Bloc-notes CoLab avec des exemples.
Il est possible de crĂ©er une fenĂȘtre dĂ©roulante (fenĂȘtre dĂ©roulante, fenĂȘtre coulissante, fenĂȘtre mobile) sur des tableaux NumPy dans le langage de programmation Python sans boucles explicites . Cet article explique la crĂ©ation de fenĂȘtres coulissantes Ă une, deux, trois et N dimensions sur des tableaux NumPy. Par consĂ©quent, le traitement des donnĂ©es augmente la vitesse de plusieurs milliers de fois et est comparable Ă la vitesse avec le C langage de programmation .
Une fenĂȘtre glissante est utilisĂ©e dans: le traitement d'images, les rĂ©seaux de neurones artificiels, le protocole Internet TCP, le traitement des donnĂ©es gĂ©nomiques, la prĂ©vision des sĂ©ries chronologiques, etc.
Avertissement : il peut y avoir des erreurs dans le code source! Si vous voyez une erreur, écrivez-moi.
introduction
Cet article fait suite Ă ma rĂ©ponse sur le site Web de StackOverflow. Mes premiĂšres expĂ©riences avec une fenĂȘtre coulissante ici et ici .
La mise en Ćuvre pratique d'une fenĂȘtre coulissante bidimensionnelle sur un tableau d'images bidimensionnelles est en fonction du roll
dossier de logic_tools.py
projet Marquage manuel des images Ă l'aide de polygones .
Des algorithmes pour une fenĂȘtre coulissante unidimensionnelle sont dĂ©jĂ implĂ©mentĂ©s ici , ici et ici .
, , (strides, ).
- Pandas, Pandas, , . , . , Cython, - , NumPy.
1. 1D ND Numpy

:
def roll(a,
b,
dx=1):
shape = a.shape[:-1] + (int((a.shape[-1] - b.shape[-1]) / dx) + 1,) + b.shape
strides = a.strides[:-1] + (a.strides[-1] * dx,) + a.strides[-1:]
return np.lib.stride_tricks.as_strided(a, shape=shape, strides=strides)
numpy.lib.stride_tricks.as_strided
(view) (shape) (strides).
(shape) , , . (strides) .
(shape) :
a.shape[:-1]
â ND-, N > 1
. N == 1
, t == ()
, N == 1
.(int((a.shape[-1] - b.shape[-1]) / dx) + 1,)
â [-1]
. dx
: 1, 2, 3 ..b.shape
â .
(strides) :
a.strides[:-1]
â ND-, N > 1
. N == 1
, t == ()
, N == 1
.(a.strides[-1] * dx,)
â . , int
4 , dx == 2
4 * 2 = 8
.a.strides[-1:]
â . , int
4 , (4,)
.
2. 2D ND Numpy

2D 2D :
, 2D - . , , , , .. , , .
def roll(a,
b,
dx=1,
dy=1):
shape = a.shape[:-2] + \
((a.shape[-2] - b.shape[-2]) // dy + 1,) + \
((a.shape[-1] - b.shape[-1]) // dx + 1,) + \
b.shape
strides = a.strides[:-2] + \
(a.strides[-2] * dy,) + \
(a.strides[-1] * dx,) + \
a.strides[-2:]
return np.lib.stride_tricks.as_strided(a, shape=shape, strides=strides)
: , , â ((a.shape[-2] - b.shape[-2]) // dy + 1,)
. :
(int((a.shape[-1] - b.shape[-1]) / dx) + 1,)
((a.shape[-1] - b.shape[-1]) // dx + 1,)
.
() , (a.strides[-2] * dy,)
2D .
counts
, coords
:
def show_results(a, b, dx=1, dy=1):
n = a.ndim
bool_array = np.all(roll(a, b, dx, dy) == b, axis=(n, n+1))
counts = np.count_nonzero(bool_array)
coords = np.transpose(np.nonzero(bool_array)) * [dy, dx]
print("Found {counts} elements with coordinates:\n{coords}".format(
counts=counts, coords=coords))
np.all
2D 4D . coords
[dy, dx]
.
3. 3D ND Numpy
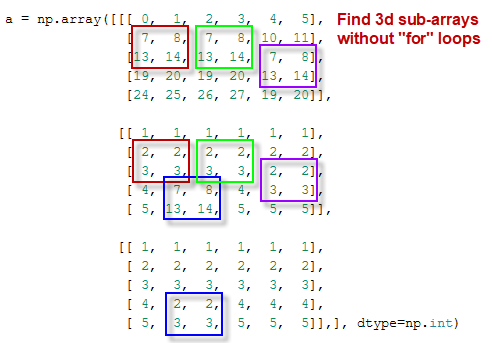
() - . , 3D ND- .
3D 3D â ( ) . CoLab 3D - , (, , ..).
def roll(a,
b,
dx=1,
dy=1,
dz=1):
shape = a.shape[:-3] + \
((a.shape[-3] - b.shape[-3]) // dz + 1,) + \
((a.shape[-2] - b.shape[-2]) // dy + 1,) + \
((a.shape[-1] - b.shape[-1]) // dx + 1,) + \
b.shape
strides = a.strides[:-3] + \
(a.strides[-3] * dz,) + \
(a.strides[-2] * dy,) + \
(a.strides[-1] * dx,) + \
a.strides[-3:]
return np.lib.stride_tricks.as_strided(a, shape=shape, strides=strides)
counts
coords
:
def show_results(a, b, dx=1, dy=1, dz=1):
n = a.ndim
bool_array = np.all(roll(a, b, dx, dy, dz) == b, axis=(n, n+1, n+2))
counts = np.count_nonzero(bool_array)
coords = np.transpose(np.nonzero(bool_array)) * [dz, dy, dx]
print("Found {counts} elements with coordinates:\n{coords}".format(
counts=counts, coords=coords))
4. MD ND , M †N

roll
show_results
MD ND , M N : M †N.
def roll(a,
b,
d=None):
n = a.ndim
m = b.ndim
if m > n:
print("Error: rolling window dimensions is larger than the array dims")
return None
if d is None:
d = np.ones(m, dtype=np.uint32)
elif d.ndim != 1 and d.size != m:
print("Error: steps number must be equal to rolling window dimensions")
return None
elif not np.issubdtype(d.dtype, np.integer) or \
not (d > 0).all():
print("Error: steps must be integer and > 0")
return None
s = np.flip(d)
sub = np.subtract(a.shape[-m:], b.shape[-m:])
steps = tuple(np.divide(sub, s).astype(np.uint32) + 1)
shape = a.shape[:-m] + steps + b.shape
section = tuple(np.multiply(a.strides[-m:], s))
strides = a.strides[:-m] + section + a.strides[-m:]
return np.lib.stride_tricks.as_strided(a, shape=shape, strides=strides)
roll
. :
steps = tuple(np.divide(sub, s).astype(np.uint32) + 1)
â .section = tuple(np.multiply(a.strides[-m:], s))
â () « ».- « »
section
ND-: strides = a.strides[:-m] + section + a.strides[-m:]
.
counts
coords
:
def show_results(a, b, d=None):
n = a.ndim
m = b.ndim
if d is None:
d = np.ones(m, dtype=np.uint32)
bool_array = roll(a, b, d) == b
bool_array = np.all(bool_array, axis=tuple(range(n, n + m)))
counts = np.count_nonzero(bool_array)
s = np.concatenate((np.ones(n-m, dtype=int), np.flip(d)))
coords = np.transpose(np.nonzero(bool_array)) * s
print("Found {counts} elements with coordinates:\n{coords}".format(
counts=counts, coords=coords))
show_results
:
- ()
bool_array
. numpy.all
m
, True
. , bool_array
â (N+M)D , np.all
m
MD :
bool_array = roll(a, b, d) == b
bool_array = np.all(bool_array, axis=tuple(range(n, n + m)))
5. MD ND M N

MD ND , M > N? , ! ND , MD M > N.
MD ND . MD ND M N. roll
show_results
.
def get_results(a, b, d=None):
n = a.ndim
m = b.ndim
if d is None:
d = np.ones(m, dtype=np.uint32)
bool_array = roll(a, b, d) == b
bool_array = np.all(bool_array, axis=tuple(range(n, n + m)))
counts = np.count_nonzero(bool_array)
s = np.concatenate((np.ones(n-m, dtype=int), np.flip(d)))
coords = np.transpose(np.nonzero(bool_array)) * s
return (counts, coords)
def show_intersections(a, b, d=None):
d_tmp = d
n = a.ndim
m = b.ndim
if d_tmp is None:
d_tmp = np.ones(m, dtype=np.uint32)
elif m > n and d_tmp.size == n:
d_tmp = np.concatenate((np.ones(m-n, dtype=int), d_tmp))
counts = 0
coords = None
if m <= n:
results = get_results(a, b, d_tmp)
counts = results[0]
coords = results[1]
else:
t = m - n
layers = np.prod(b.shape[:t])
temp = b.reshape((layers,) + b.shape[t:])
for i in range(layers):
results = get_results(a, temp[i], d_tmp[t:])
counts += results[0]
if coords is None:
coords = results[1]
else:
coords = np.concatenate((coords, results[1]))
print("Found {counts} elements with coordinates:\n{coords}".format(
counts=counts, coords=coords))
get_results
, show_results
.
show_intersections
. M <= N
, show_intersections
get_results
, . M > N
, b
a
.
t = m - n
MD b
ND a
. b
a
: layers = np.prod(b.shape[:t])
. ( , reshape) b
MD (N+1)D :
temp = b.reshape((layers,) + b.shape[t:])
: (N+1)D
ND
, (N+1)
layers
:
for i in range(layers):
results = get_results(a, temp[i], d_tmp[t:])
Combinez le nombre de correspondances counts
et les coordonnées trouvées de ces correspondances coords
pour chaque couche:
for i in range(layers):
results = get_results(a, temp[i], d_tmp[t:])
counts += results[0]
if coords is None:
coords = results[1]
else:
coords = np.concatenate((coords, results[1]))
Tous les exemples sont dans le bloc-notes CoLab .
Merci pour l'attention!