How much longer is it necessary to extend self-isolation in the country and regions so that every first one does not get sick, do they apply strict sanctions to violators of self-isolation, call for stronger precautions or leave the majority of the population with sincere faith in the usefulness of medical masks?
To get answers to at least some of the questions, this article will analyze the SIR model from the sensational YouTube video and simulations of various epidemiological situations based on it, as well as a qualitative comparison of the influence of various factors on the speed and scale of the spread of infection.
In addition, further posts will explore more complex models describing the dynamics of the spread of the COVID-19 virus.
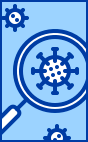
UFO Care Minute
The pandemic COVID-19, a potentially severe acute respiratory infection caused by the SARS-CoV-2 coronavirus (2019-nCoV), has officially been announced in the world. There is a lot of information on HabrΓ© on this topic - always remember that it can be both reliable / useful, and vice versa.
We urge you to be critical of any published information.
Wash your hands, take care of your loved ones, stay at home whenever possible and work remotely.
Read publications about: coronavirus | remote work
Brief description of the model
So, let's imagine that we are observing some closed city, where N residents live on a permanent basis, who do not go anywhere and do not host nonresident guests, but are quite socially active inside their city.
That is, we observe a connected closed community (or system). At some point, a person infected with COVID-19 appears in this system, and now, we already divide all the residents of our community into three potential groups:
- S (Susceptible) - vulnerable (can become infected),
- I (Infected) - infected (those who are unlucky),
- R (Removed) β , :
- Recovered β (, , )
- Dead β (, β¦ ).

β
, , , , , .
β , . , . , . , SIR, .
, , . , .
:
- (Dead/Removed) β 12% ( ). .
- 50 ( ).
- 50 .
- ( , ).
:
- CP (contagion probability) β : 30% β , ( ), 20% 10%.
- SD (social distance) β . , . SD β . , 100-SD.
SD , . , . : 10%, 40%*, 75%*, 90%. - IP (isolation period) β . : 30 ( ), 45 ( ), 70 ( ), .
- AC (asymptomatic cases) β ** β 5% ( ), 40% ( ), 70% ( ).
- DT (disease time) β 14 ( ), 21 ( ), 38 ( ).
*
** ,
: CP=30% ( ), SD=75% ( ), IP=unlimited ( ), AC=40% ( ), DT=21 ( ). β .
CP

: 10%, 20%, 30%
β , ( !). ( , , ) .
, , 30% .
, SD

: 10%, 40%, 75%, 90%
. , . 10%, , 90%. , , .
IP
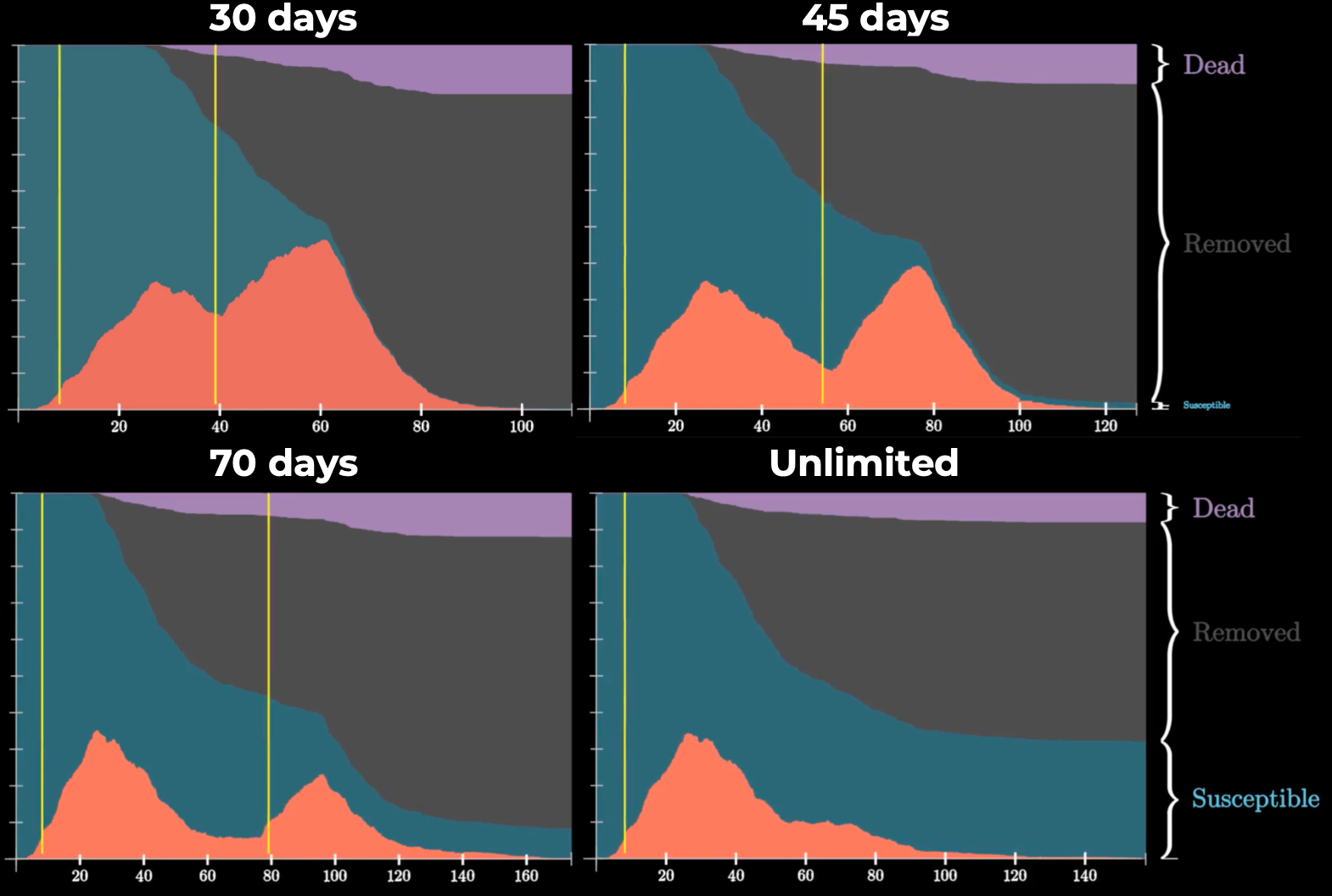
: 30 , 45 , 70 ,
"" . . , .
AC
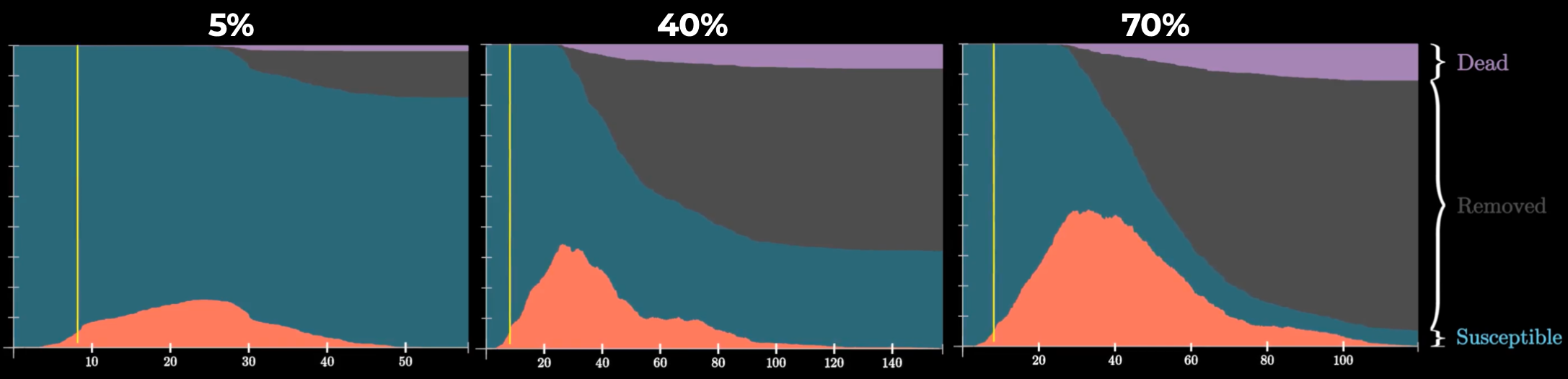
: 5%, 40%, 70%
, 5%, , . 70%, , β .
