La formation des réseaux de neurones à la reconnaissance des formes est un processus long et gourmand en ressources. Surtout quand à portée de main, il n'y a qu'un ordinateur portable peu coûteux, et pas un ordinateur avec une carte graphique puissante. Dans ce cas, Google Colaboratory viendra à la rescousse , qui propose d'utiliser le GPU de niveau Tesla K80 absolument gratuit ( plus ).
Cet article décrit le processus de préparation des données, la formation du modèle tensorflow dans Google Colaboratory et son lancement sur un appareil Android.
Préparation des données
Par exemple, essayons de former un réseau de neurones pour reconnaître les dés blancs sur fond noir. En conséquence, pour commencer, vous devez créer un ensemble de données suffisant pour la formation (pour l'instant, arrêtons-nous à environ 100 photos).

Pour la formation, nous utiliserons l' API de détection d'objets Tensorflow . Nous préparerons toutes les données nécessaires à la formation sur ordinateur portable. Nous avons besoin d'un environnement conda et d' un gestionnaire de gestion des dépendances . Instructions d'installation ici .
Créons un environnement de travail:
conda create -n object_detection_prepare pip python=3.6
Et activez-le:
conda activate object_detection_prepare
Définissez les dépendances dont nous avons besoin:
pip install --ignore-installed --upgrade tensorflow==1.14
pip install --ignore-installed pandas
pip install --ignore-installed Pillow
pip install lxml
conda install pyqt=5
object_detection, object_detection/images.
Google Colab , , "tcmalloc: large alloc....".
object_detection/preprocessing .
:
python ./object_detection/preprocessing/image_resize.py -i ./object_detection/images --imageWidth=800 --imageHeight=600
, 800x600 object_detection/images/resized. object_detection/images.
labelImg.
labelImg object_detection
labelImg
cd [FULL_PATH]/object_detection/labelImg
:
pyrcc5 -o libs/resources.py resources.qrc
( ):
python labelImg.py

“Open dir” object_detection/images , . (1, 2, 3, 4, 5, 6). ( *.xml) .
object_detection/training_demo, Google Colab .
( ) 80/20 object_detection/training_demo/images/train object_detection/training_demo/images/test.
object_detection/training_demo/annotations, . label_map.pbtxt, . :
label_map.pbtxtitem {
id: 1
name: '1'
}
item {
id: 2
name: '2'
}
item {
id: 3
name: '3'
}
item {
id: 4
name: '4'
}
item {
id: 5
name: '5'
}
item {
id: 6
name: '6'
}
, ? , TFRecord. [1].
: xml -> csv csv -> record
preprocessing :
cd [FULL_PATH]\object_detection\preprocessing
1. xml csv
:
python xml_to_csv.py -i [FULL_PATH]/object_detection/training_demo/images/train -o [FULL_PATH]/object_detection/training_demo/annotations/train_labels.csv
:
python xml_to_csv.py -i [FULL_PATH]/object_detection/training_demo/images/test -o [FULL_PATH]/object_detection/training_demo/annotations/test_labels.csv
2. csv record
:
python generate_tfrecord.py --label_map_path=[FULL_PATH]\object_detection\training_demo\annotations\label_map.pbtxt --csv_input=[FULL_PATH]\object_detection\training_demo\annotations\train_labels.csv --output_path=[FULL_PATH]\object_detection\training_demo\annotations\train.record --img_path=[FULL_PATH]\object_detection\training_demo\images\train
:
python generate_tfrecord.py --label_map_path=[FULL_PATH]\object_detection\training_demo\annotations\label_map.pbtxt --csv_input=[FULL_PATH]\object_detection\training_demo\annotations\test_labels.csv --output_path=[FULL_PATH]\object_detection\training_demo\annotations\test.record --img_path=[FULL_PATH]\object_detection\training_demo\images\test
, , .
.
ssdlite_mobilenet_v2_coco, android .
object_detection/training_demo/pre-trained-model.
-
object_detection/training_demo/pre-trained-model/ssdlite_mobilenet_v2_coco_2018_05_09
pipeline.config object_detection/training_demo/training ssdlite_mobilenet_v2_coco.config.
, :
1.
model.ssd.num_classes: 6
2. ( ), ,
train_config.batch_size: 18
train_config.num_steps: 20000
train_config.fine_tune_checkpoint:"./training_demo/pre-trained-model/ssdlite_mobilenet_v2_coco_2018_05_09/model.ckpt"
3. (object_detection/training_demo/images/train)
eval_config.num_examples: 64
4.
train_input_reader.label_map_path: "./training_demo/annotations/label_map.pbtxt"
train_input_reader.tf_record_input_reader.input_path:"./training_demo/annotations/train.record"
5.
eval_input_reader.label_map_path: "./training_demo/annotations/label_map.pbtxt"
eval_input_reader.tf_record_input_reader.input_path:"./training_demo/annotations/test.record"
, .
training_demo training_demo.zip Google Drive.
google drive Google Colab,
, .
Google Drive training_demo.zip, Get shareable link id :
drive.google.com/open?id=[YOUR_FILE_ID_HERE]
Google Colab — Google Drive.

