The classification of documents or text is one of the most important tasks in natural language processing (NLP).
It has many uses, such as categorizing news, filtering spam, searching for inappropriate comments, etc.
Large companies have no problem collecting large data sets, so learning a text classification model from scratch is a feasible task.
However, for most real-world tasks, large data sets are rare, and you have to be smart to build your model.
In this article I will talk about practical approaches to text transformations that will make it possible to classify documents, even if the data set is small.
Introduction to Document Classification
The process of document classification begins with the cleaning and preparation of the body.
Then this body is encoded with any type of text representation, after which you can start modeling.
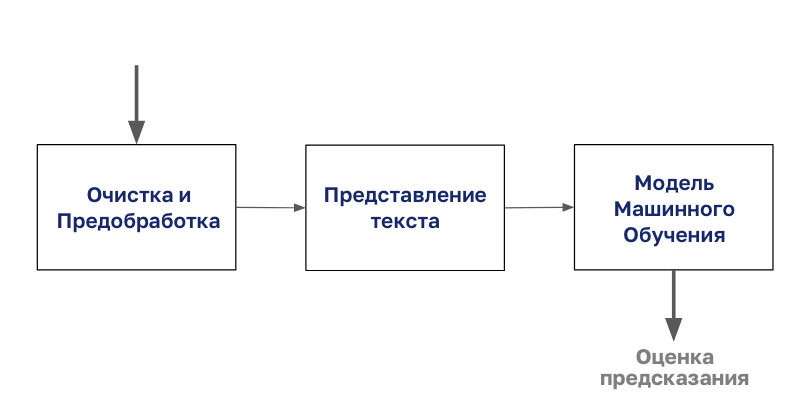
In this article, we will focus on the “Presenting Text” step from this diagram.
Test dataset for classification
We will use the data from the competition. True or not? NLP with Kaggle disaster tweets.
The challenge is to predict which tweets were about real disasters and which were not.
If you want to repeat the article step by step, do not forget to install the libraries used in it.
:
import pandas as pd
tweet= pd.read_csv('../input/nlp-getting-started/train.csv')
test=pd.read_csv('../input/nlp-getting-started/test.csv')
tweet.head(3)

, , , .
.
print('There are {} rows and {} columns in train'.format(tweet.shape[0],tweet.shape[1]))
print('There are {} rows and {} columns in test'.format(test.shape[0],test.shape[1]))
8000 .
, 280 .
, NLP, .
, , , .
, :
- — .
- - — «a» «the».
- — («studies», «studing» → «study»).
def preprocess_news(df):
'''Function to preprocess and create corpus'''
new_corpus=[]
lem=WordNetLemmatizer()
for text in df["question_text"]:
words=[w for w in word_tokenize(text) if (w not in stop)]
words=[lem.lemmatize(w) for w in words]
new_corpus.append(words)
return new_corpus
corpus=preprocess_news(df)
, , .
, .
.
CountVectorizer
CountVectorizer — .
, , .
:
text = ["She sells seashells in the seashore"]
vectorizer = CountVectorizer()
vectorizer.fit(text)
print(vectorizer.vocabulary_)
vector = vectorizer.transform(text)
print(vector.shape)
print(type(vector))
print(vector.toarray())

, CountVectorizer Numpy, .
, , .
vector=vectorizer.transform(["I sell seashells in the seashore"])
vector.toarray()

, :
, — «sells» «she».
CountVectorizer, .
vec=CountVectorizer(max_df=10,max_features=10000)
vec.fit(df.question_text.values)
vector=vec.transform(df.question_text.values)
, CountVectorizer , :
- max_features — n , .
- min_df — , .
- max_df — , .
( ).
TfidfVectorizer
Countvectorizer , , "the" ( ) .
— TfidfVectorizer.
— Term frequency-inverse document frequency ( — ).
:
from sklearn.feature_extraction.text import TfidfVectorizer
text = ["She sells seashells by the seashore","The sea.","The seashore"]
vectorizer = TfidfVectorizer()
vectorizer.fit(text)
print(vectorizer.vocabulary_)
print(vectorizer.idf_)
vector = vectorizer.transform([text[0]])
print(vector.shape)
print(vector.toarray())
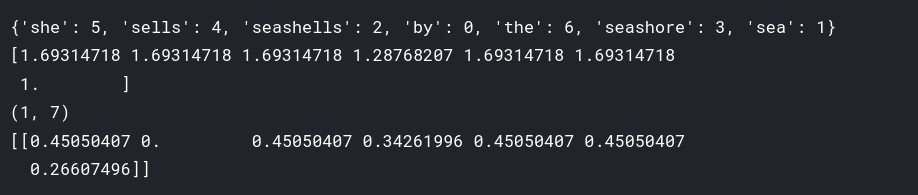
6 , «the», 4 .
