Las redes neuronales son bastante populares. Su principal ventaja es que pueden generalizar datos bastante complejos en los que otros algoritmos muestran baja calidad. Pero, ¿qué pasa si la calidad de la red neuronal sigue siendo insatisfactoria?
Y aquí los conjuntos vienen al rescate ...
¿Qué son los conjuntos?
Un conjunto de algoritmos de aprendizaje automático es el uso de varios modelos (no necesariamente diferentes) en lugar de uno. Es decir, primero entrenamos cada modelo y luego combinamos sus predicciones. Resulta que nuestros modelos juntos forman un modelo más complejo (en términos de capacidad de generalización: la capacidad de "comprender" los datos), que a menudo se denomina metamodelo . Muy a menudo, el metamodelo ya no está entrenado en nuestra muestra de datos inicial, sino en las predicciones de otros modelos. Parece tener en cuenta la experiencia de todos los modelos, y esto ayuda a reducir los errores.
Plan
- Primero miramos un modelo PyTorch simple y obtenemos su calidad.
- Luego ensamblaremos varios modelos usando Scikit-learn y aprenderemos cómo alcanzar un nivel superior
Un solo modelo
Por lo tanto, trabajaremos con el conjunto de datos Dígitos de Sklearn, ya que puede obtenerse simplemente en dos líneas de código:
from sklearn.datasets import load_digits
import numpy as np
import matplotlib.pyplot as plt
x, y = load_digits(n_class=10, return_X_y=True)
Veamos cómo se ven nuestros datos:
print(x.shape)
>>> (1797, 64)
print(np.unique(y))
>>> array([0, 1, 2, 3, 4, 5, 6, 7, 8, 9])
index = 0
print(y[index])
plt.imshow(x[index].reshape(8, 8), cmap="Greys")
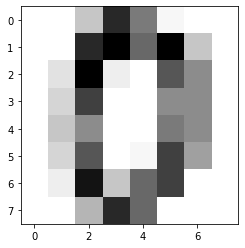
x[index]
>>> array([ 0., 0., 5., 13., 9., 1., 0., 0., 0., 0., 13., 15., 10.,
15., 5., 0., 0., 3., 15., 2., 0., 11., 8., 0., 0., 4.,
12., 0., 0., 8., 8., 0., 0., 5., 8., 0., 0., 9., 8.,
0., 0., 4., 11., 0., 1., 12., 7., 0., 0., 2., 14., 5.,
10., 12., 0., 0., 0., 0., 6., 13., 10., 0., 0., 0.])
Sí, los números toman valores del 0 al 15. Entonces, necesitamos normalización:
from sklearn.model_selection import train_test_split
from sklearn.preprocessing import StandardScaler
x_train, x_test, y_train, y_test = train_test_split(x, y, test_size=0.2,
shuffle=True, random_state=42)
print(x_train.shape)
>>> (1437, 64)
print(x_test.shape)
>>> (360, 64)
Usamos StandardScaler: transfiere todos los datos a los límites de -1 a 1. Lo entrenamos solo en la muestra de entrenamiento, para que no se adapte a la prueba, por lo que la puntuación del modelo después de la prueba estará más cerca de la puntuación en datos reales:
scaler = StandardScaler()
scaler.fit(x_train)
Y normalizamos nuestros datos:
x_train_scaled = scaler.transform(x_train)
x_test_scaled = scaler.transform(x_test)
Tiempo de la antorcha!
