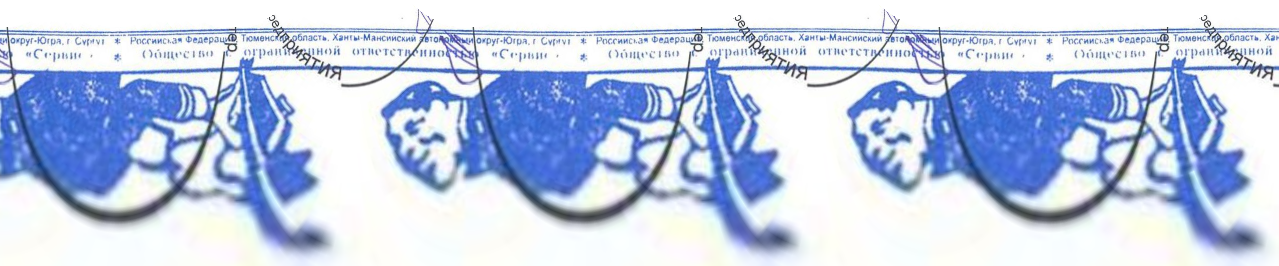
Il n'y a pas si longtemps, nous étions confrontés à la tùche de trouver et d'extraire des tampons de documents. Pourquoi? Par exemple, pour vérifier la présence de scellés dans les contrats de deux parties (parties au contrat). Nous dans les bacs avions déjà un prototype pour les trouver, écrit en OpenCV, mais il était humide. Nous avons décidé de déterrer cette relique, d'en secouer la poussiÚre et de faire une solution de travail sur sa base.
La plupart des techniques dĂ©crites ici peuvent ĂȘtre appliquĂ©es en dehors de la recherche de tampons. Par exemple:
- segmentation des couleurs;
- rechercher des objets / cercles ronds;
- conversion d'image en systÚme de coordonnées polaires;
- intersection d'objets, Intersection over Union (IoU, coefficient Jacquard).
En conséquence, nous avions deux options: résoudre en utilisant des réseaux de neurones ou ressusciter un prototype sur OpenCV. Pourquoi avons-nous décidé de prendre OpenCV? La réponse se trouve à la fin de l'article.
Des exemples de code seront présentés en Python et C #. Pour Python, vous avez besoin des packages opencv-python et numpy , pour C #, vous avez besoin d'OpenCvSharp et d' opencv .
J'espÚre que vous connaissez les algorithmes de base d'OpenCV. Je ne vais pas m'y plonger profondément, car la plupart d'entre eux méritent un article séparé et détaillé, mais je vais laisser des liens avec le tapis. partie de tous assoiffés.
, ( HLS-), . - , , .
. â .
, ? .
. / , , -, , .
, , python . ( , , ). ( Intersection over Union), .
:
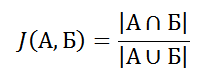
:
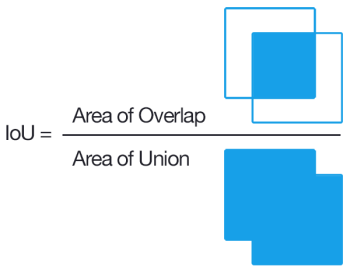
, , , , - . , , , :
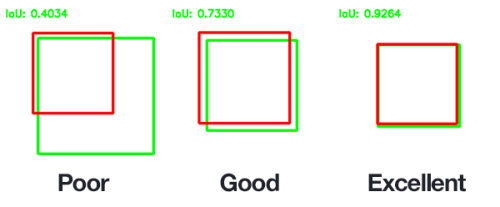
( 0.6) , F1- ( â ).
IoUimport sys
from collections import namedtuple
Metrics = namedtuple("metrics", ["tp", "fp", "fn"])
def bb_intersection_over_union(a, b):
x_a = max(a[0], b[0])
y_a = max(a[1], b[1])
x_b = min(a[2], b[2])
y_b = min(a[3], b[3])
inter_area = max(0, x_b - x_a + 1) * max(0, y_b - y_a + 1)
a_area = (a[2] - a[0] + 1) * (a[3] - a[1] + 1)
b_area = (b[2] - b[0] + 1) * (b[3] - b[1] + 1)
iou = inter_area / float(a_area + b_area - inter_area)
return iou
def same_stamp(a, b):
""", """
iou = bb_intersection_over_union(a, b)
print(f'iou: {iou}')
return iou > 0.6
def compare_stamps(extracted, mapped):
"""
:param extracted: , .
