Avez-vous eu une telle chose que vous avez aimĂ© une chose dans la boutique en ligne, mais ne voulez pas l'acheter sans l'essayer? Bien sĂ»r, dans certains magasins, il est possible d'essayer des vĂȘtements aprĂšs la commande avant le paiement. Cependant, selon les statistiques, la part des commandes en ligne dans les magasins en ligne de vĂȘtements et de chaussures augmente chaque annĂ©e, mais la part des retours augmente Ă©galement, elle s'Ă©lĂšve Ă 50-70% - ce sont des coĂ»ts logistiques Ă©normes qui peuvent ĂȘtre considĂ©rablement rĂ©duits en utilisant la cabine d'essayage en ligne. Imaginez que vous tĂ©lĂ©chargez votre photo, choisissez des vĂȘtements et elle est transfĂ©rĂ©e sur votre image. Des cabines d'essayage virtuelles pour chaussures existent dĂ©jĂ , elles fonctionnent assez bien. Il y a quelque temps, ce sujet nous intĂ©ressait, qu'en est-il des vĂȘtements? De telles Ćuvres existent aussi, mais beaucoup moins rĂ©ussies; dans beaucoup dâentre elles, en plus de lâarticle, rien ne se trouve,On ne peut que rĂȘver d'un exemple de travail. Nous avons dĂ©cidĂ© de rĂ©soudre ce problĂšme et de prendre en charge l'un des rĂ©seaux de la bibliothĂšque OpenCV. Ce qui est arrivĂ© de cela peut ĂȘtre vu dansexemple virtual_try_on.py .
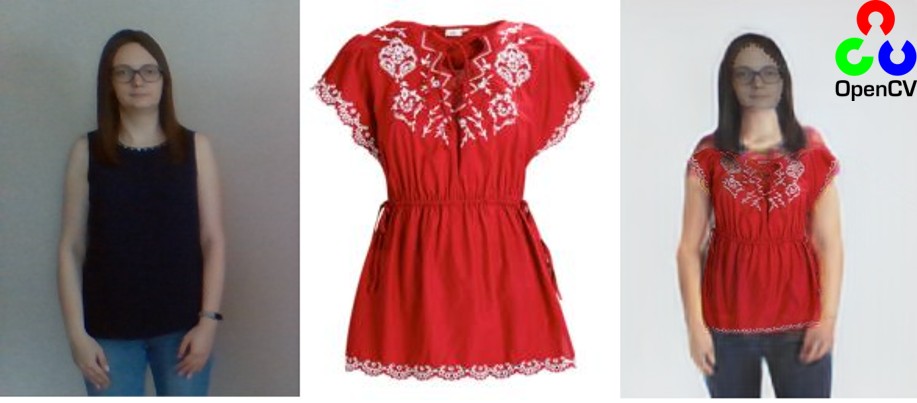
Le résultat n'est pas parfait, mais dans ce domaine est considéré comme assez bon.
Si vous voulez savoir comment fonctionne la cabine d'essayage virtuelle et quelles difficultés nous avons rencontrées lors de l'intégration du modÚle dans OpenCV - bienvenue au chat!
2019 CP-VTON . CP-VTON , , (, ). 3D , 3D-. . github. CP-VTON , , .
.
CP-VTON : GMM (Geometric Matching Module) â TOM (Try-On Module) â .
GMM , TOM â , â , â , â GMM, â ground truth ( ), â ground truth ( , ). , . VITON, . , , , , (, , ). . , GMM , . TOM , .
. , . OpenPose. . LIP_JPPNet. OpenCV () sample human_parsing.py.
, â , : , , . , .

.
GMM . , , . . . , . .

TOM . Unet. , . Unet , . , Unet . , . Upsample . (encoder) VGG-19. . Unet . , .
â .

