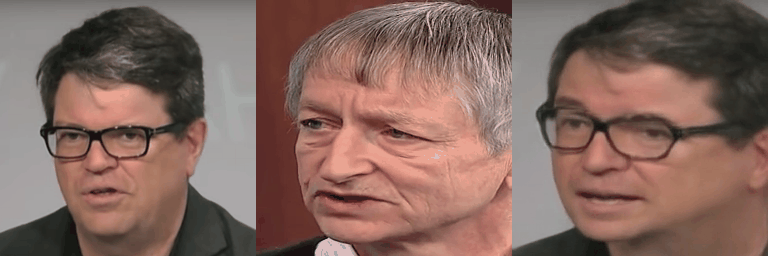
First Order Motion Model working example
Is it possible to make an entire movie from one photograph? And having recorded the movements of one person, replace him with another in the video? Of course, the answer to these questions is extremely important for areas such as cinema, photography, and the development of computer games. The solution could be digital photo processing using specialized software. The problem in question among specialists in this field is called the task of automatic synthesis of video or image animation.
To obtain the expected result, existing approaches combine objects extracted from the original image and movements that can be delivered as a separate video - βdonorβ.
Now, in most areas, image animation is done using computer graphics tools. This approach requires additional knowledge about the object that we want to animate - its 3D model is usually necessary (how it works now in the film industry can be found here ). Most of the latest solutions to this problem are based on in-depth training of models, which are based on generative-competitive neural networks (GAN) and variational autoencoders (VAE). These models usually use pre-trained modules to search for key points of objects in the image. The main problem with this approach is that these modules can only recognize the objects on which they were trained.
, ? Β«First Order Motion Model for Image AnimationΒ». β First Order Motion Model, . , (, , ), , .
β¦
, .
, , (occlusion map). . , , .
: .
. .
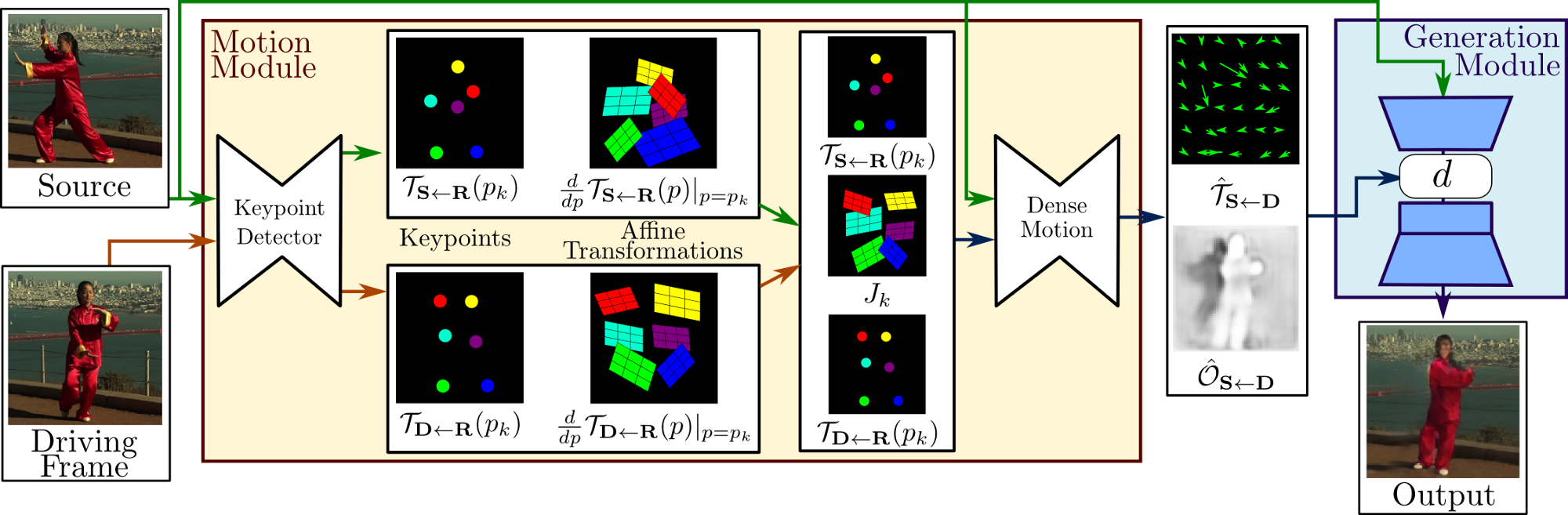
. , ( ) . . .
.
. . , ( ), . , , . , .
:

, , .
:
. U-Net, , .
softmax , .
( ), . , , , , . , (), . U-Net.
:

β ( β ) :

, . , . down-sampling . c . , . β , , , . :
, β ( ).
, . , .
, . reconstruction loss, . - VGG-19. reconstruction loss :
β , β , β i- , VGG-19, β .
- . . , . , . , , , .
, , , thin plane spline. . ,
. C :
( β ):

. , reconstruction loss 2 .
, . . , :

, β .
!
4 :
- VoxCeleb β 22496 , YouTube;
- UvA-Nemo β , 1240 ;
- BAIR robot pushing β , , , . 42880 128 .
- 280 TaiChi YouTube.
X2Face Monkey-Net, .

As can be seen from the table, the First Order Motion model is superior to other approaches in all respects.
The long-awaited examples

Mgif
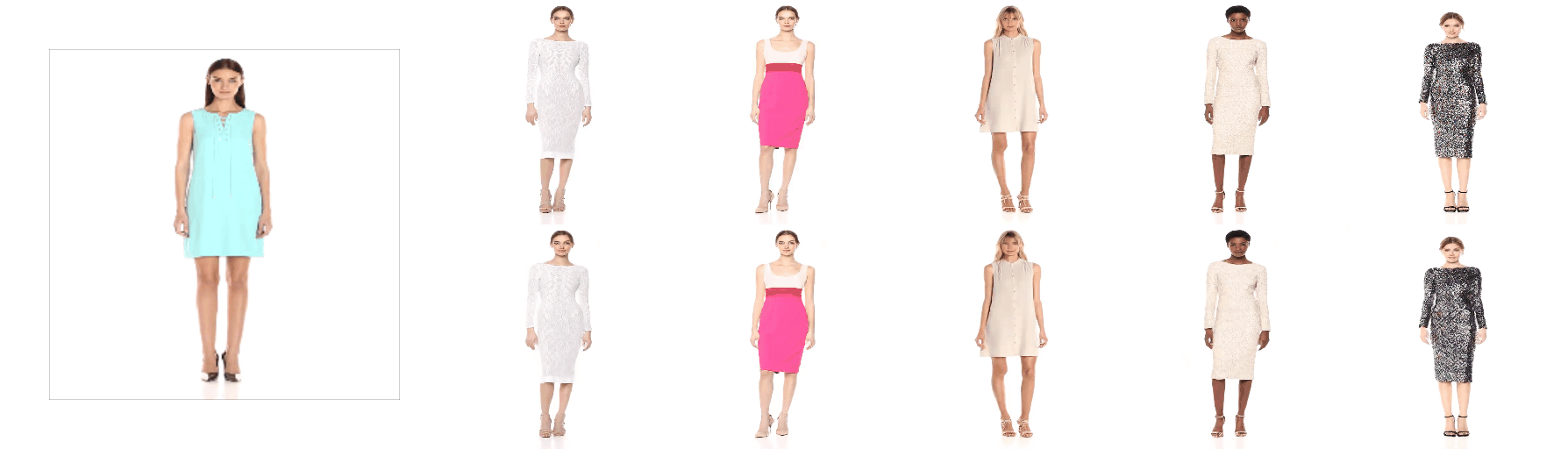
Fashion
Now try it yourself! It is very simple, everything is prepared here .