:param mapped: () .
"""
tp = []
fp = []
fn = list(mapped)
for stamp in extracted:
for check in fn:
if same_stamp(stamp, check):
tp.append(check)
fn.remove(check)
break
else:
fp.append(stamp)
return Metrics(len(tp), len(fp), len(fn))
def compare(file, sectors):
"""
.
:param file: .
:param sectors: .
"""
print(f'file: {file}')
try:
stamps = extract_stamps(file)
metrics = compare_stamps(stamps, [ss for ss in sectors if 'stamp' in ss['tags']])
return file, metrics
except:
print(sys.exc_info())
return file, Metrics(0, 0, 0)
if __name__ == '__main__':
file_metrics = {}
for file, sectors in dataset.items():
file_metrics[file] = compare(file, sectors)
total_metrics = Metrics(*(sum(x) for x in zip(*file_metrics.values())))
precision = total_metrics.tp / (total_metrics.tp + total_metrics.fp) if total_metrics.tp > 0 else 0
recall = total_metrics.tp / (total_metrics.tp + total_metrics.fn) if total_metrics.tp > 0 else 0
f1 = 2 * precision * recall / (precision + recall) if (precision + recall) > 0 else 0
print('precision\trecall\tf1')
print('{:.4f}\t{:.4f}\t{:.4f}'.format(precision * 100, recall * 100, f1 * 100).replace('.', ','))
print('tp\tfp\tfn')
print('{}\t{}\t{}'.format(total_metrics.tp, total_metrics.fp, total_metrics.fn))
print('tp\tfp\tfn')
for file in dataset.keys():
metric = file_metrics.get(file)
print(f'{metric.tp}\t{metric.fp}\t{metric.fn}')
print(f'precision: {precision}, recall: {recall}, f1: {f1}, {total_metrics}')
HLS-
() - . , . ( ):
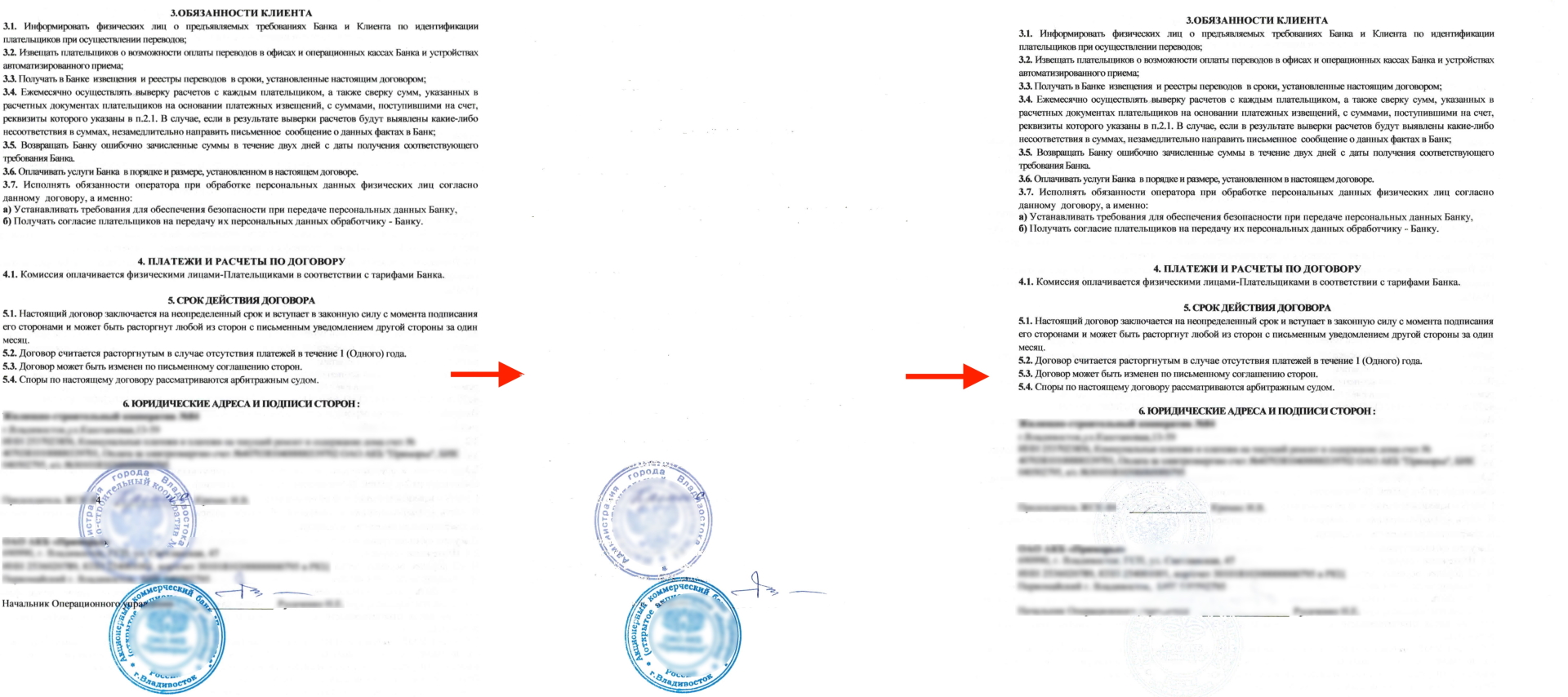
.
HLS . Hue, Lightness, Saturation, , , . :
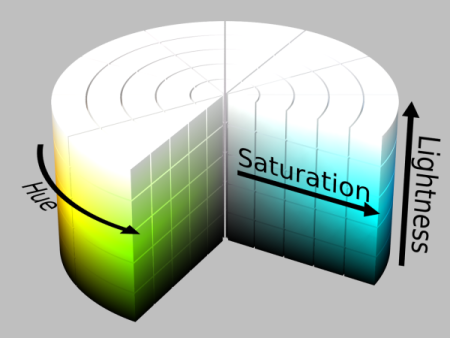
? - . , ~180 ~280.
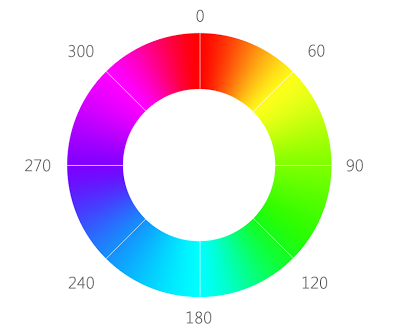
, - , , .
(Python)def colored_mask(img, threshold = -1):
denoised = cv2.medianBlur(img, 3)
cv2.imwrite('denoised.bmp', denoised)
gray = cv2.cvtColor(denoised, cv2.COLOR_BGR2GRAY)
cv2.imwrite('gray.bmp', gray)
adaptiveThreshold = threshold if threshold >= 0 else cv2.mean(img)[0]
color = cv2.cvtColor(denoised, cv2.COLOR_BGR2HLS)
mask = cv2.inRange(color, (0, int(adaptiveThreshold / 6), 60), (180, adaptiveThreshold, 255))
dst = cv2.bitwise_and(gray, gray, mask=mask)
cv2.imwrite('colors_mask.bmp', dst)
return dst
(C#)private void ColoredMask(Mat src, Mat dst, double threshold = -1)
{
using (var gray = new Mat())
using (var color = new Mat())
using (var mask = new Mat())
using (var denoised = new Mat())
{
Cv2.MedianBlur(src, denoised, 3);
denoised.Save("colors_denoised.bmp");
Cv2.CvtColor(denoised, gray, ColorConversionCodes.BGR2GRAY);
gray.Save("colors_gray.bmp");
var adaptiveThreshold = threshold < 0 ? src.Mean()[0] : threshold;
Cv2.CvtColor(denoised, color, ColorConversionCodes.BGR2HLS);
Cv2.InRange(color, new Scalar(0, adaptiveThreshold / 6, 60), new Scalar(180, adaptiveThreshold, 255), mask);
Cv2.BitwiseAnd(gray, gray, dst, mask);
dst.Save("colors_mask.bmp");
}
}
:
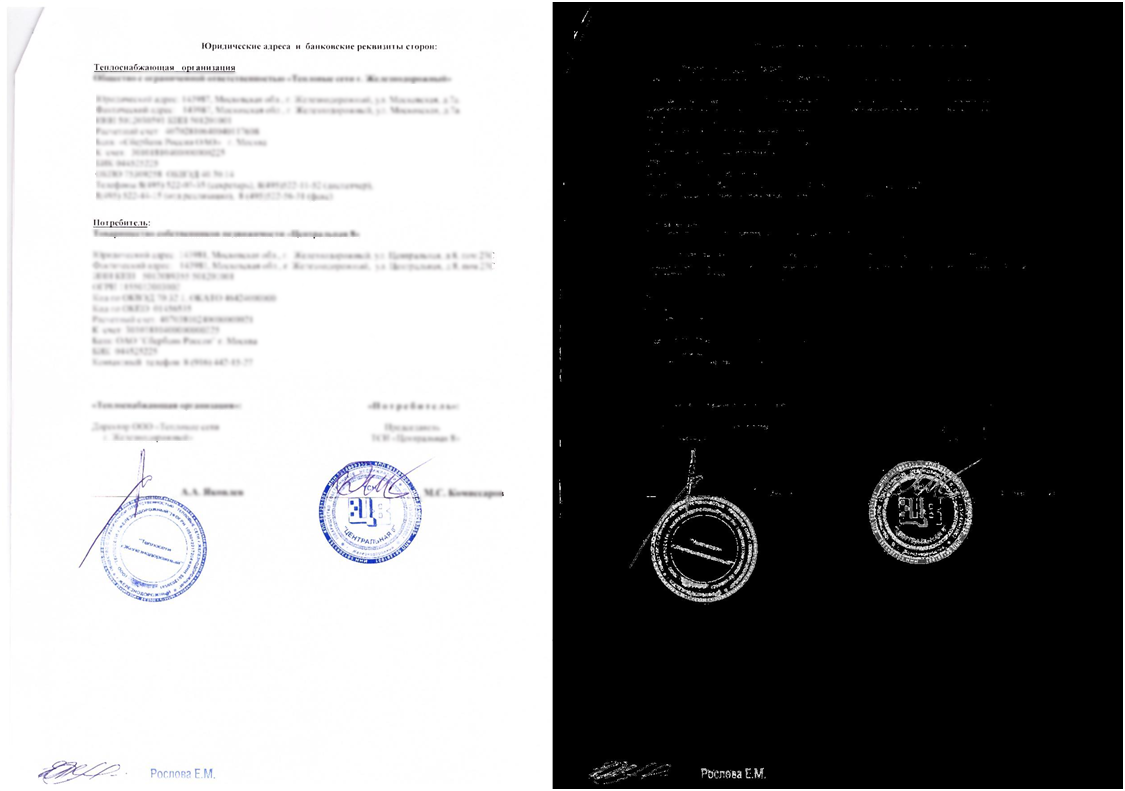
, HLS OpenCV 0 360, 0 180. â uint8/uchar/byte, 0 255, 360 2.
, , ? , , â â.
, . , ( ) , , â . , .
, , . OpenCV , , . , , .