import torch
from torch.utils.data import TensorDataset, DataLoader
import torch.nn as nn
import torch.nn.functional as F
from torch.optim import Adam
Una red convolucional simple es suficiente para demostrar:
class SimpleCNN(nn.Module):
def __init__(self):
super(SimpleCNN, self).__init__()
self.conv1 = nn.Conv2d(in_channels=1, out_channels=3, kernel_size=5, stride=1, padding=2)
self.conv1_s = nn.Conv2d(in_channels=3, out_channels=3, kernel_size=5, stride=2, padding=2)
self.conv2 = nn.Conv2d(in_channels=3, out_channels=6, kernel_size=3, stride=1, padding=1)
self.conv2_s = nn.Conv2d(in_channels=6, out_channels=6, kernel_size=3, stride=2, padding=1)
self.conv3 = nn.Conv2d(in_channels=6, out_channels=10, kernel_size=3, stride=1, padding=1)
self.conv3_s = nn.Conv2d(in_channels=10, out_channels=10, kernel_size=3, stride=2, padding=1)
self.flatten = nn.Flatten()
self.fc1 = nn.Linear(10, 10)
def forward(self, x):
x = F.relu(self.conv1(x))
x = F.relu(self.conv1_s(x))
x = F.relu(self.conv2(x))
x = F.relu(self.conv2_s(x))
x = F.relu(self.conv3(x))
x = F.relu(self.conv3_s(x))
x = self.flatten(x)
x = self.fc1(x)
x = F.softmax(x)
return x
Defina algunos hiperparámetros:
batch_size = 64
learning_rate = 1e-3
epochs = 200
Primero, convierta los datos a los tensores con los que trabaja PyTorch:
x_train_tensor = torch.tensor(x_train_scaled.reshape(-1, 1, 8, 8).astype(np.float32))
x_test_tensor = torch.tensor(x_test_scaled.reshape(-1, 1, 8, 8).astype(np.float32))
y_train_tensor = torch.tensor(y_train.astype(np.long))
y_test_tensor = torch.tensor(y_test.astype(np.long))
PyTorch , — . — , X y ( ), DataLoader — , <X, y> 64 :
train_dataset = TensorDataset(x_train_tensor, y_train_tensor)
train_loader = DataLoader(train_dataset, batch_size=batch_size)
test_dataset = TensorDataset(x_test_tensor, y_test_tensor)
test_loader = DataLoader(test_dataset, batch_size=batch_size)
, :
simple_cnn = SimpleCNN().cuda()
criterion = nn.CrossEntropyLoss()
optimizer = Adam(simple_cnn.parameters(), lr=learning_rate)
:
for epoch in range(epochs):
simple_cnn.train()
train_samples_count = 0
true_train_samples_count = 0
running_loss = 0
for batch in train_loader:
x_data = batch[0].cuda()
y_data = batch[1].cuda()
y_pred = simple_cnn(x_data)
loss = criterion(y_pred, y_data)
optimizer.zero_grad()
loss.backward()
optimizer.step()
running_loss += loss.item()
y_pred = y_pred.argmax(dim=1, keepdim=False)
true_classified = (y_pred == y_data).sum().item()
true_train_samples_count += true_classified
train_samples_count += len(x_data)
train_accuracy = true_train_samples_count / train_samples_count
print(f"[{epoch}] train loss: {running_loss}, accuracy: {round(train_accuracy, 4)}")
simple_cnn.eval()
test_samples_count = 0
true_test_samples_count = 0
running_loss = 0
for batch in test_loader:
x_data = batch[0].cuda()
y_data = batch[1].cuda()
y_pred = simple_cnn(x_data)
loss = criterion(y_pred, y_data)
loss.backward()
running_loss += loss.item()
y_pred = y_pred.argmax(dim=1, keepdim=False)
true_classified = (y_pred == y_data).sum().item()
true_test_samples_count += true_classified
test_samples_count += len(x_data)
test_accuracy = true_test_samples_count / test_samples_count
print(f"[{epoch}] test loss: {running_loss}, accuracy: {round(test_accuracy, 4)}")
,- Torch' , . . 20 .
[180] train loss: 40.52328181266785, accuracy: 0.6966
[180] test loss: 10.813781499862671, accuracy: 0.6583
[181] train loss: 40.517325043678284, accuracy: 0.6966
[181] test loss: 10.811877608299255, accuracy: 0.6611
[182] train loss: 40.517088294029236, accuracy: 0.6966
[182] test loss: 10.814386487007141, accuracy: 0.6611
[183] train loss: 40.515315651893616, accuracy: 0.6966
[183] test loss: 10.812204122543335, accuracy: 0.6611
[184] train loss: 40.5108939409256, accuracy: 0.6966
[184] test loss: 10.808713555335999, accuracy: 0.6639
[185] train loss: 40.50885498523712, accuracy: 0.6966
[185] test loss: 10.80833113193512, accuracy: 0.6639
[186] train loss: 40.50892996788025, accuracy: 0.6966
[186] test loss: 10.809209108352661, accuracy: 0.6639
[187] train loss: 40.508036971092224, accuracy: 0.6966
[187] test loss: 10.806900978088379, accuracy: 0.6667
[188] train loss: 40.507275462150574, accuracy: 0.6966
[188] test loss: 10.79791784286499, accuracy: 0.6611
[189] train loss: 40.50368785858154, accuracy: 0.6966
[189] test loss: 10.799399137496948, accuracy: 0.6667
[190] train loss: 40.499858379364014, accuracy: 0.6966
[190] test loss: 10.795265793800354, accuracy: 0.6611
[191] train loss: 40.498780846595764, accuracy: 0.6966
[191] test loss: 10.796114206314087, accuracy: 0.6639
[192] train loss: 40.497228503227234, accuracy: 0.6966
[192] test loss: 10.790620803833008, accuracy: 0.6639
[193] train loss: 40.44325613975525, accuracy: 0.6973
[193] test loss: 10.657087206840515, accuracy: 0.7
[194] train loss: 39.62049174308777, accuracy: 0.7495
[194] test loss: 10.483307123184204, accuracy: 0.7222
[195] train loss: 39.24516046047211, accuracy: 0.7613
[195] test loss: 10.462445378303528, accuracy: 0.7278
[196] train loss: 39.16947162151337, accuracy: 0.762
[196] test loss: 10.488057255744934, accuracy: 0.7222
[197] train loss: 39.196797251701355, accuracy: 0.7634
[197] test loss: 10.502906918525696, accuracy: 0.7222
[198] train loss: 39.395434617996216, accuracy: 0.7537
[198] test loss: 10.354896545410156, accuracy: 0.7472
[199] train loss: 39.331292152404785, accuracy: 0.7509
[199] test loss: 10.367400050163269, accuracy: 0.7389
, : .
