In this article I will talk about six tools that can significantly speed up your pandas code. I assembled the tools according to one principle - ease of integration into the existing code base. For most tools, you just need to install the module and add a couple lines of code.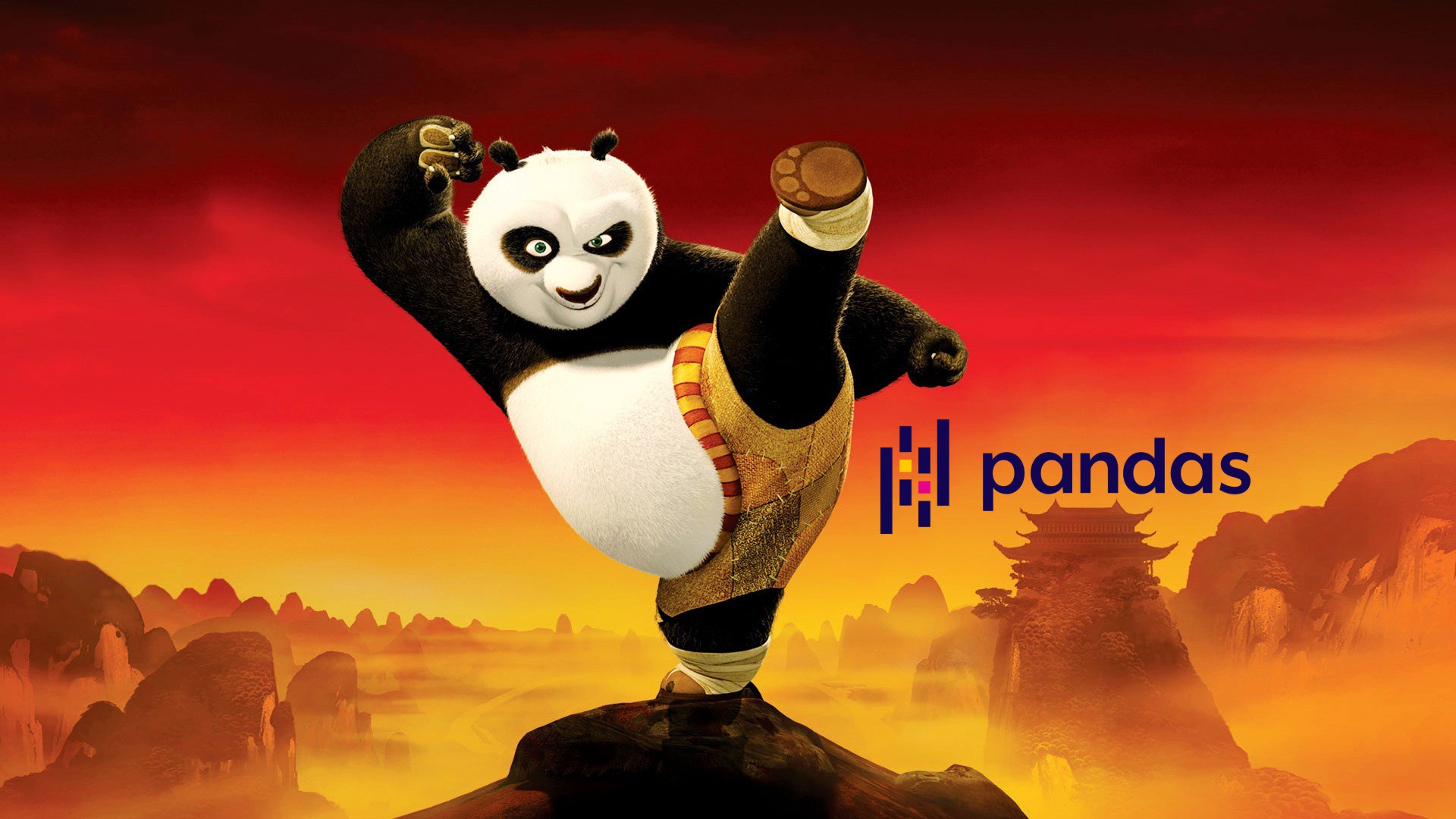
Pandas API, , . , , , .
, Spark DataFlow. :
1:
- Numba
- Multiprocessing
- Pandarallel
Numba
Python. Numba — JIT , , Numpy, Pandas. , .
— , - apply
.
import numpy as np
import numba
df = pd.DataFrame(np.random.randint(0,100,size=(100000, 4)),columns=['a', 'b', 'c', 'd'])
def multiply(x):
return x * 5
@numba.vectorize
def multiply_numba(x):
return x * 5
, . . .
In [1]: %timeit df['new_col'] = df['a'].apply(multiply)
23.9 ms ± 1.93 ms per loop (mean ± std. dev. of 7 runs, 10 loops each)
In [2]: %timeit df['new_col'] = df['a'] * 5
545 µs ± 21.4 µs per loop (mean ± std. dev. of 7 runs, 1000 loops each)
In [3]: %timeit df['new_col'] = multiply_numba(df['a'].to_numpy())
329 µs ± 2.37 µs per loop (mean ± std. dev. of 7 runs, 1000 loops each)
~70 ! , , Pandas , . :
def square_mean(row):
row = np.power(row, 2)
return np.mean(row)
@numba.njit
def square_mean_numba(arr):
res = np.empty(arr.shape[0])
arr = np.power(arr, 2)
for i in range(arr.shape[0]):
res[i] = np.mean(arr[i])
return res
:
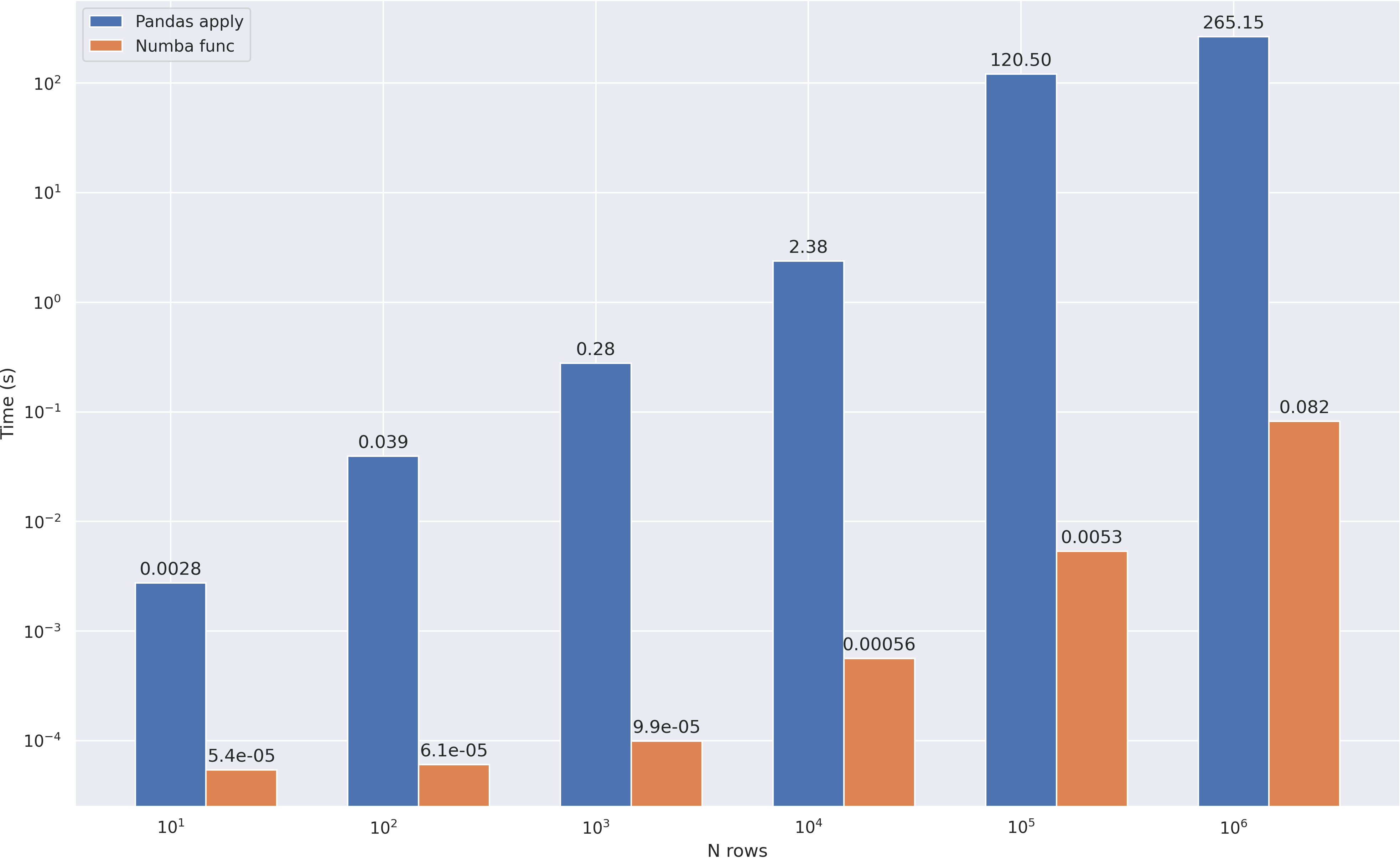
Multiprocessing
, , , . - , python.
. . , apply
:
df = pd.read_csv('abcnews-date-text.csv', header=0)
df = pd.concat([df] * 10)
df.head()
def mean_word_len(line):
for i in range(6):
words = [len(i) for i in line.split()]
res = sum(words) / len(words)
return res
def compute_avg_word(df):
return df['headline_text'].apply(mean_word_len)
:
from multiprocessing import Pool
n_cores = 4
pool = Pool(n_cores)
def apply_parallel(df, func):
df_split = np.array_split(df, n_cores)
df = pd.concat(pool.map(func, df_split))
return df
:

Pandarallel
Pandarallel — pandas, . , , + progress bar ;) , pandarallel.

. , . pandarallel — :
from pandarallel import pandarallel
pandarallel.initialize()
, — apply
parallel_aply
:
df['headline_text'].parallel_apply(mean_word_len)
:

- overhead 0.5 .
parallel_apply
, . 1 , , . - , , , 2-3 .
- Pandarallel
parallel_apply
(groupby
), .
, , . / , API progress bar.
To be continued
In this part, we looked at 2 fairly simple approaches to pandas optimization - using jit compilation and task parallelization. In the next part I will talk about more interesting and complex tools, but for now I suggest you test the tools yourself to make sure they are effective.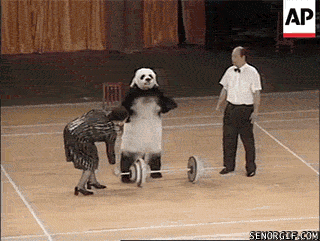
PS: Trust, but verify - all the code used in the article (benchmarks and graphing), I posted on github