CPU. GPU, runtime.


.
:
1. TensorFlow Models:
!git clone https://github.com/tensorflow/models.git
2. protobuf object_detection:
!apt-get -qq install libprotobuf-java protobuf-compiler
%cd ./models/research/
!protoc object_detection/protos/*.proto --python_out=.
%cd ../..
3. PYTHONPATH:
import os
os.environ['PYTHONPATH'] += ":/content/models/research/"
os.environ['PYTHONPATH'] += ":/content/models/research/slim"
os.environ['PYTHONPATH'] += ":/content/models/research/object_detection"
os.environ['PYTHONPATH'] += ":/content/models/research/object_detection/utils"
4. Google Drive PyDrive :
!pip install -U -q PyDrive
from pydrive.auth import GoogleAuth
from pydrive.drive import GoogleDrive
from google.colab import auth
from oauth2client.client import GoogleCredentials
auth.authenticate_user()
gauth = GoogleAuth()
gauth.credentials = GoogleCredentials.get_application_default()
drive = GoogleDrive(gauth)
5. ( id ) :
drive_file_id="[YOUR_FILE_ID_HERE]"
training_demo_zip = drive.CreateFile({'id': drive_file_id})
training_demo_zip.GetContentFile('training_demo.zip')
!unzip training_demo.zip
!rm training_demo.zip
6. :
!python ./models/research/object_detection/legacy/train.py --logtostderr --train_dir=./training_demo/training --pipeline_config_path=./training_demo/training/ssdlite_mobilenet_v2_coco.config
--train_dir=./training_demo/training — ,
--pipeline_config_path=./training_demo/training/ssdlite_mobilenet_v2_coco.config —
7. frozen graph, :
!python /content/models/research/object_detection/export_inference_graph.py --input_type image_tensor --pipeline_config_path /content/training_demo/training/ssdlite_mobilenet_v2_coco.config --trained_checkpoint_prefix /content/training_demo/training/model.ckpt-[CHECKPOINT_NUMBER]
--output_directory /content/training_demo/training/output_inference_graph_v1.pb
--pipeline_config_path /content/training_demo/training/ssdlite_mobilenet_v2_coco.config —
--trained_checkpoint_prefix /content/training_demo/training/model.ckpt-[CHECKPOINT_NUMBER] — , .
--output_directory /content/training_demo/training/output_inference_graph_v1.pb —
[CHECKPOINT_NUMBER], content/training_demo/training/. model.ckpt-1440.index, model.ckpt-1440.meta. 1440 — [CHECKPOINT_NUMBER] .

. ~20000 .