, : 74% . Not great, not terrible.
!
Bagging. : , , . : "" , - . .
sklearn ensembles
, . — , BaggingClassifier
:
import sklearn
from sklearn.ensemble import BaggingClassifier
. sklearn, — , sklearn.base.BaseEstimator
. , . fit
( ), predict_proba
, ( , ), predict
( ), .
, "" .
. , , .
-, ( 2 — get_params
set_params
), , . , net_type
, __init__
net_type
.
-, , , ( sklearn , ) copy
, deepcopy
. , , () , , .
class PytorchModel(sklearn.base.BaseEstimator):
def __init__(self, net_type, net_params, optim_type, optim_params, loss_fn,
input_shape, batch_size=32, accuracy_tol=0.02, tol_epochs=10,
cuda=True):
self.net_type = net_type
self.net_params = net_params
self.optim_type = optim_type
self.optim_params = optim_params
self.loss_fn = loss_fn
self.input_shape = input_shape
self.batch_size = batch_size
self.accuracy_tol = accuracy_tol
self.tol_epochs = tol_epochs
self.cuda = cuda
: fit
. , — , Loss, . : , ? . , 200 ( ). , . — , accuracy . , accuracy . , (tol_epochs
) ( accuracy , accuracy_tol
).
def fit(self, X, y):
self.net = self.net_type(**self.net_params)
if self.cuda:
self.net = self.net.cuda()
self.optim = self.optim_type(self.net.parameters(), **self.optim_params)
uniq_classes = np.sort(np.unique(y))
self.classes_ = uniq_classes
X = X.reshape(-1, *self.input_shape)
x_tensor = torch.tensor(X.astype(np.float32))
y_tensor = torch.tensor(y.astype(np.long))
train_dataset = TensorDataset(x_tensor, y_tensor)
train_loader = DataLoader(train_dataset, batch_size=self.batch_size,
shuffle=True, drop_last=False)
last_accuracies = []
epoch = 0
keep_training = True
while keep_training:
self.net.train()
train_samples_count = 0
true_train_samples_count = 0
for batch in train_loader:
x_data, y_data = batch[0], batch[1]
if self.cuda:
x_data = x_data.cuda()
y_data = y_data.cuda()
y_pred = self.net(x_data)
loss = self.loss_fn(y_pred, y_data)
self.optim.zero_grad()
loss.backward()
self.optim.step()
y_pred = y_pred.argmax(dim=1, keepdim=False)
true_classified = (y_pred == y_data).sum().item()
true_train_samples_count += true_classified
train_samples_count += len(x_data)
train_accuracy = true_train_samples_count / train_samples_count
last_accuracies.append(train_accuracy)
if len(last_accuracies) > self.tol_epochs:
last_accuracies.pop(0)
if len(last_accuracies) == self.tol_epochs:
accuracy_difference = max(last_accuracies) - min(last_accuracies)
if accuracy_difference <= self.accuracy_tol:
keep_training = False
. — , Loss, - :
def predict_proba(self, X, y=None):
X = X.reshape(-1, *self.input_shape)
x_tensor = torch.tensor(X.astype(np.float32))
if y:
y_tensor = torch.tensor(y.astype(np.long))
else:
y_tensor = torch.zeros(len(X), dtype=torch.long)
test_dataset = TensorDataset(x_tensor, y_tensor)
test_loader = DataLoader(test_dataset, batch_size=self.batch_size,
shuffle=False, drop_last=False)
self.net.eval()
predictions = []
for batch in test_loader:
x_data, y_data = batch[0], batch[1]
if self.cuda:
x_data = x_data.cuda()
y_data = y_data.cuda()
y_pred = self.net(x_data)
predictions.append(y_pred.detach().cpu().numpy())
predictions = np.concatenate(predictions)
return predictions
:
def predict(self, X, y=None):
predictions = self.predict_proba(X, y)
predictions = predictions.argmax(axis=1)
return predictions
class PytorchModel(sklearn.base.BaseEstimator):
def __init__(self, net_type, net_params, optim_type, optim_params, loss_fn,
input_shape, batch_size=32, accuracy_tol=0.02, tol_epochs=10,
cuda=True):
self.net_type = net_type
self.net_params = net_params
self.optim_type = optim_type
self.optim_params = optim_params
self.loss_fn = loss_fn
self.input_shape = input_shape
self.batch_size = batch_size
