El aprendizaje profundo a veces parece pura magia, ¡especialmente cuando la computadora aprende a hacer algo realmente creativo, por ejemplo, para pintar cuadros! La tecnología utilizada para esto se llama GAN, una red generativa competitiva, y en este artículo veremos cómo se organizan dichas redes y cómo capacitarlas para generar imágenes usando Azure Machine Learning.
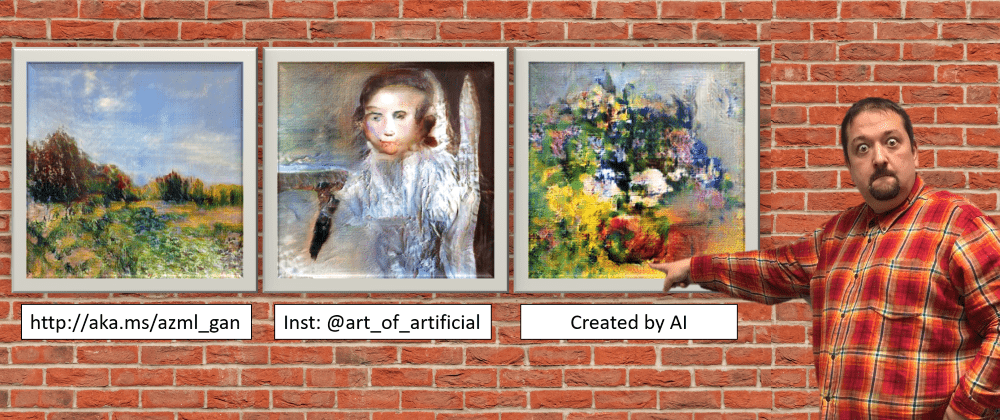
Esta publicación es parte de la iniciativa AI April . Todos los días de abril, mis colegas de Microsoft escriben artículos interesantes sobre inteligencia artificial y aprendizaje automático. Mire el calendario : de repente encontrará otros temas de interés para usted. Los artículos son principalmente en inglés.
Azure ML VS Code ), , Azure ML. , "" MNIST. Azure ML — , , :
WikiArt. , , , WikiArt Retriever, WikiArt Dataset GANGogh.
, dataset
. , , :
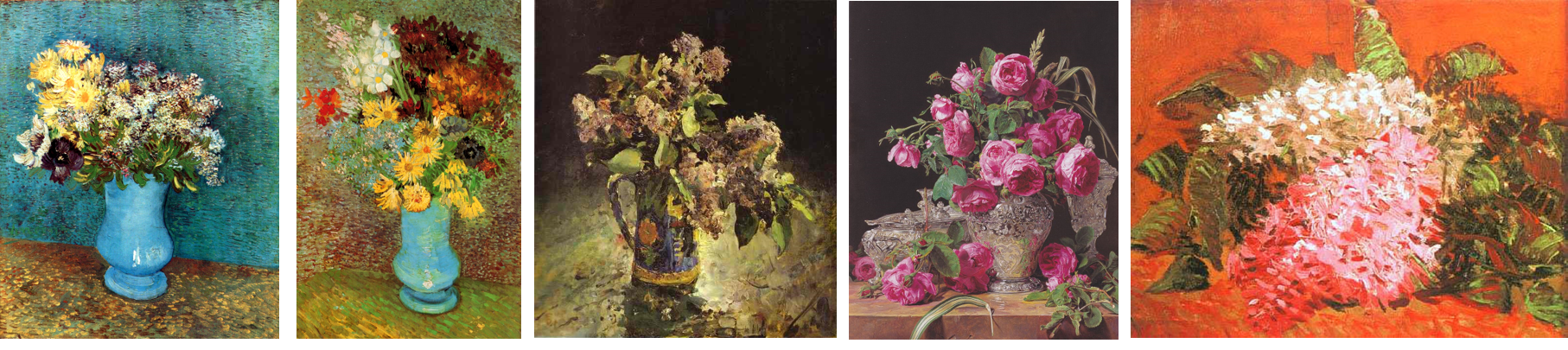
, , ( , ), (, ) .
-
- (GAN).
GAN keragan Keras, .