0 1, - .
Word2vec
, .
(embeddings) .
n- .
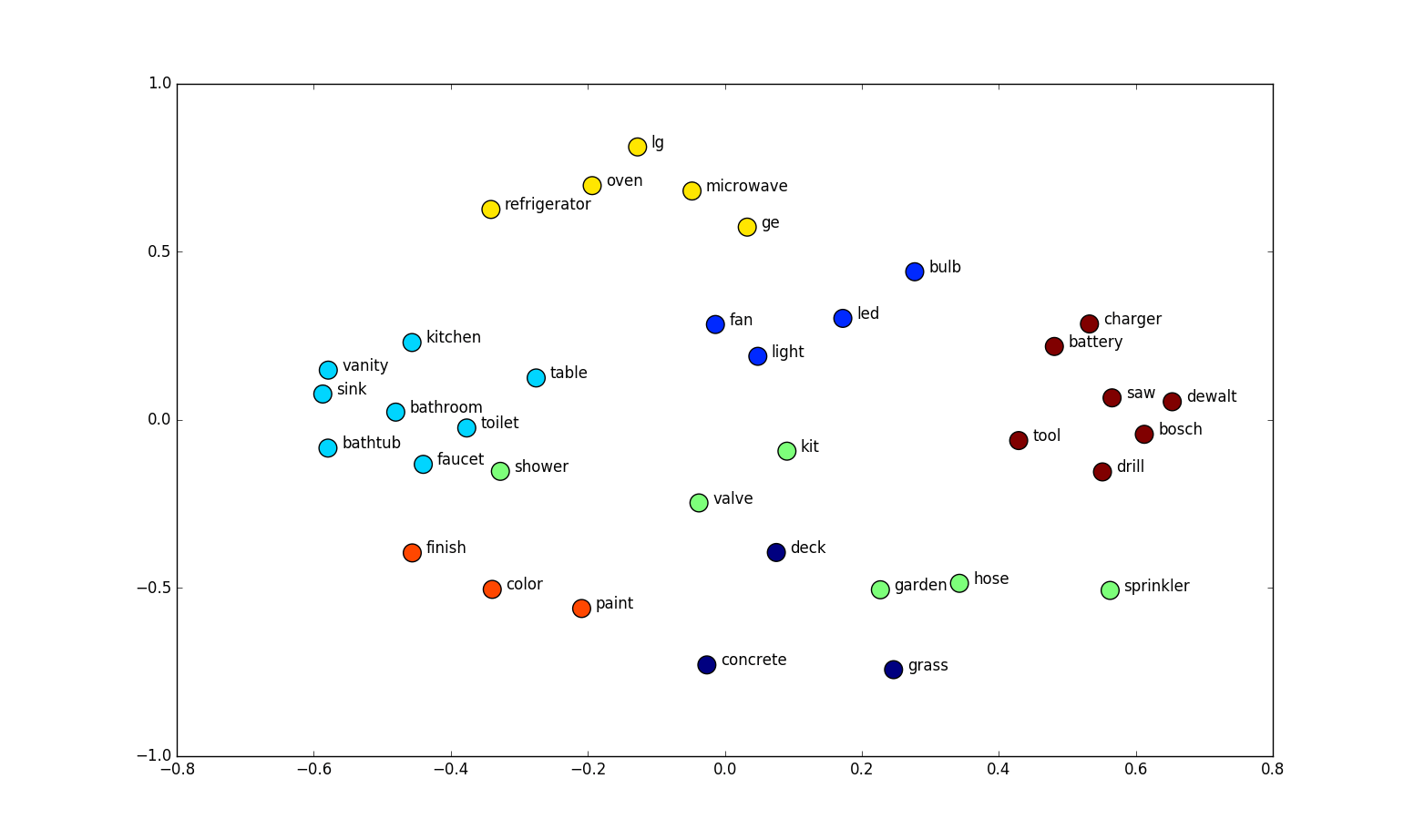
Word2Vec Google .
, .
, , .