self.accuracy_tol = accuracy_tol
self.tol_epochs = tol_epochs
self.cuda = cuda
def fit(self, X, y):
self.net = self.net_type(**self.net_params)
if self.cuda:
self.net = self.net.cuda()
self.optim = self.optim_type(self.net.parameters(), **self.optim_params)
uniq_classes = np.sort(np.unique(y))
self.classes_ = uniq_classes
X = X.reshape(-1, *self.input_shape)
x_tensor = torch.tensor(X.astype(np.float32))
y_tensor = torch.tensor(y.astype(np.long))
train_dataset = TensorDataset(x_tensor, y_tensor)
train_loader = DataLoader(train_dataset, batch_size=self.batch_size,
shuffle=True, drop_last=False)
last_accuracies = []
epoch = 0
keep_training = True
while keep_training:
self.net.train()
train_samples_count = 0
true_train_samples_count = 0
for batch in train_loader:
x_data, y_data = batch[0], batch[1]
if self.cuda:
x_data = x_data.cuda()
y_data = y_data.cuda()
y_pred = self.net(x_data)
loss = self.loss_fn(y_pred, y_data)
self.optim.zero_grad()
loss.backward()
self.optim.step()
y_pred = y_pred.argmax(dim=1, keepdim=False)
true_classified = (y_pred == y_data).sum().item()
true_train_samples_count += true_classified
train_samples_count += len(x_data)
train_accuracy = true_train_samples_count / train_samples_count
last_accuracies.append(train_accuracy)
if len(last_accuracies) > self.tol_epochs:
last_accuracies.pop(0)
if len(last_accuracies) == self.tol_epochs:
accuracy_difference = max(last_accuracies) - min(last_accuracies)
if accuracy_difference <= self.accuracy_tol:
keep_training = False
def predict_proba(self, X, y=None):
X = X.reshape(-1, *self.input_shape)
x_tensor = torch.tensor(X.astype(np.float32))
if y:
y_tensor = torch.tensor(y.astype(np.long))
else:
y_tensor = torch.zeros(len(X), dtype=torch.long)
test_dataset = TensorDataset(x_tensor, y_tensor)
test_loader = DataLoader(test_dataset, batch_size=self.batch_size,
shuffle=False, drop_last=False)
self.net.eval()
predictions = []
for batch in test_loader:
x_data, y_data = batch[0], batch[1]
if self.cuda:
x_data = x_data.cuda()
y_data = y_data.cuda()
y_pred = self.net(x_data)
predictions.append(y_pred.detach().cpu().numpy())
predictions = np.concatenate(predictions)
return predictions
def predict(self, X, y=None):
predictions = self.predict_proba(X, y)
predictions = predictions.argmax(axis=1)
return predictions
, :
base_model = PytorchModel(net_type=SimpleCNN, net_params=dict(), optim_type=Adam,
optim_params={"lr": 1e-3}, loss_fn=nn.CrossEntropyLoss(),
input_shape=(1, 8, 8), batch_size=32, accuracy_tol=0.02,
tol_epochs=10, cuda=True)
base_model.fit(x_train_scaled, y_train)
preds = base_model.predict(x_test_scaled)
true_classified = (preds == y_test).sum()
test_accuracy = true_classified / len(y_test)
print(f"Test accuracy: {test_accuracy}")
>>> Test accuracy: 0.7361111111111112
.
meta_classifier = BaggingClassifier(base_estimator=base_model, n_estimators=10)
meta_classifier.fit(x_train_scaled.reshape(-1, 64), y_train)
>>> BaggingClassifier(
base_estimator=PytorchModel(accuracy_tol=0.02, batch_size=32,
cuda=True, input_shape=(1, 8, 8),
loss_fn=CrossEntropyLoss(),
net_params={},
net_type=<class '__main__.SimpleCNN'>,
optim_params={'lr': 0.001},
optim_type=<class 'torch.optim.adam.Adam'>,
tol_epochs=10),
bootstrap=True, bootstrap_features=False, max_features=1.0,
max_samples=1.0, n_estimators=10, n_jobs=None,
oob_score=False, random_state=None, verbose=0,
warm_start=False)
score
accuracy :
print(meta_classifier.score(x_test_scaled.reshape(-1, 64), y_test))
>>> 0.95
, . . . , , ( "" - ).
?
-, . , Loss' - .
-, Boosting. Bagging' , , . ( ) . sklearn, Loss - .
, . , "" . , .
, . — - , , , , , .
. , Data science' . Computer vision, . ( — !) — FARADAY Lab. — , .
c: