Hello everyone! My name is Sasha, I am CTO & Co-Founder at LoyaltyLab. Two years ago, I went with friends, like all poor students, in the evening for a beer to the nearest convenience store. We were very upset that the retailer, knowing that we would come for a beer, did not offer a discount on chips or crackers, although this is so logical! We did not understand why this situation was happening and decided to make our company. Well, as a bonus, write yourself discounts every Friday for the very chips.
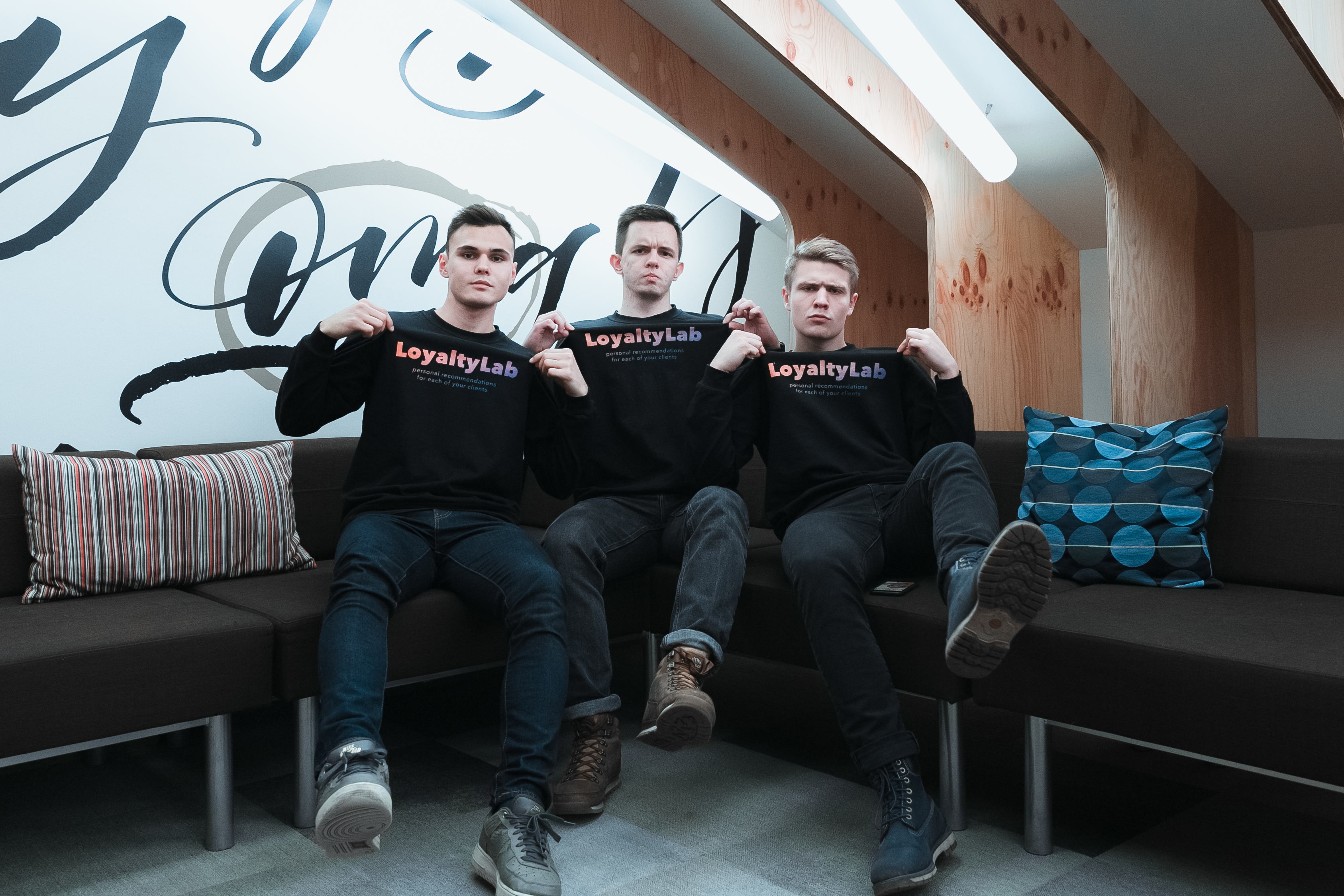
And it all went so far that I speak at NVIDIA GTC with material on the technical side of the product . We are happy to share our best practices with the community, so I am posting my report in the form of an article.
