In a previous article, we looked at some simple ways to speed up Pandas through jit compilation and using multiple cores using tools like Numba and Pandarallel. This time we will talk about more powerful tools with which you can not only speed up pandas, but also cluster it, thus allowing you to process big data.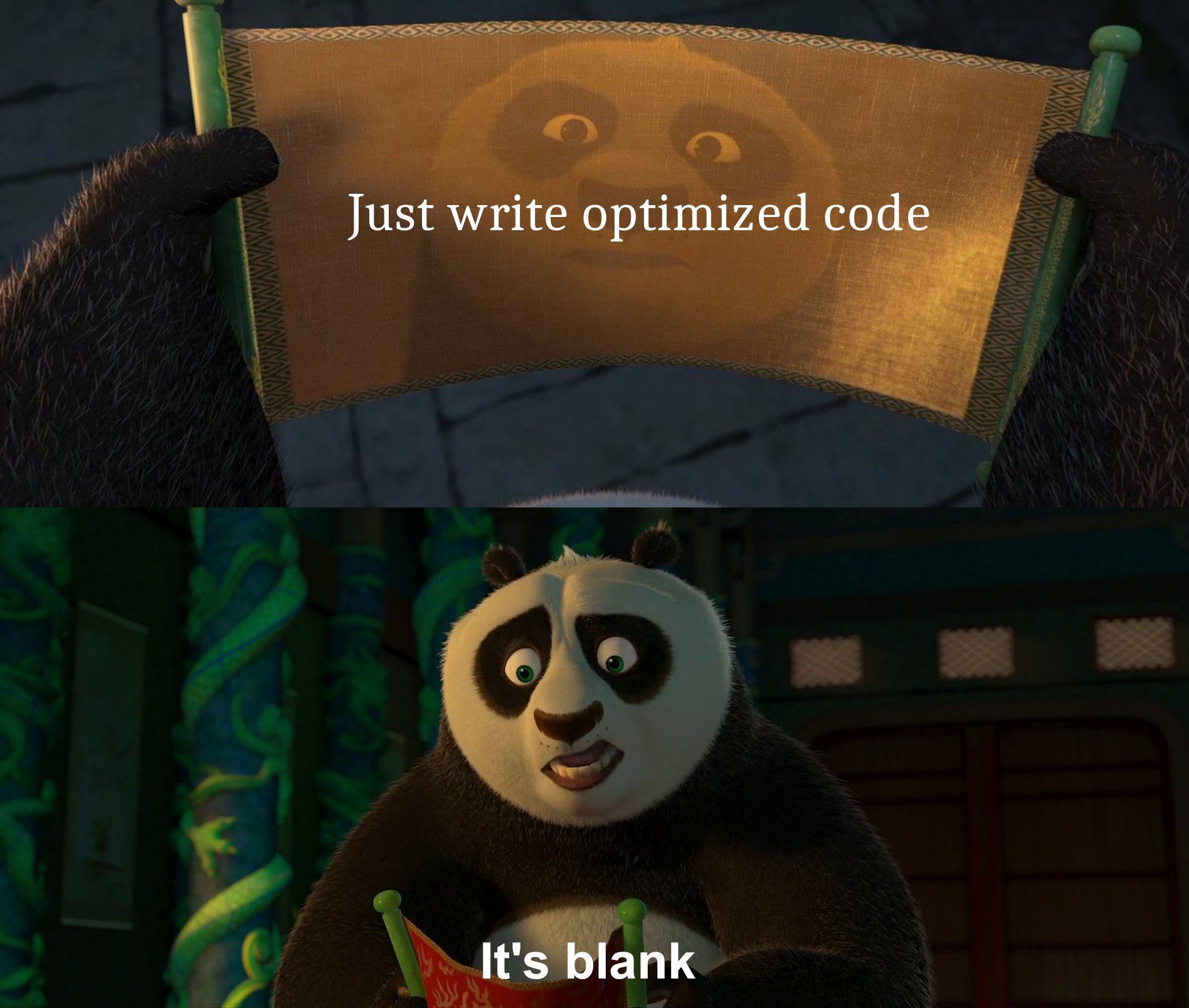
- Numba
- Multiprocessing
- Pandarallel
Part 2
Swifter
Swifter — , pandas. — , pandas. pandarallel , Dask, .
:
- ( )
- , - , , swifter .
:
def multiply(x):
return x * 5
, swifter, pandas, , pandarallel:

, , swifter , , . , .
, . , swifter:
def mean_word_len(line):
for i in range(6):
words = [len(i) for i in line.split()]
res = sum(words) / len(words)
return res
df['headline_text'].swifter.allow_dask_on_strings().apply(mean_word_len)
:

. ( 100 000 ), swifter pandas, . , pandas , , swifter , pandarallel.
Modin
Modin , Dask Ray, . , , . modin dataframe
( pandas), ~80% , 20% pandas, API.
, , env
:
%env MODIN_ENGINE=ray
import modin.pandas as mpd
modin . csv 1.2 GB:
df = mpd.read_csv('abcnews-date-text.csv', header=0)
df = mpd.concat([df] * 15)
df.to_csv('big_csv.csv')
modin pandas:
In [1]: %timeit mpd.read_csv('big_csv.csv', header=0)
8.61 s ± 176 ms per loop (mean ± std. dev. of 5 runs, 1 loop each)
In [2]: %timeit pd.read_csv('big_csv.csv', header=0)
22.9 s ± 1.95 s per loop (mean ± std. dev. of 5 runs, 1 loop each)
3 . , , - . modin :

, apply
— modin, , RAM . , , :
df = pd.DataFrame(np.random.randint(0, 100, size=(10**7, 6)), columns=list('abcdef'))

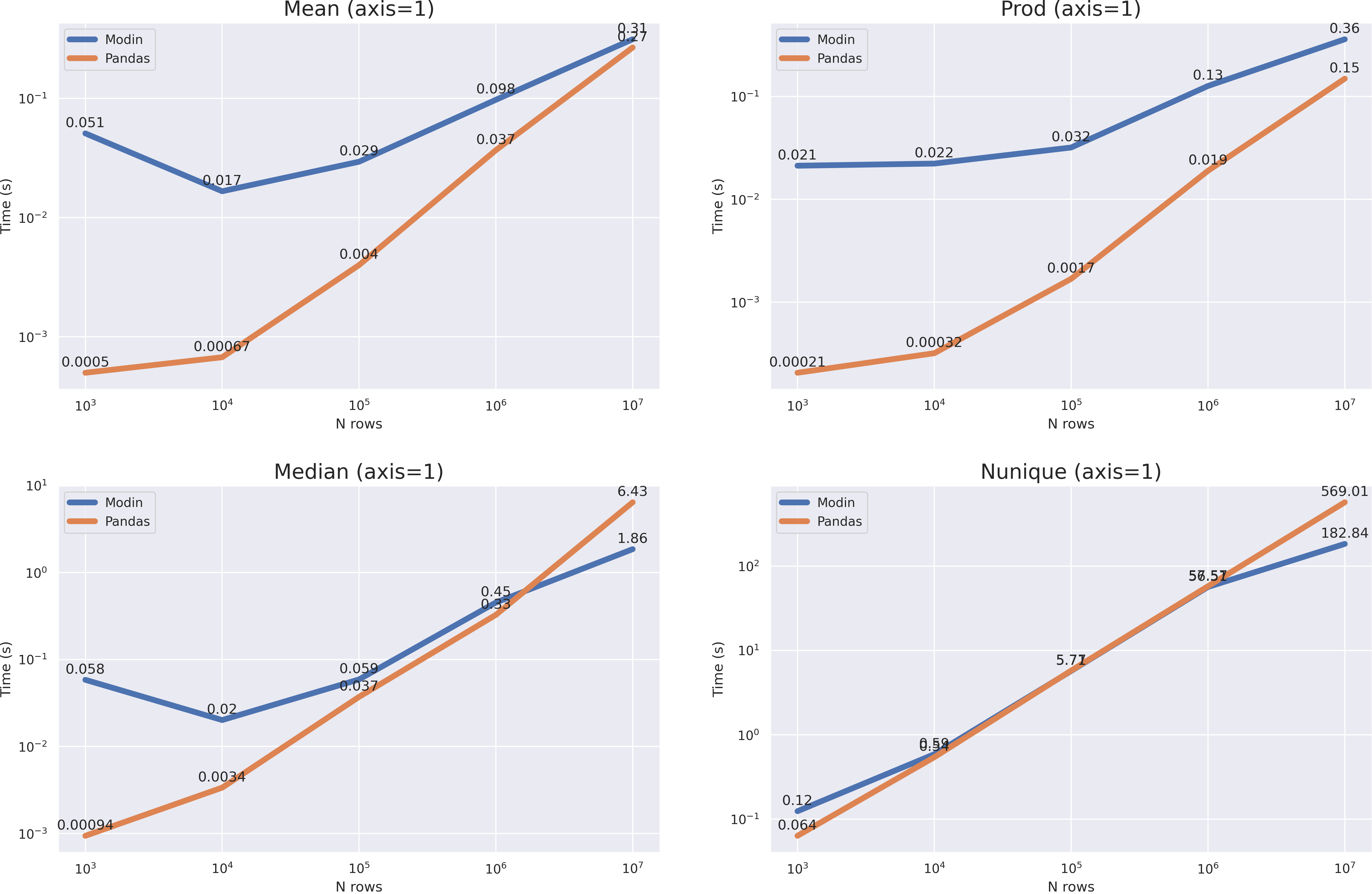
? . median
nunique
10**7
, mean
prod(axis=1)
, , pandas 10**8
modin .
- API modin pandas, ,
- , . , 1GB
- — 80%
- , — Ray/Dask modin
- , ,
- Ray Dask . Ray:

Dask
Dask — . , . numpy pandas, — dask sklearn xgboost, . , . pandas.
dask — .
from distributed import Client
client = Client(n_workers=8)
Dask, modin, dataframe
, :
import dask.dataframe as dd
. :
In [1]: %timeit dd.read_csv('big_csv.csv', header=0)
6.79 s ± 798 ms per loop (mean ± std. dev. of 7 runs, 1 loop each)
In [2]: %timeit pd.read_csv('big_csv.csv', header=0)
19.8 s ± 2.75 s per loop (mean ± std. dev. of 7 runs, 1 loop each)
Dask - 3 . — apply
. pandarallel swifter, :
dd.from_pandas(df, npartitions=8).apply(mean_word_len, meta=(float)).compute(),

, dask , 10**4
. :
df = pd.DataFrame(np.random.randint(0, 100, size=(10**7, 6)), columns=list('abcdef'))

