¿Tenía algo que le gustó en la tienda en línea, pero no quiere comprarlo sin probarlo? Por supuesto, en algunas tiendas existe la oportunidad de probarse la ropa después de ordenar antes del pago. Sin embargo, según las estadísticas, la proporción de pedidos en línea en tiendas en línea de ropa y zapatos está creciendo cada año, pero la proporción de devoluciones también está creciendo, asciende a 50-70%; estos son enormes costos logísticos que pueden reducirse significativamente usando el probador en línea. Imagine que carga su foto, elige ropa y se transfiere a su imagen. Los probadores virtuales para zapatos ya existen, funcionan con bastante éxito. Hace algún tiempo estábamos interesados en este tema, ¿qué pasa con la ropa? Tales trabajos también existen, pero mucho menos exitosos; en muchos de ellos, además del artículo, no se puede encontrar nadaUno solo puede soñar con un ejemplo de trabajo. Decidimos solucionar esto y admitir una de las redes en la biblioteca OpenCV. Lo que vino de esto se puede ver enMuestra virtual_try_on.py .
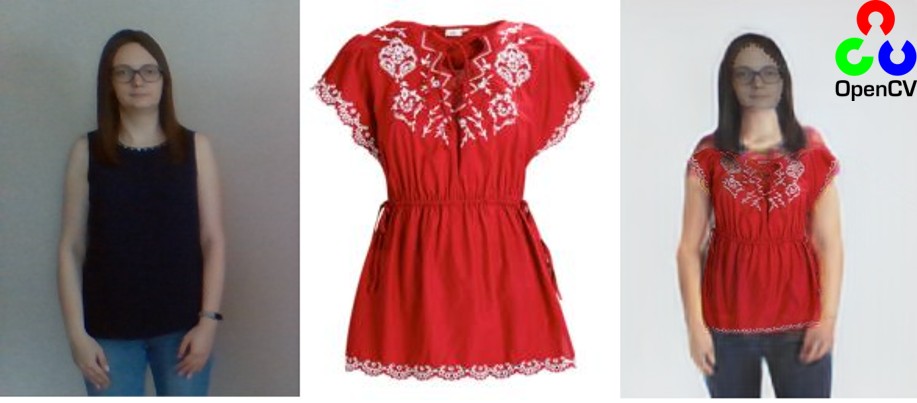
El resultado no es perfecto, pero en esta área se considera bastante bueno.
Si desea saber cómo funciona el probador virtual y qué dificultades encontramos al integrar el modelo en OpenCV, ¡bienvenido al gato!
2019 CP-VTON . CP-VTON , , (, ). 3D , 3D-. . github. CP-VTON , , .
.
CP-VTON : GMM (Geometric Matching Module) — TOM (Try-On Module) — .
GMM , TOM — , — , — , — GMM, — ground truth ( ), — ground truth ( , ). , . VITON, . , , , , (, , ). . , GMM , . TOM , .
. , . OpenPose. . LIP_JPPNet. OpenCV () sample human_parsing.py.
, — , : , , . , .

.
GMM . , , . . . , . .

TOM . Unet. , . Unet , . , Unet . , . Upsample . (encoder) VGG-19. . Unet . , .
— .

Try-On — . . , , . perceptual loss. VGG , . VGG — , .
OpenCV
. json , OpenPose Caffe, LIP.
, , .
python3 virtual_try_on.py -i person_img.jpg -c cloth.jpg
OpenCV PIL. . LIP , . CP-VTON . , :
shape = (segm > 0).astype(np.float32)
head = (segm == 1).astype(np.float32) + \
(segm == 2).astype(np.float32) + \
(segm == 4).astype(np.float32) + \
(segm == 13).astype(np.float32)
cloth = (segm == 5).astype(np.float32) + \
(segm == 6).astype(np.float32) + \
(segm == 7).astype(np.float32)
, PIL , . human_colormap.mat. ? Matlab . , scipy . () .
– . 16 , .
mask = mask.resize((width // 16, height // 16), Image.BILINEAR)
mask = mask.resize((width, height), Image.BILINEAR)
. . , OpenCV. PIL resize cv.resize .

, PIL resize, — cv.resize.
, ? .

, PIL resize, — cv.resize.
, . ? , . , – . , bilinear resize bilinear, area. scale factor, 33 = 16 * 2 + 1, OpenCV – 3. , , . . . , . . :
PILclass BilinearFilter(object):
"""
PIL bilinear resize implementation
image = image.resize((image_width // 16, image_height // 16), Image.BILINEAR)
"""
def _precompute_coeffs(self, inSize, outSize):
filterscale = max(1.0, inSize / outSize)
ksize = int(np.ceil(filterscale)) * 2 + 1
kk = np.zeros(shape=(outSize * ksize, ), dtype=np.float32)
bounds = np.empty(shape=(outSize * 2, ), dtype=np.int32)
centers = (np.arange(outSize) + 0.5) * filterscale + 0.5
bounds[::2] = np.where(centers - filterscale < 0, 0, centers - filterscale)
bounds[1::2] = np.where(centers + filterscale > inSize, inSize, centers + filterscale) - bounds[::2]
xmins = bounds[::2] - centers + 1
points = np.array([np.arange(row) + xmins[i] for i, row in enumerate(bounds[1::2])]) / filterscale
for xx in range(0, outSize):
point = points[xx]
bilinear = np.where(point < 1.0, 1.0 - abs(point), 0.0)
ww = np.sum(bilinear)
kk[xx * ksize : xx * ksize + bilinear.size] = np.where(ww == 0.0, bilinear, bilinear / ww)
return bounds, kk, ksize
def _resample_horizontal(self, out, img, ksize, bounds, kk):
for yy in range(0, out.shape[0]):
for xx in range(0, out.shape[1]):
xmin = bounds[xx * 2 + 0]
xmax = bounds[xx * 2 + 1]
k = kk[xx * ksize : xx * ksize + xmax]
out[yy, xx] = np.round(np.sum(img[yy, xmin : xmin + xmax] * k))
def _resample_vertical(self, out, img, ksize, bounds, kk):
for yy in range(0, out.shape[0]):
ymin = bounds[yy * 2 + 0]
ymax = bounds[yy * 2 + 1]
k = kk[yy * ksize: yy * ksize + ymax]
out[yy] = np.round(np.sum(img[ymin : ymin + ymax, 0:out.shape[1]] * k[:, np.newaxis], axis=0))
def imaging_resample(self, img, xsize, ysize):
height, width, *args = img.shape
bounds_horiz, kk_horiz, ksize_horiz = self._precompute_coeffs(width, xsize)
bounds_vert, kk_vert, ksize_vert = self._precompute_coeffs(height, ysize)
out_hor = np.empty((img.shape[0], xsize), dtype=np.uint8)
self._resample_horizontal(out_hor, img, ksize_horiz, bounds_horiz, kk_horiz)
out = np.empty((ysize, xsize), dtype=np.uint8)
self._resample_vertical(out, out_hor, ksize_vert, bounds_vert, kk_vert)
return out
4 , , OpenCV. . , . , . 256 192, . sample . , - , .
P.S. 2020 OpenCV 20-. . !