(Python)
circles = cv2.HoughCircles(mask, cv2.HOUGH_GRADIENT, 1, 20, param1, param2, minRadius, maxRadius)
circles = np.uint16(np.around(circles))
cimg = cv2.cvtColor(img,cv2.COLOR_GRAY2BGR)
for i in circles[0,:]:
cv2.circle(cimg,(i[0],i[1]),i[2],(165,25,165),2)
(C#)
var houghCircles = Cv2.HoughCircles(morphed, HoughMethods.Gradient, 1, max, cannyEdgeThreshold, houghThreshold, min, max);
foreach (var circle in houghCircles)
Cv2.Circle(morphed, circle.Center, (int)circle.Radius, new Scalar(165, 25, 165), 2);
:
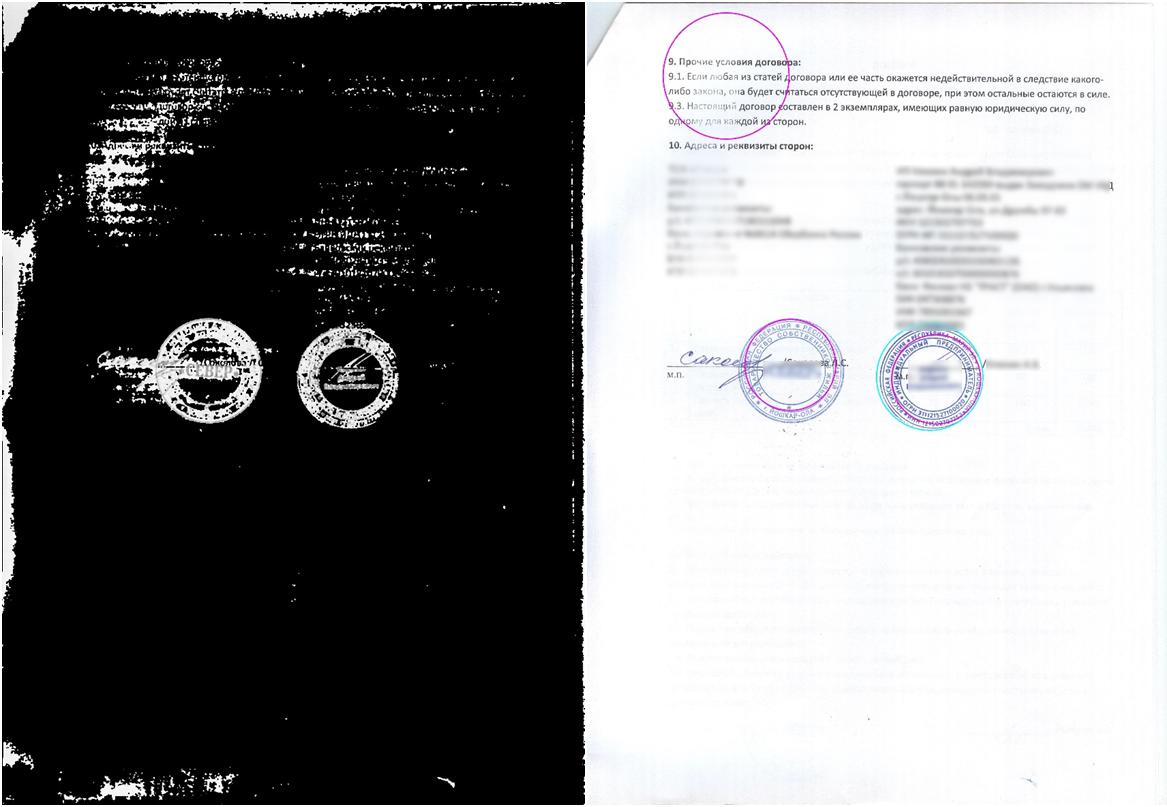
, , . , , . , , , . (- ââ - , - ).
- . .
, , . ââ , , . â , .
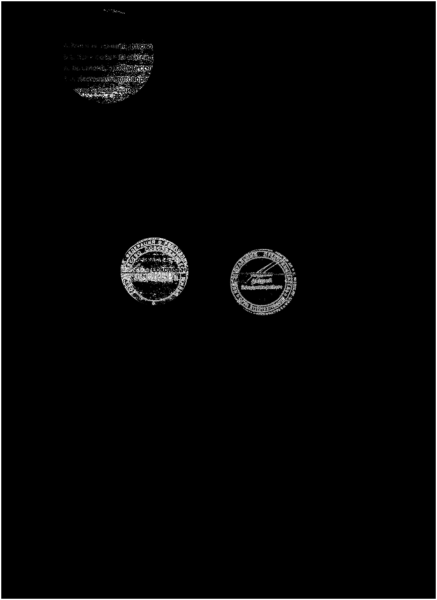
, , , . . , . ââ .