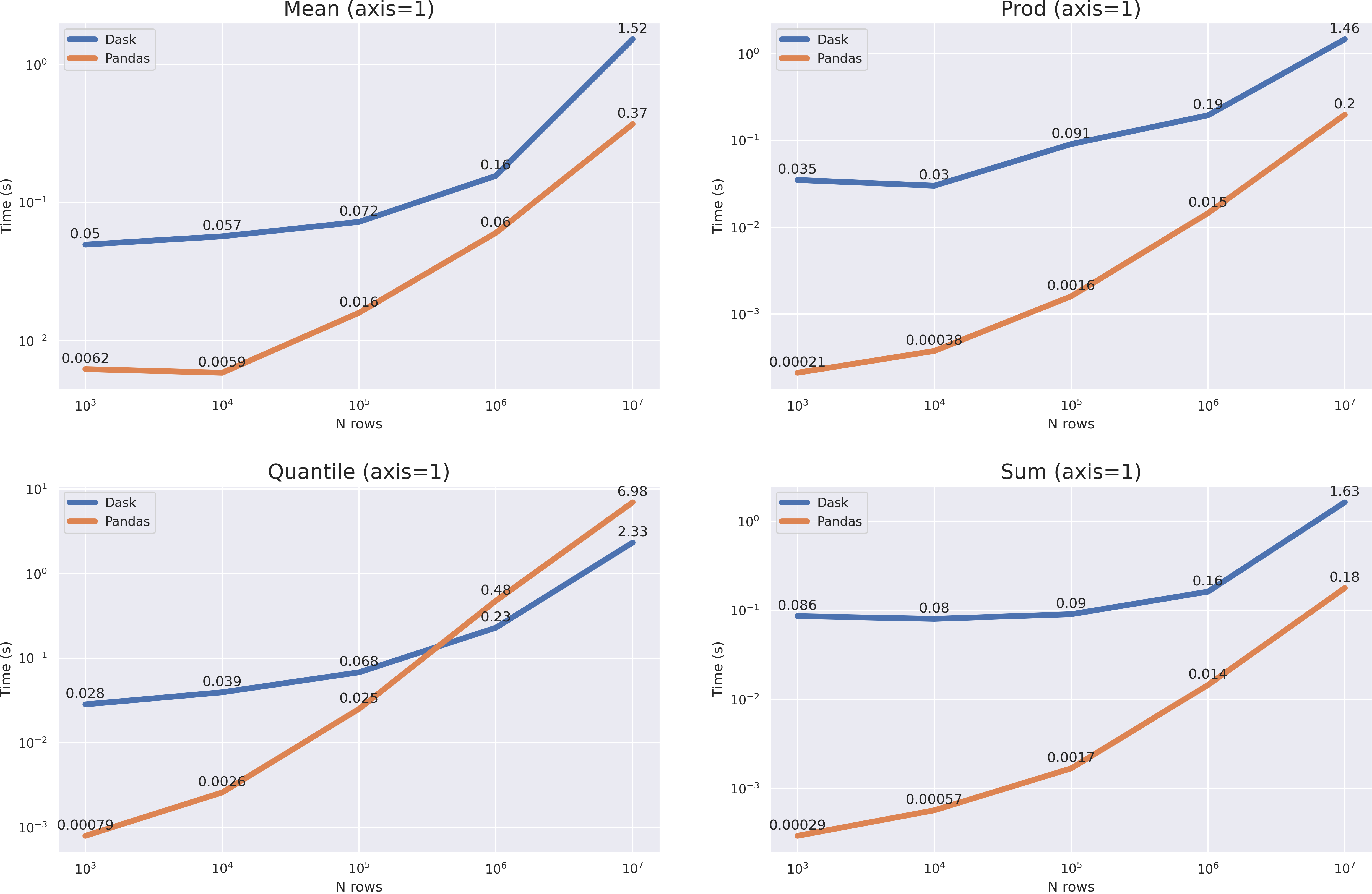
modin, , . axis=0
, , >10**8
dask . axis=1
pandas ( quantile(axis=1)
).
, pandas , , dask — , , pandas ( , RAM).
apply
- . , .
- ,
- API dask pandas, , Dask
- :

Conclusion
, , . , Dask, : ? ? ? , , .
! , !
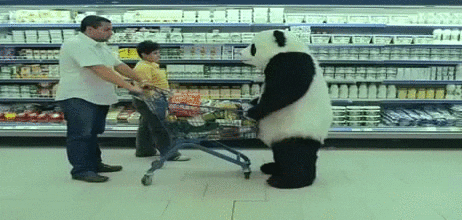
P.s Trust, but verify — , ( ), github