GAN :
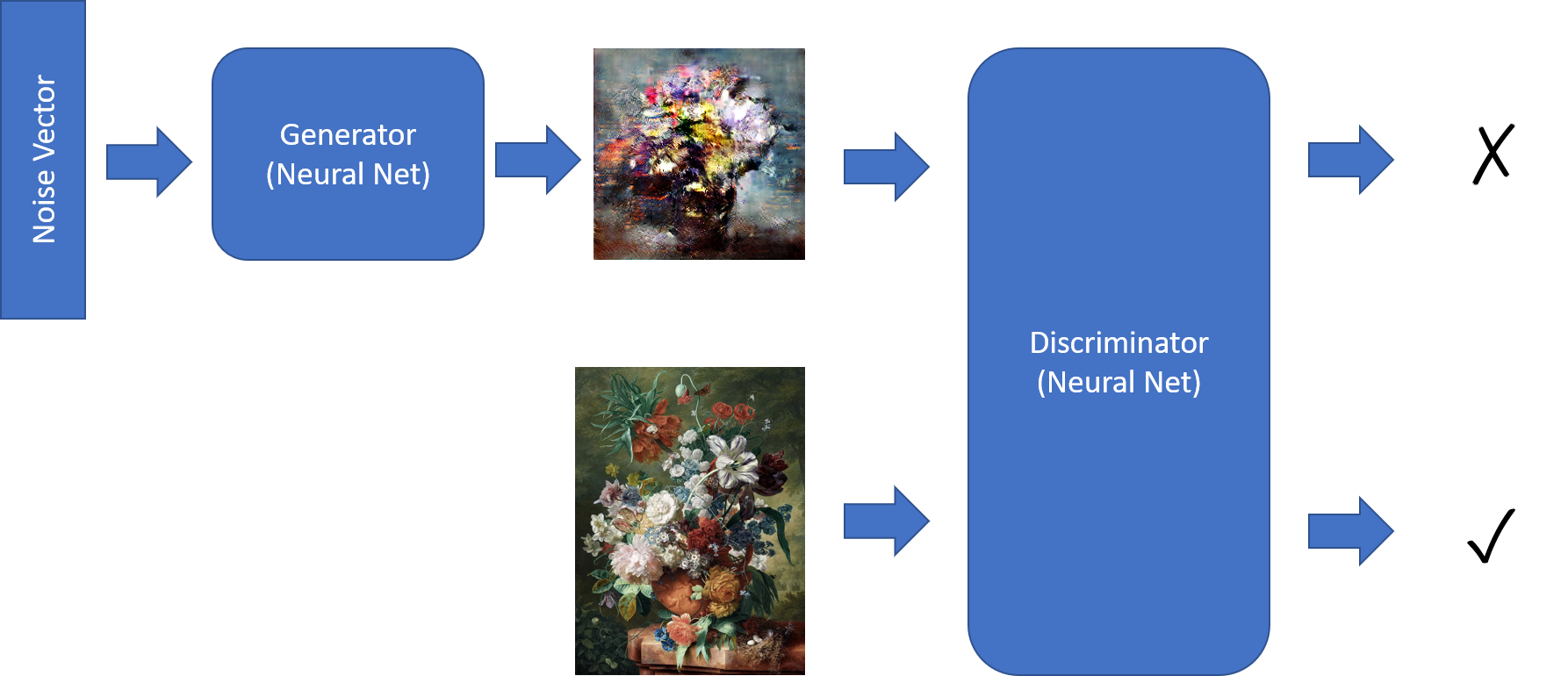
GAN :
- :
noise = np.random.normal(0, 1, (batch_size, latent_dim))
gen_imgs = generator.predict(noise)
imgs = get_batch(batch_size)
- .
ones
zeros
:
d_loss_r = discriminator.train_on_batch(imgs, ones)
d_loss_f = discriminator.train_on_batch(gen_imgs, zeros)
d_loss = np.add(d_loss_r , d_loss_f)*0.5
- , , :
g_loss = combined.train_on_batch(noise, ones)
, — :
discriminator = create_discriminator()
generator = create_generator()
discriminator.compile(loss='binary_crossentropy',optimizer=optimizer,
metrics=['accuracy'])
discriminator.trainable = False
z = keras.models.Input(shape=(latent_dim,))
img = generator(z)
valid = discriminator(img)
combined = keras.models.Model(z, valid)
combined.compile(loss='binary_crossentropy', optimizer=optimizer)
, (CNN). 64x64 :
discriminator = Sequential()
for x in [16,32,64]:
discriminator.add(Conv2D(x, (3,3), strides=1, padding="same"))
discriminator.add(AveragePooling2D())
discriminator.addBatchNormalization(momentum=0.8))
discriminator.add(LeakyReLU(alpha=0.2))
discriminator.add(Dropout(0.3))
discriminator.add(Flatten())
discriminator.add(Dense(1, activation='sigmoid'))
3 :
- 64x64x3 16- , (
AveragePooling2D
) 32x32x16. - 32x32x16 16x16x32
- 8x8x64.