«The cat sat on the mat».
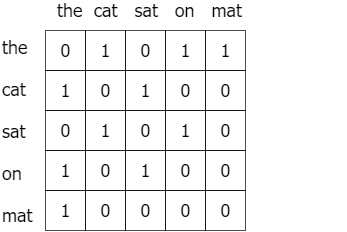
Word2vec :
, , , . word2vec python:
import gensim
from gensim.models import Word2Vec
model = gensim.models.Word2Vec(corpus,
min_count = 1, size = 100, window = 5)
, word2vec.
:
- size — .
- min_count — .
- window — , . .
.
.
.
, gensim.
from gensim.models.KeyedVectors import load_word2vec_format
def load_word2vec():
word2vecDict = load_word2vec_format(
'../input/word2vec-google/GoogleNews-vectors-negative300.bin',
binary=True, unicode_errors='ignore')
embeddings_index = dict()
for word in word2vecDict.wv.vocab:
embeddings_index[word] = word2vecDict.word_vec(word)
return embeddings_index
:
w2v_model=load_word2vec()
w2v_model['London'].shape

, 300- .
( — , . , , . . )
, .
FastText
Genism — FastText.
Facebook .
Continuous Bag of Words Skip-Gram.
FastText , n-.
, , «orange».
«ora», «ran», «ang», «nge» ( ).
( ) «orange» n-.
, n- .
, «stupedofantabulouslyfantastic», , , , genism , .
FastText , , .
«fantastic» «fantabulous».
, .
.
n- .
.
from gensim.models import FastText
def load_fasttext():
print('loading word embeddings...')
embeddings_index = {}
f = open('../input/fasttext/wiki.simple.vec',encoding='utf-8')
for line in tqdm(f):
values = line.strip().rsplit(' ')
word = values[0]
coefs = np.asarray(values[1:], dtype='float32')
embeddings_index[word] = coefs
f.close()
print('found %s word vectors' % len(embeddings_index))
return embeddings_index
embeddings_index=load_fastext()
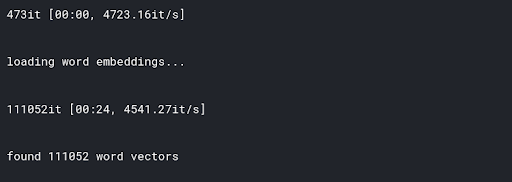
:
embeddings_index['london'].shape

GloVe
GloVe (global vectors for word representation) « ».
, .
, .
word2vec, .
.
.
.
.
, .
n- .
:

.
.
:
def load_glove():
embedding_dict = {}
path = '../input/glove-global-vectors-for-word-representation/glove.6B.100d.txt'
with open(path, 'r') as f:
for line in f:
values = line.split()
word = values[0]
vectors = np.asarray(values[1:], 'float32')
embedding_dict[word] = vectors
f.close()
return embedding_dict
embeddings_index = load_glove()
, , GloVe.
- .
embeddings_index['london'].shape
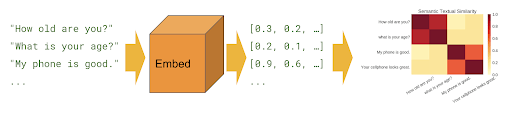
.
.
.
, .
:
, .
.
.
Tensorflow.
module_url = "../input/universalsentenceencoderlarge4"
embed = hub.load(module_url)
.
sentence_list=df.question_text.values.tolist()
sentence_emb=embed(sentence_list)['outputs'].numpy()
.
Elmo, BERT
, .
.
«stick», «», «» , , .
NLP BERT , . .
, Keras .
, .
, Keras Tokenizer pad_sequences.
MAX_LEN=50
tokenizer_obj=Tokenizer()
tokenizer_obj.fit_on_texts(corpus)
sequences=tokenizer_obj.texts_to_sequences(corpus)
tweet_pad=pad_sequences(sequences,
maxlen=MAX_LEN,
truncating='post',
padding='post')
.
word_index=tokenizer_obj.word_index
print('Number of unique words:',len(word_index))
, .