8. tflite.
tensorflow lite tflite. frozen graph tflite ( export_inference_graph.py):
!python /content/models/research/object_detection/export_tflite_ssd_graph.py --pipeline_config_path /content/training_demo/training/ssdlite_mobilenet_v2_coco.config --trained_checkpoint_prefix /content/training_demo/training/model.ckpt-[CHECKPOINT_NUMBER] --output_directory /content/training_demo/training/output_inference_graph_tf_lite.pb
tflite , output_inference_graph_tf_lite.pb:

Netron. .
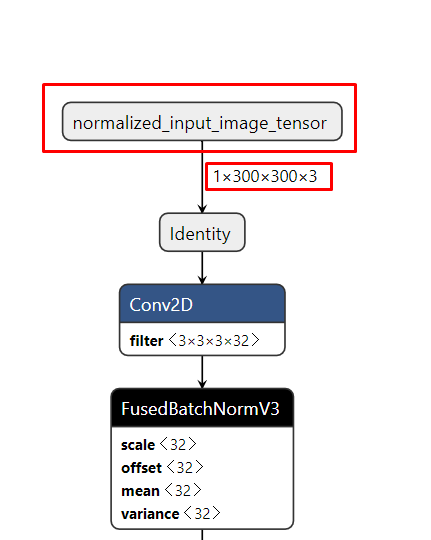

pb tflite :
!tflite_convert --output_file=/content/training_demo/training/model_q.tflite --graph_def_file=/content/training_demo/training/output_inference_graph_tf_lite_v1.pb/tflite_graph.pb --input_arrays=normalized_input_image_tensor --output_arrays='TFLite_Detection_PostProcess','TFLite_Detection_PostProcess:1','TFLite_Detection_PostProcess:2','TFLite_Detection_PostProcess:3' --input_shapes=1,300,300,3 --enable_select_tf_ops --allow_custom_ops --inference_input_type=QUANTIZED_UINT8 --inference_type=FLOAT --mean_values=128 --std_dev_values=128
--output_file=/content/training_demo/training/model_q.tflite —
--graph_def_file=/content/training_demo/training/output_inference_graph_tf_lite_v1.pb/tflite_graph.pb — frozen graph,
--input_arrays=normalized_input_image_tensor — ,
--output_arrays='TFLite_Detection_PostProcess','TFLite_Detection_PostProcess:1','TFLite_Detection_PostProcess:2','TFLite_Detection_PostProcess:3' — ,
--input_shapes=1,300,300,3 — ,
--enable_select_tf_ops — runtime TensorFlow Lite
--allow_custom_ops — TensorFlow Lite Optimizing Converter
--inference_type=FLOAT —
--inference_input_type=QUANTIZED_UINT8 —
--mean_values=128 --std_dev_values=128 — , QUANTIZED_UINT8
Google Drive:
!zip -r ./training_demo/training.zip ./training_demo/training/
training_result = drive.CreateFile({'title': 'training_result.zip'})
training_result.SetContentFile('training_demo/training.zip')
training_result.Upload()
Invalid client secrets file, google drive.
android
android object detection, kotlin CameraX. .
CameraX ImageAnalysis. ObjectDetectorAnalyzer.
:
1. YUV . RGB :
val rgbArray = convertYuvToRgb(image)
2. (, , , 300x300), Bitmap :
val rgbBitmap = getRgbBitmap(rgbArray, image.width, image.height)
val transformation = getTransformation(rotationDegrees, image.width, image.height)
Canvas(resizedBitmap).drawBitmap(rgbBitmap, transformation, null)
3. bitmap , :
ImageUtil.storePixels(resizedBitmap, inputArray)
val objects = detect(inputArray)
4. RecognitionResultOverlayView :
val scaleFactorX = measuredWidth / result.imageWidth.toFloat()
val scaleFactorY = measuredHeight / result.imageHeight.toFloat()
result.objects.forEach { obj ->
val left = obj.location.left * scaleFactorX
val top = obj.location.top * scaleFactorY
val right = obj.location.right * scaleFactorX
val bottom = obj.location.bottom * scaleFactorY
canvas.drawRect(left, top, right, bottom, boxPaint)
canvas.drawText(obj.text, left, top - 25f, textPaint)
}
, assets training_demo/training/model_q.tflite ( detect.tflite) labelmap.txt, :
SSD Mobilenet V1, 1, 0, labelOffset 1 0 collectDetectionResult ObjectDetector.
.
, Xiaomi Redmi 4X :
: