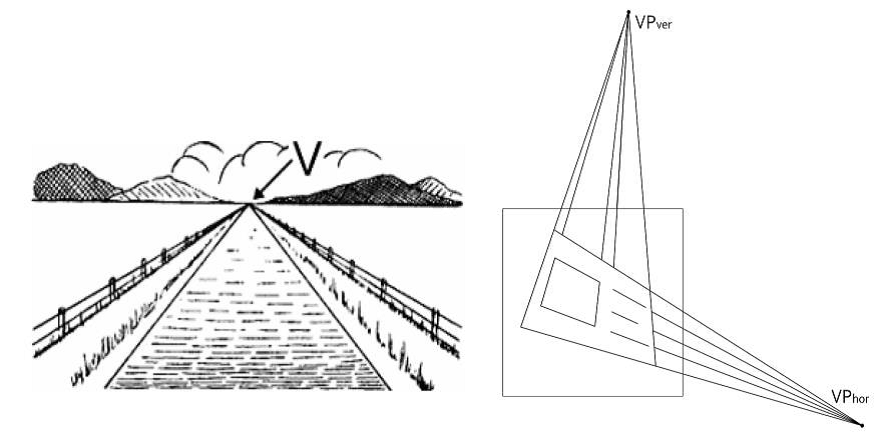
Si bien se enseñan docenas e incluso cientos de arquitecturas comprobadas de redes neuronales artificiales (ANN) en el mundo del reconocimiento de objetos, calentando el planeta con poderosas tarjetas de video y creando una "panacea" para todas las tareas de visión por computadora, estamos firmemente en el camino de la investigación en Smart Engines, ofreciendo nuevas arquitecturas ANN efectivas para resolver problemas específicos Hoy hablaremos de HafNet, una nueva forma de buscar puntos de fuga en las imágenes.
Transformación Hough y su rápida implementación.
. , , , , . ( ). () : , , , .. , , , , , .
(xi,yi). , yi=xia+b a b. b=-xia+yi ab, , (xi,yi) . : , , , . : , . ( – , ).
, , , – .
, . .

, , : – O(n3), n – .
() – , , () . O(n2 log(n)), . , , , [5]. , : : « » ( H1), « » ( H2), « » ( H3) « » ( H4). , H12 H34 , .
( , ). , , . .
. , , - , : ( , , ), – . , . . , - . , H12 , . , , H34 , . , , H12 , , , . ( , H12 ).

( ). , ( – ).
, , : ? , … , !
HoughNet
, , - (, – , – ). «» ( , ). «» . «» , , ?
, ( HoughNet), , - . , , – , , . , ( [1]).
: «» , «» . .
. MIDV-500 [6]. , . 50 . ( , 30 ) , – .
, , ICDAR 2011 dewarping contest dataset ( 100 - , ) .

«» ( ), state-of-the-art [7] [8].
[1] Sheshkus A. et al. HoughNet: neural network architecture for vanishing points detection // 2019 International Conference on Document Analysis and Recognition (ICDAR). – 2020. doi: 10.1109/ICDAR.2019.00140.
[2] . ., . ., . . // . – 2014. – . 64. – №. 3. – . 25-34.
[3] .. : . … . . .-. . – ., 2019. – 24 .
[4] [ ]: . . – : https://ru.wikipedia.org/wiki/_/ ( : 13.03.2020).
[5] Nikolaev D. P., Karpenko S. M., Nikolaev I. P., Nikolayev P. P. Hough Transform: Underestimated Tool in the Computer Vision Field // 22st European Conference on Modelling and Simulation, ECMS 2008. – Nicosia, Cyprys, 2008. – P. 238–243.
[6] Arlazarov V. V. et al. MIDV-500: a dataset for identity document analysis and recognition on mobile devices in video stream // . – 2019. – . 43. – №. 5.
[7] Y. Takezawa, M. Hasegawa, and S. Tabbone, “Cameracaptured document image perspective distortion correction using vanishing point detection based on radon transform,” in Pattern Recognition (ICPR), 2016 23rd International Conference on. IEEE, 2016, pp. 3968–3974.
[8] Y. Takezawa, M. Hasegawa, and S. Tabbone, “Robust perspective rectification of camera-captured document images,” in Document Analysis and Recognition (ICDAR), 2017 14th IAPR International Conference on, vol. 6. IEEE, 2017, pp. 27–32.