.
def prepare_matrix(embedding_dict, emb_size=300):
num_words = len(word_index)
embedding_matrix = np.zeros((num_words, emb_size))
for word, i in tqdm(word_index.items()):
if i > num_words:
continue
emb_vec = embedding_dict.get(word)
if emb_vec is not None:
embedding_matrix[i] = emb_vec
return embedding_matrix
.
trainable=False, .
def new_model(embedding_matrix):
inp = Input(shape=(MAX_LEN,))
x = Embedding(num_words, embedding_matrix.shape[1], weights=[embedding_matrix],
trainable=False)(inp)
x = Bidirectional(
LSTM(60, return_sequences=True, name='lstm_layer',
dropout=0.1, recurrent_dropout=0.1))(x)
x = GlobalAveragePool1D()(x)
x = Dense(1, activation="sigmoid")(x)
model = Model(inputs=inp, outputs=x)
model.compile(loss='binary_crossentropy',
optimizer='adam',
metrics=['accuracy'])
return model
, , word2vec:
embeddings_index=load_word2vec()
embedding_matrix=prepare_matrix(embeddings_index)
model=new_model(embedding_matrix)
history=model.fit(X_train,y_train,
batch_size=8,
epochs=5,
validation_data=(X_test,y_test),
verbose=2)
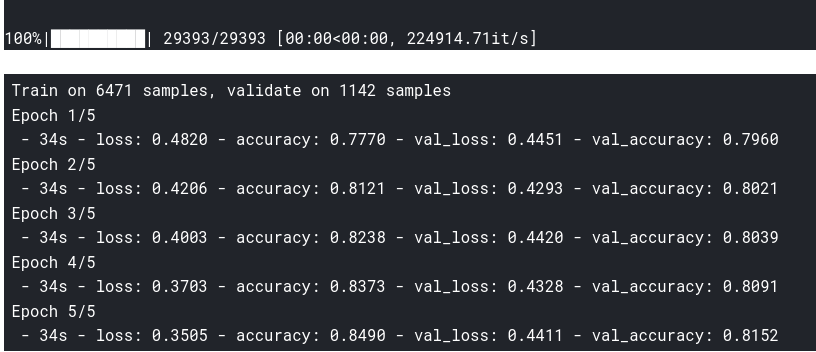
, .
?
Neptune .
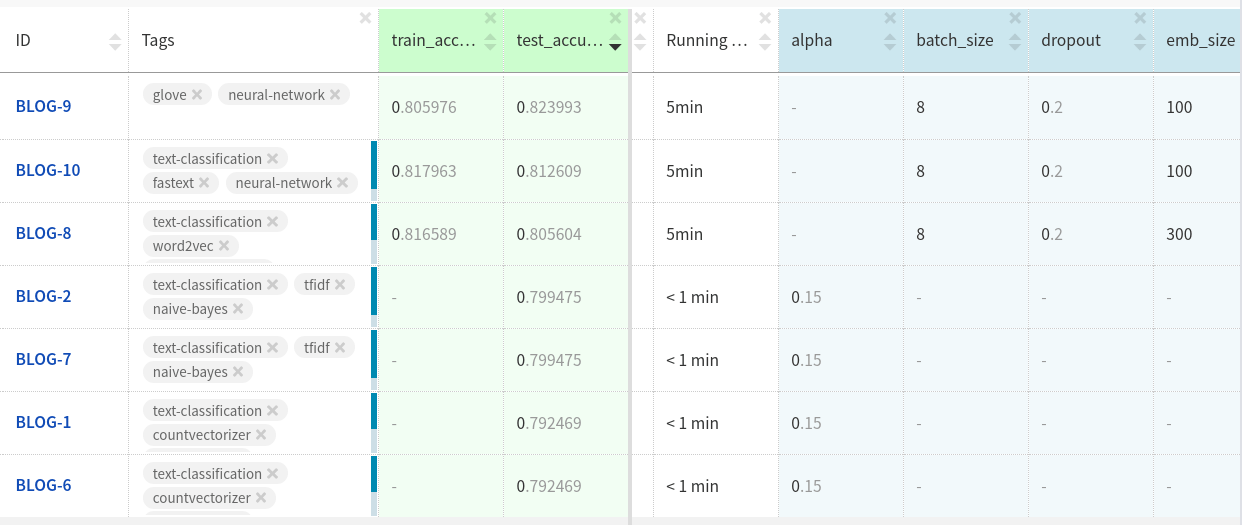
GloVe attachments in test datasets performed slightly better than the other two attachments.
Perhaps you will achieve more if you further customize the model and clean the data.
You can look at the experiments here .
Conclusion
In this article, we examined and implemented various methods for presenting attributes for text classification used when working with small data sets.
I hope they come in handy in your projects.