(Python)def equals(first, second, epsilon):
diff = cv2.subtract(first, second)
nonZero = cv2.countNonZero(diff)
area = first.size * epsilon
return nonZero <= area
for i in circles[0, :]:
empty = np.zeros((256, 256, 1), dtype="uint8")
cv2.circle(empty, (i[0], i[1]), i[2], (255, 255, 255), -1)
crop = img * (empty.astype(img.dtype))
cv2.imwrite('crop.bmp', crop)
if not equals(crop, empty, threshold):
result.append(i)
(C#)foreach (var circle in circles)
{
var x = (int) circle.Center.X;
var y = (int) circle.Center.Y;
var radius = (int) Math.Floor(circle.Radius);
using (var empty = Mat.Zeros(src.Rows, src.Cols, MatType.CV_8UC1))
using (var mask = empty.ToMat())
using (var crop = new Mat())
{
Cv2.Circle(mask, x, y, radius, Scalar.White, -1);
src.CopyTo(crop, mask);
crop.Save("crop.bmp");
if (!MatEquals(crop, empty, threshold))
result.Add(circle);
}
}
private bool MatEquals(Mat a, Mat b, double epsilon = 0.0)
{
var notEquals = a.NotEquals(b);
var nonZero = Cv2.CountNonZero(notEquals);
var area = a.Rows * epsilon * a.Cols;
return nonZero <= area;
}
. , , , - ?
, â , â (minor) (major) ( , , ).
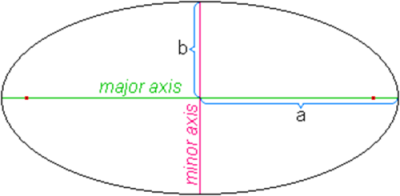
: , , , .
() , , voila â .
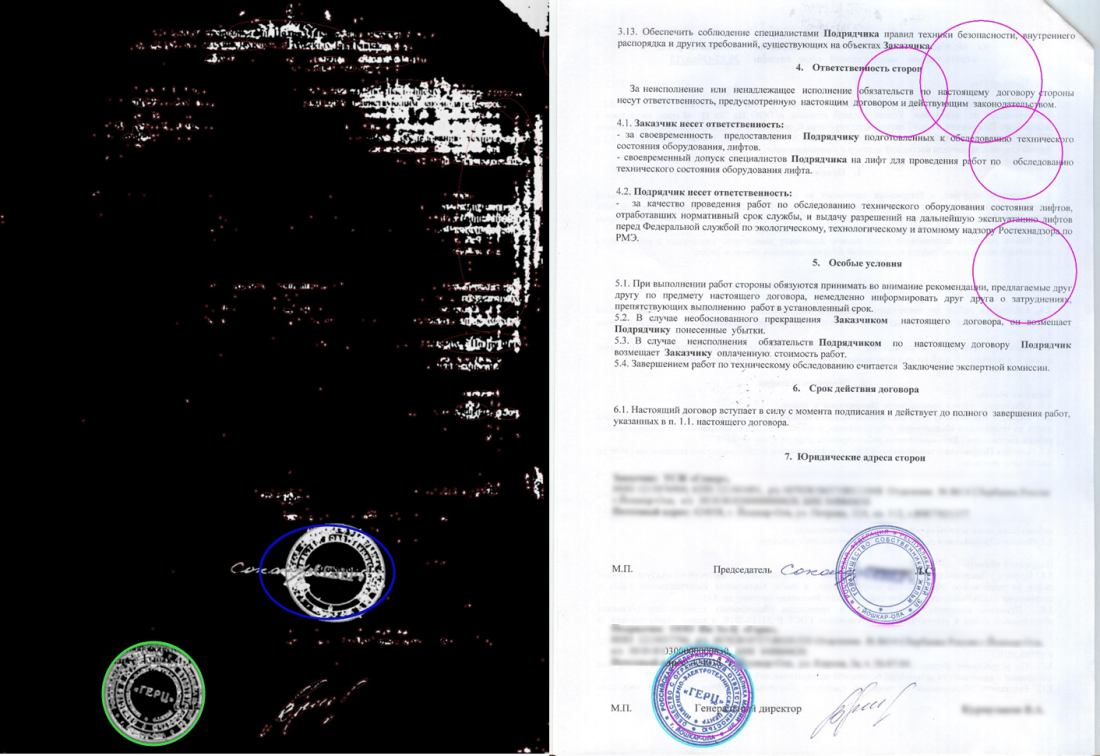
â , â , â , â , .
