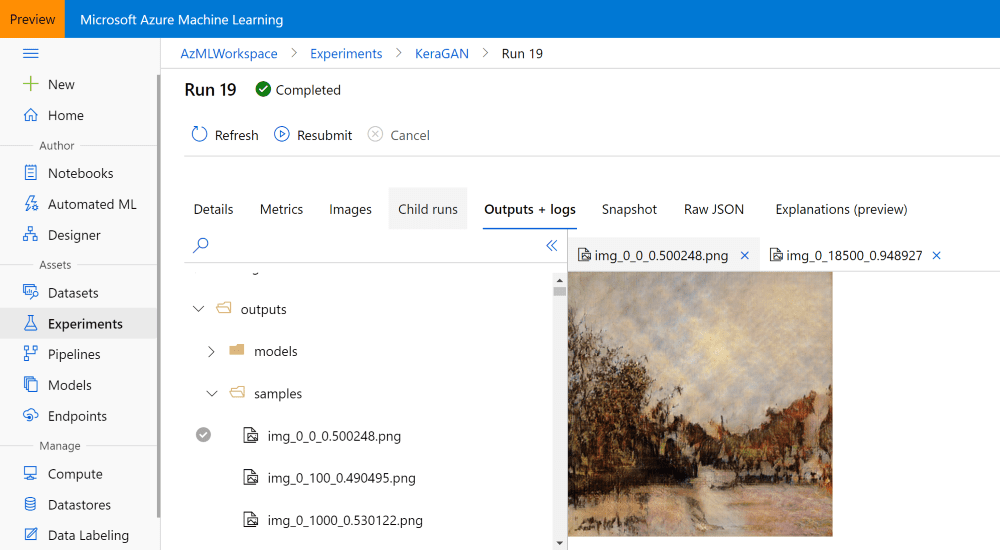
, , . run
, , , ( ):
run.download_files(prefix='outputs/samples')
outputs/samples
, .
run
( , ), , run id, :
run = Run(experiment=exp,run_id='KeraGAN_1584082108_356cf603')
. , , . , ( gen_
):
fnames = run.get_file_names()
fnames = filter(lambda x : x.startswith('outputs/models/gen_'),fnames)
: outputs/models/gen_0.h5
, outputs/models/gen_100.h5
.. :
no = max(map(lambda x: int(x[19:x.find('.')]), fnames))
fname = 'outputs/models/gen_{}.h5'.format(no)
fname_wout_path = fname[fname.rfind('/')+1:]
run.download_file(fname)
, fname_wout_path
.
, Keras, , , , :
model = keras.models.load_model(fname_wout_path)
latent_dim=model.layers[0].input.shape[1].value
res = model.predict(np.random.normal(0,1,(10,latent_dim)))
, [-1,1], [0,1], matplotlib
:
res = (res+1.0)/2
fig,ax = plt.subplots(1,10,figsize=(15,10))
for i in range(10):
ax[i].imshow(res[i])
:

, :
( ) , â @art_of_artificial, .
, - . , , . , :
- , Azure. , . , â â . ? ...
keragan, , DCGAN, Maxime Ellerbach, GANGogh. GAN Keras .
Azure ML