, ( — Dense
).
. , , — latent_dim=100
. , , 100..
— 100 . . UpSampling2D
, :
generator = Sequential()
generator.add(Dense(8 * 8 * 2 * size, activation="relu",
input_dim=latent_dim))
generator.add(Reshape((8, 8, 2 * size)))
for x in [64;32;16]:
generator.add(UpSampling2D())
generator.add(Conv2D(x, kernel_size=(3,3),strides=1,padding="same"))
generator.add(BatchNormalization(momentum=0.8))
generator.add(Activation("relu"))
generator.add(Conv2D(3, kernel_size=3, padding="same"))
generator.add(Activation("tanh"))
64x64x3, . tanh
[-1;1] — , . , , ImageDataset
, .
Azure ML
- , Azure ML !
, , . Azure ML , (accuracy) (loss). run.log
, , Azure ML.
, , ( ) . , , .. - , .
, Azure ML , . log_image
, numpy-, , matplotlib
. , , . callbk
, keragan
:
def callbk(tr):
if tr.gan.epoch % 20 == 0:
res = tr.gan.sample_images(n=3)
fig,ax = plt.subplots(1,len(res))
for i,v in enumerate(res):
ax[i].imshow(v[0])
run.log_image("Sample",plot=plt)
:
gan = keragan.DCGAN(args)
imsrc = keragan.ImageDataset(args)
imsrc.load()
train = keragan.GANTrainer(image_dataset=imsrc,gan=gan,args=args)
train.train(callbk)
, keragan
, args
, , , , learning rate ..
Azure ML VS Code, , SDK, Azure ML. submit_gan.ipynb, :
- :
ws = Workspace.from_config()
- :
cluster = ComputeTarget(workspace=ws, name='My Cluster')
. GPU, [NC6][AzureVMNC]. - :
ds.upload(...)
.
, , :
exp = Experiment(workspace=ws, name='KeraGAN')
script_params = {
'--path': ws.get_default_datastore(),
'--dataset' : 'faces',
'--model_path' : './outputs/models',
'--samples_path' : './outputs/samples',
'--batch_size' : 32,
'--size' : 512,
'--learning_rate': 0.0001,
'--epochs' : 10000
}
est = TensorFlow(source_directory='.',
script_params=script_params,
compute_target=cluster,
entry_script='train_gan.py',
use_gpu = True,
conda_packages=['keras','tensorflow','opencv','tqdm','matplotlib'],
pip_packages=['git+https://github.com/shwars/keragan@v0.0.1']
run = exp.submit(est)
model_path=./outputs/models
samples_path=./outputs/samples
, ( ) outputs
. , , Azure ML.
Estimator
, GPU, Tensorflow
. Estimator
, " " . Estimator
- .
— , keragan
GitHub. PyPI pip
-, , GitHub , commit ID. , PyPI.
, Azure ML Portal:
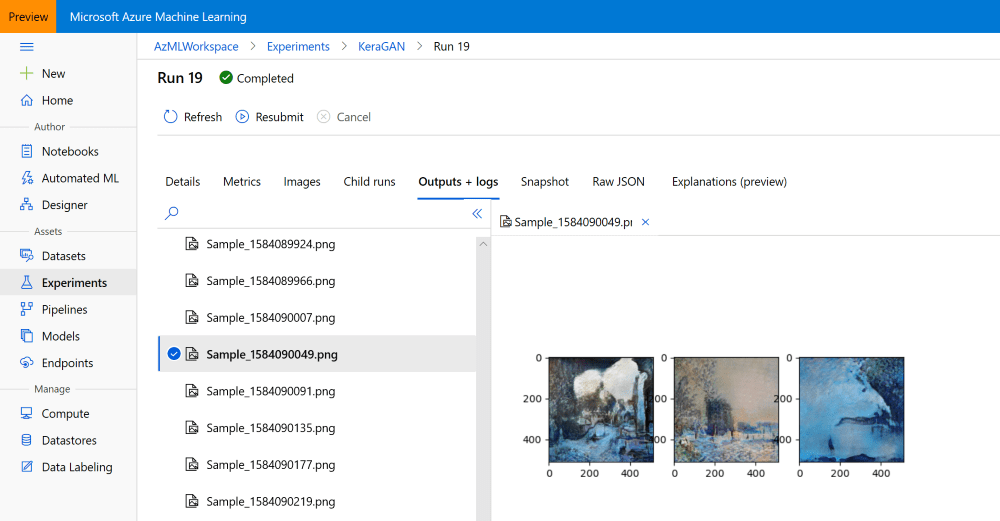
GAN , . -, learning rate: , — . .
:
--size
, . (64 128) , ( 1024) . 1024 , , progressive growing--learning_rate
. , .--dateset
. , , Azure ML datastore, .
, for
, . , . .
, , . outputs/models
, — outputs/samples
. Azure ML Portal :
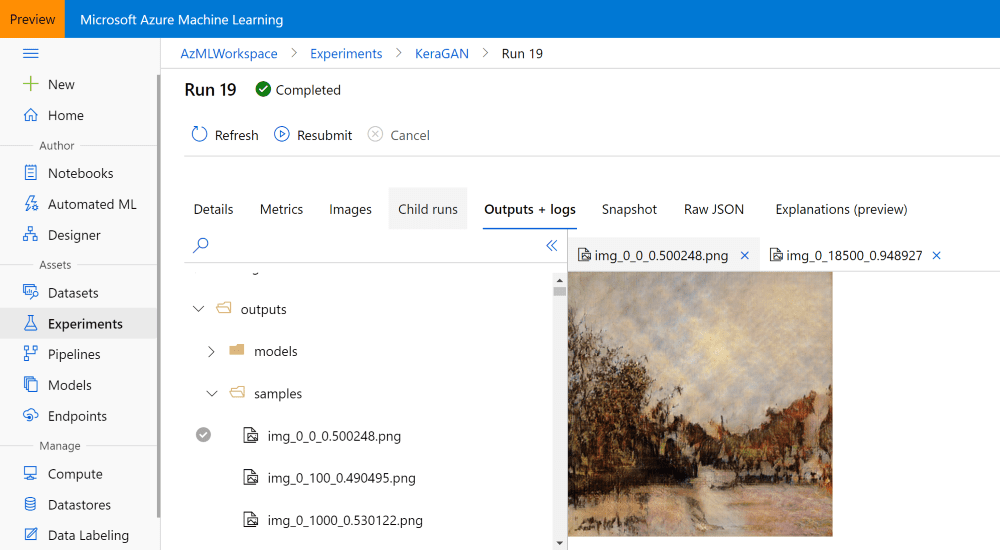
, , . run
, , , ( ):
run.download_files(prefix='outputs/samples')
outputs/samples
, .
run
( , ), , run id, :
run = Run(experiment=exp,run_id='KeraGAN_1584082108_356cf603')
. , , . , ( gen_
):
fnames = run.get_file_names()
fnames = filter(lambda x : x.startswith('outputs/models/gen_'),fnames)
: outputs/models/gen_0.h5
, outputs/models/gen_100.h5
.. :
no = max(map(lambda x: int(x[19:x.find('.')]), fnames))
fname = 'outputs/models/gen_{}.h5'.format(no)
fname_wout_path = fname[fname.rfind('/')+1:]
run.download_file(fname)
, fname_wout_path
.
, Keras, , , , :
model = keras.models.load_model(fname_wout_path)
latent_dim=model.layers[0].input.shape[1].value
res = model.predict(np.random.normal(0,1,(10,latent_dim)))
, [-1,1], [0,1], matplotlib
:
res = (res+1.0)/2
fig,ax = plt.subplots(1,10,figsize=(15,10))
for i in range(10):
ax[i].imshow(res[i])
:

, :
( ) , — @art_of_artificial, .
, - . , , . , :
- , Azure. , . , — — . ? ...
keragan, , DCGAN, Maxime Ellerbach, GANGogh. GAN Keras .
Azure ML