, COVID-19 .
, : , , .
DT
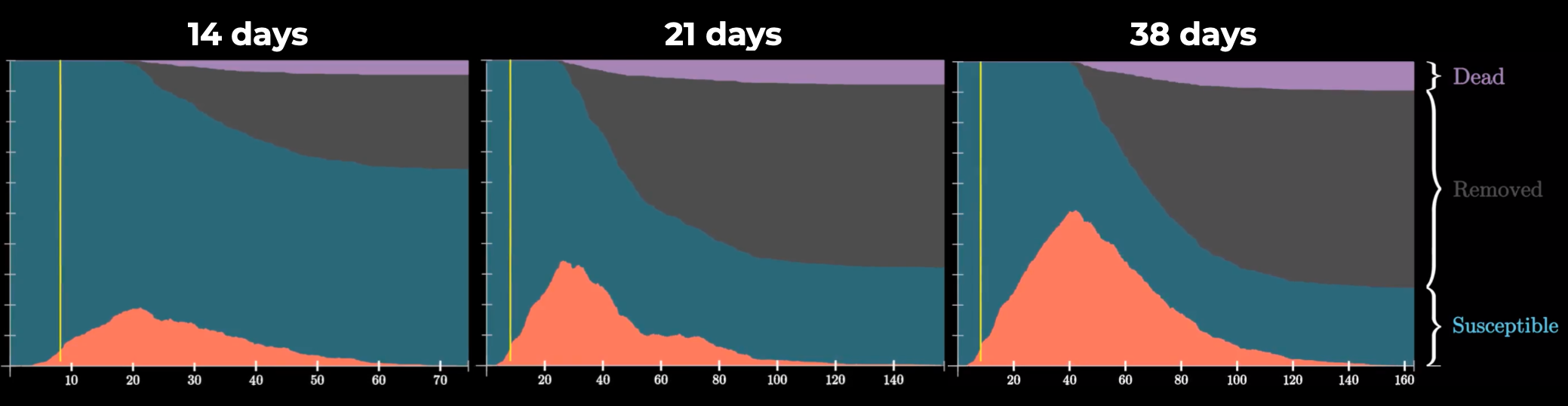
: 14 , 21 , 38
, . , , , .
, , .
" " (, , , ), .

, . 90 .
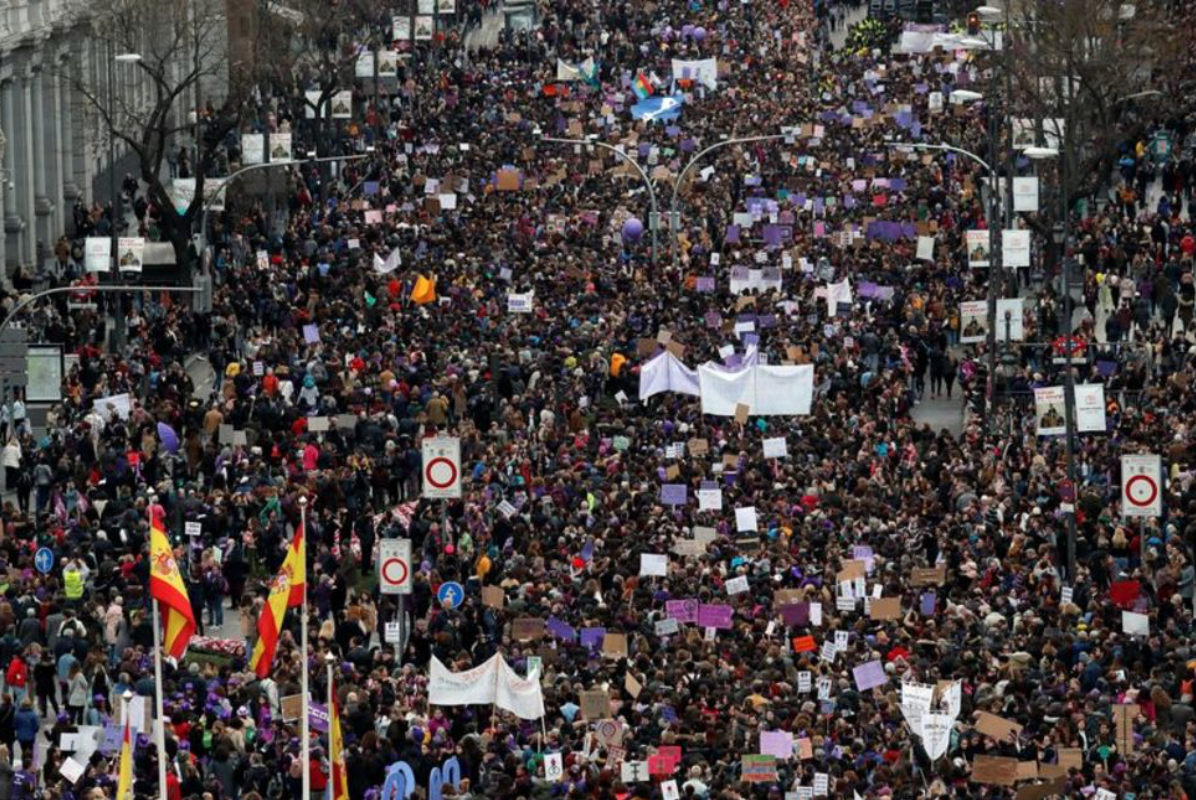
8 , , , .
, , , .
, ,, . 100 86 , . , , 31 2 -, 61- , .
, , , , .
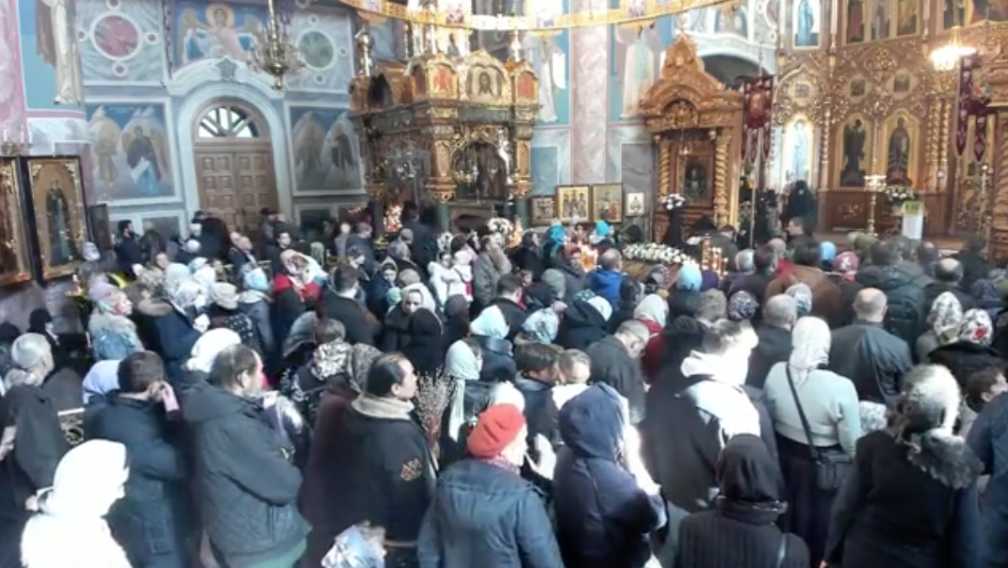
, 12 , - - . . .