(Python)segments = []
contours, hierarchy = cv2.findContours(img, cv2.RETR_EXTERNAL, cv2.CHAIN_APPROX_SIMPLE)
for contour in contours:
if contour.shape[0] < 5:
continue
ellipse = cv2.fitEllipse(contour)
width = ellipse[1][0]
height = ellipse[1][1]
minor = min(width, height)
major = max(width, height)
if minor / 2 > minorMin and major / 2 < majorMax:
r1 = math.fabs(1 - math.fabs(major - minor) / max(minor, major))
cv2.ellipse(src, ellipse, (255, 0, 0), 3)
if r1 > roundness:
segments.append((ellipse[0], major / 2))
cv2.ellipse(src, ellipse, (0, 255, 0), 3)
else: cv2.ellipse(src, ellipse, (0, 0, 255), 1)
cv2.imwrite('test_res.bmp', src)
(Python)def distance(p1, p2):
return math.sqrt(math.pow(p2[0] - p1[0], 2) + math.pow(p2[1] - p1[1], 2))
def isNested(inner, outer, epsilon):
distance = distance(inner[0], outer[0])
radius = outer[1] * epsilon
return distance < radius and inner[1] < radius - distance
nested = []
for i in inner:
for o in outer:
if (isNested(i, o, 1.3)):
if (distance(i[0], i[1]) < 30 and i[1] / o[1] > 0.75):
nested.append(i)
else: nested.append(o)
(C#)Cv2.FindContours(src, out var contours, hierarchy, RetrievalModes.External, ContourApproximationModes.ApproxSimple);
foreach (var contour in contours)
{
if (contour.Height < 5)
continue;
var ellipse = Cv2.FitEllipse(contour);
var minor = Math.Min(ellipse.Size.Width, ellipse.Size.Height);
var major = Math.Max(ellipse.Size.Width, ellipse.Size.Height);
if (minor / 2 > minorSize && major / 2 < majorSize)
{
var r1 = Math.Abs(1 - Math.Abs(major - minor) / Math.Max(minor, major));
if (r1 > roundness)
{
var circle = new CircleSegment(ellipse.Center, major / 2);
segments.Add(circle);
}
}
contour.Dispose();
}
}
(C#)private bool IsCirclesNested(CircleSegment inner, CircleSegment outer, double epsilon)
{
var distance = inner.Center.DistanceTo(outer.Center);
var secondRadius = outer.Radius * epsilon;
return distance < secondRadius && inner.Radius < secondRadius - distance;
}
var nested = new List<CircleSegment>();
foreach (var i in inner)
foreach (var o in outer)
{
if (IsCirclesNested(i, o, 1.3))
{
if (i.Center.DistanceTo(o.Center) < 30 &&
i.Radius / o.Radius > 0.75)
nested.Add(i);
else nested.Add(o);
}
}
return outer.Union(inner).Except(nested).ToList();
, , , . . â , .
â â, , - . â â? ââ . ? , - . :
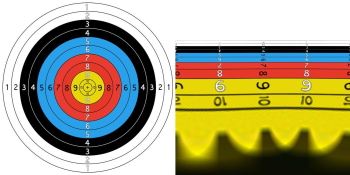
, , : , , . :
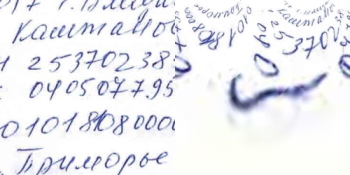
â X Y , X, . , , , .
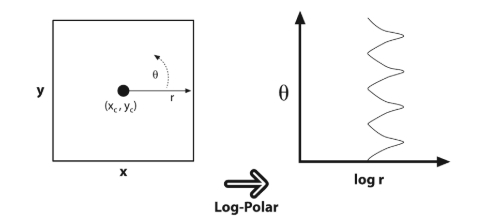
â , , ( ). , , ( , , , ).
, , . ( ) 90 .
(Python)maxRadius = math.sqrt(math.pow(src.shape[0], 2) + math.pow(src.shape[1], 2)) / 2
magnitude = src.shape[0] / math.log(maxRadius)
center = (src.shape[0] / 2, src.shape[1] / 2)
polar = cv2.logPolar(src, center, magnitude, cv2.INTER_AREA)
(C#)var maxRadius = Math.Sqrt(Math.Pow(stampImage.Width, 2) + Math.Pow(stampImage.Height, 2)) / 2;
var magnitude = stampImage.Width / Math.Log(maxRadius);
var center = new Point2f(stampImage.Width / 2, stampImage.Height / 2);
Cv2.LogPolar(stampImage, cartesianImage, center, magnitude, InterpolationFlags.Area);
, , OpenCVâ . , , , , OpenCV. , .
:
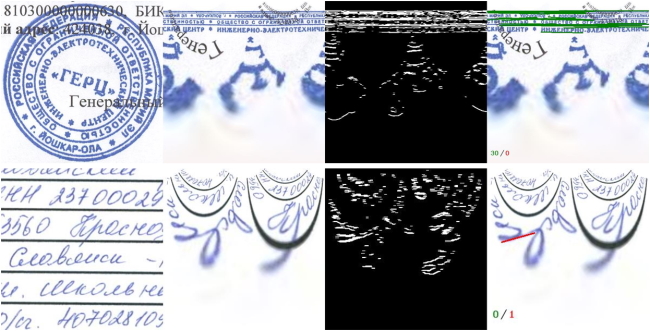
, , 90 , .
(Python)
sobel = cv2.Sobel(polar, cv2.CV_16S, 1, 0)
kernel = cv2.getStructuringElement(shape=cv2.MORPH_RECT, ksize=(1, 5))
img = cv2.convertScaleAbs(sobel)
img = cv2.cvtColor(img, cv2.COLOR_BGR2GRAY)
img = cv2.threshold(img, 0, 255, cv2.THRESH_BINARY + cv2.THRESH_OTSU)[1]
img = cv2.morphologyEx(img, cv2.MORPH_OPEN, kernel)
img = cv2.morphologyEx(img, cv2.MORPH_CLOSE, kernel)
def is_vertical(img_src, line):
tolerance = 10
coords = line[0]
angle = math.atan2(coords[3] - coords[1], coords[2] - coords[0]) * 180.0 / math.pi
edge = img_src.shape[0] * 0.66
out_of_bounds = coords[0] < edge and coords[2] < edge
return math.fabs(90 - math.fabs(angle)) <= tolerance and not out_of_bounds
lines = cv2.HoughLinesP(img, 1, math.pi / 180, 15, img.shape[0] / 5, 10)
vertical = [line for line in lines if is_vertical(img, line)]
correct_lines = len(vertical)
(C#)
using (var sobel = new Mat())
using (var kernel = Cv2.GetStructuringElement(MorphShapes.Rect, new Size(1, 5)))
{
Cv2.Sobel(img, sobel, MatType.CV_16S, 1, 0);
Cv2.ConvertScaleAbs(sobel, img);
Cv2.Threshold(img, img, 0, 255, ThresholdTypes.Binary | ThresholdTypes.Otsu);
Cv2.MorphologyEx(img, img, MorphTypes.Open, kernel);
Cv2.MorphologyEx(img, img, MorphTypes.Close, kernel);
}
bool AlmostVerticalLine(LineSegmentPoint line)
{
const int tolerance = 10;
var angle = Math.Atan2(line.P2.Y - line.P1.Y, line.P2.X - line.P1.X) * 180.0 / Math.PI;
var edge = edges.Width * 0.66;
var outOfBounds = line.P1.X < edge && line.P2.X < edge;
return Math.Abs(90 - Math.Abs(angle)) <= tolerance && !outOfBounds;
}
var lines = Cv2.HoughLinesP(img, 1, Math.PI / 180, 15, img.Width / 5, 10);
var correctLinesCount = lines.Count(AlmostVerticalLine);
, 85%. :
, â â ?â. :
, , , . OpenCV , . U-Net, OpenCV , .