Try-On â . . , , . perceptual loss. VGG , . VGG â , .
OpenCV
. json , OpenPose Caffe, LIP.
, , .
python3 virtual_try_on.py -i person_img.jpg -c cloth.jpg
OpenCV PIL. . LIP , . CP-VTON . , :
shape = (segm > 0).astype(np.float32)
head = (segm == 1).astype(np.float32) + \
(segm == 2).astype(np.float32) + \
(segm == 4).astype(np.float32) + \
(segm == 13).astype(np.float32)
cloth = (segm == 5).astype(np.float32) + \
(segm == 6).astype(np.float32) + \
(segm == 7).astype(np.float32)
, PIL , . human_colormap.mat. ? Matlab . , scipy . () .
â . 16 , .
mask = mask.resize((width // 16, height // 16), Image.BILINEAR)
mask = mask.resize((width, height), Image.BILINEAR)
. . , OpenCV. PIL resize cv.resize .

, PIL resize, â cv.resize.
, ? .

, PIL resize, â cv.resize.
, . ? , . , â . , bilinear resize bilinear, area. scale factor, 33 = 16 * 2 + 1, OpenCV â 3. , , . . . , . . :
PILclass BilinearFilter(object):
"""
PIL bilinear resize implementation
image = image.resize((image_width // 16, image_height // 16), Image.BILINEAR)
"""
def _precompute_coeffs(self, inSize, outSize):
filterscale = max(1.0, inSize / outSize)
ksize = int(np.ceil(filterscale)) * 2 + 1
kk = np.zeros(shape=(outSize * ksize, ), dtype=np.float32)
bounds = np.empty(shape=(outSize * 2, ), dtype=np.int32)
centers = (np.arange(outSize) + 0.5) * filterscale + 0.5
bounds[::2] = np.where(centers - filterscale < 0, 0, centers - filterscale)
bounds[1::2] = np.where(centers + filterscale > inSize, inSize, centers + filterscale) - bounds[::2]
xmins = bounds[::2] - centers + 1
points = np.array([np.arange(row) + xmins[i] for i, row in enumerate(bounds[1::2])]) / filterscale
for xx in range(0, outSize):
point = points[xx]
bilinear = np.where(point < 1.0, 1.0 - abs(point), 0.0)
ww = np.sum(bilinear)
kk[xx * ksize : xx * ksize + bilinear.size] = np.where(ww == 0.0, bilinear, bilinear / ww)
return bounds, kk, ksize
def _resample_horizontal(self, out, img, ksize, bounds, kk):
for yy in range(0, out.shape[0]):
for xx in range(0, out.shape[1]):
xmin = bounds[xx * 2 + 0]
xmax = bounds[xx * 2 + 1]
k = kk[xx * ksize : xx * ksize + xmax]
out[yy, xx] = np.round(np.sum(img[yy, xmin : xmin + xmax] * k))
def _resample_vertical(self, out, img, ksize, bounds, kk):
for yy in range(0, out.shape[0]):
ymin = bounds[yy * 2 + 0]
ymax = bounds[yy * 2 + 1]
k = kk[yy * ksize: yy * ksize + ymax]
out[yy] = np.round(np.sum(img[ymin : ymin + ymax, 0:out.shape[1]] * k[:, np.newaxis], axis=0))
def imaging_resample(self, img, xsize, ysize):
height, width, *args = img.shape
bounds_horiz, kk_horiz, ksize_horiz = self._precompute_coeffs(width, xsize)
bounds_vert, kk_vert, ksize_vert = self._precompute_coeffs(height, ysize)
out_hor = np.empty((img.shape[0], xsize), dtype=np.uint8)
self._resample_horizontal(out_hor, img, ksize_horiz, bounds_horiz, kk_horiz)
out = np.empty((ysize, xsize), dtype=np.uint8)
self._resample_vertical(out, out_hor, ksize_vert, bounds_vert, kk_vert)
return out
4 , , OpenCV. . , . , . 256 192, . sample . , - , .
P.S. 2020 OpenCV 20-. . !