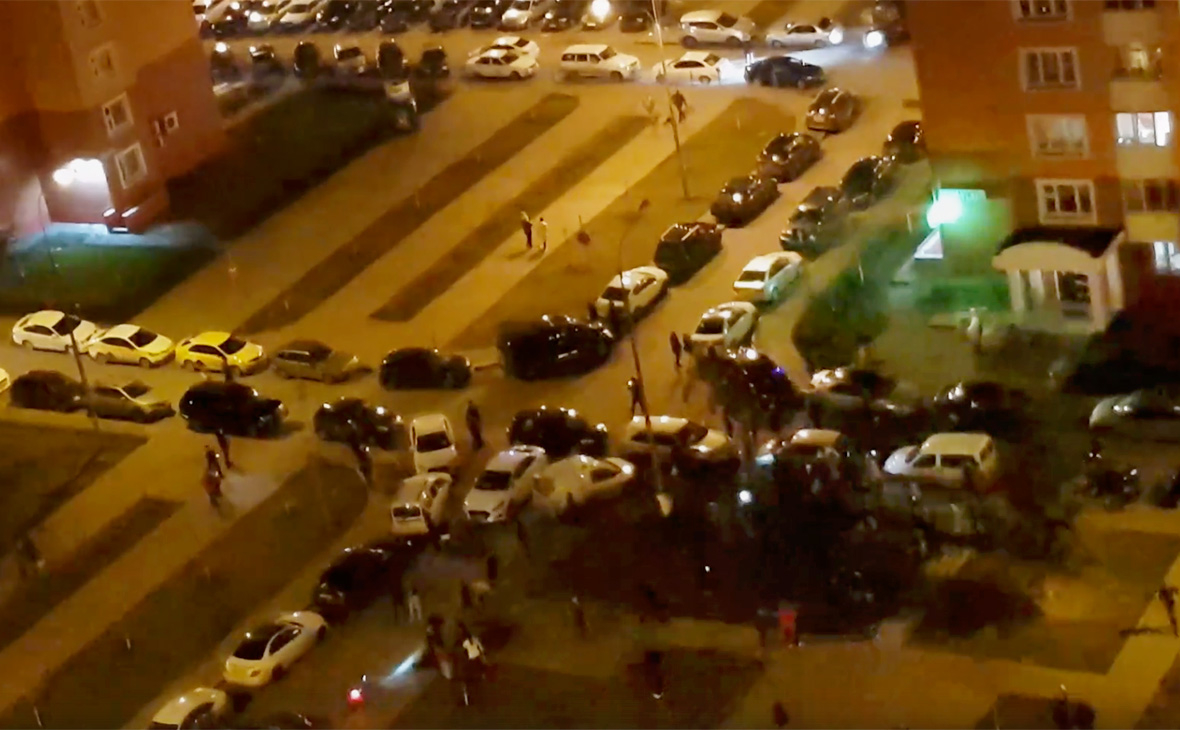
Β« 2Β» Β« Β», ,
Of course, this simple model does not take into account a number of factors influencing the epidemic, both controlled by people and depending only on the nature of the virus itself, and is not always accurately transferred to reality. However, it fulfills its function - it provides a qualitative assessment of the main mechanisms affecting the dynamics of the epidemic, and clearly demonstrates this effect.
Useful materials
Special thanks pixml and keysloss for help in writing an article and preparing simulations.