Introduction
Like everything at the beginning of the journey, we started with a review of how recommendation systems are made. And the most popular was the architecture of the following type:
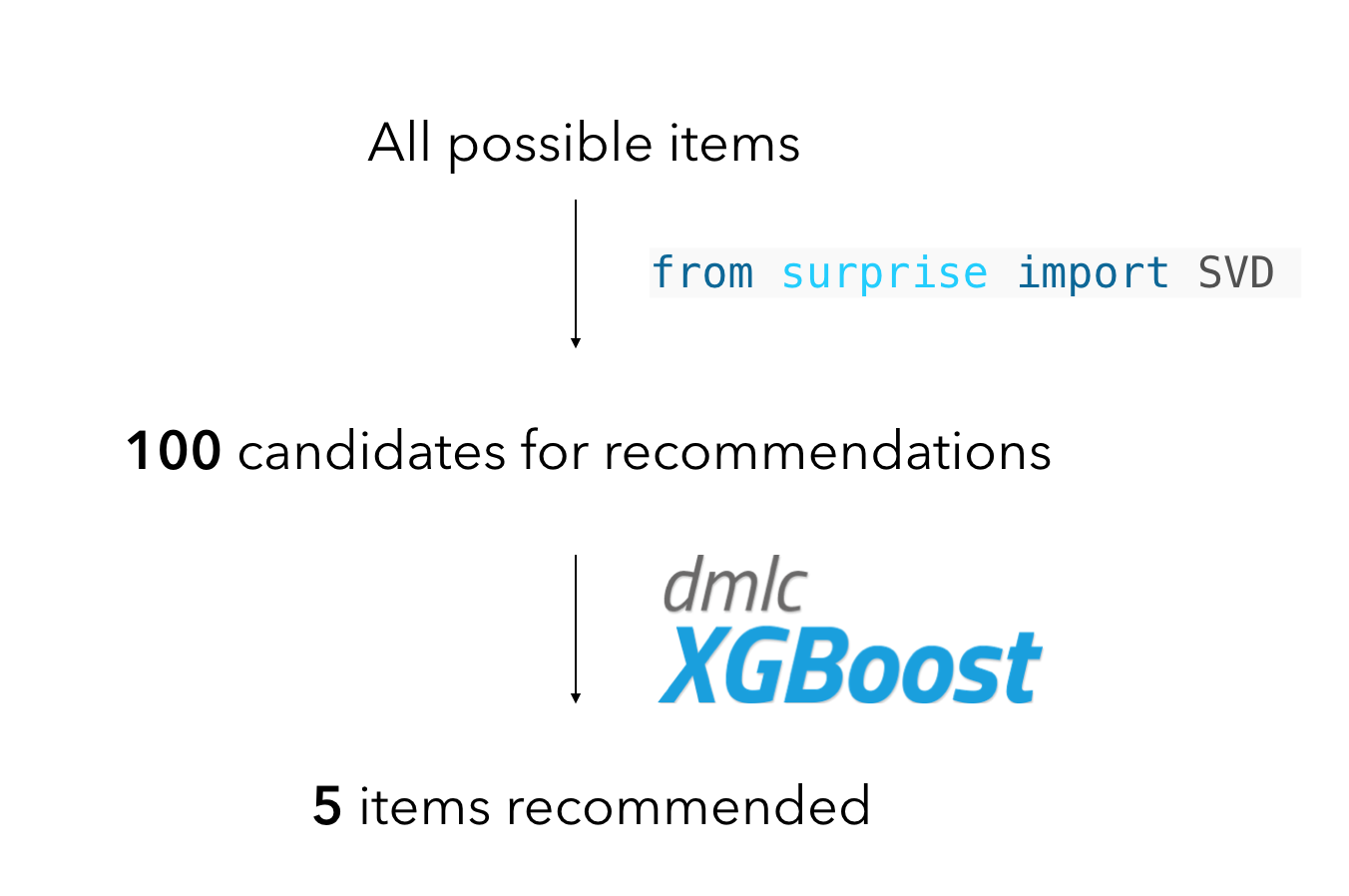
:
- , .
- c .
:
- candidate/ β user-product, .
- candidates extraction/extractor/ β β β .
. β ALS. , , . , , . , ALS, baselineβ.
, , , realtime , , 30 , , , . , , , , β , .
baseline , ALS . , , :
- Precision β .
- Recall β , target .
- F1-score β F-, .
. 3 :
- precision@5 β -5 .
- response-rate@5 β ( 5 ).
- avg roc-auc per user β roc-auc .
, time-series -, k , test k+1 . / . -, β . , .
, , baseline, ALS .
:
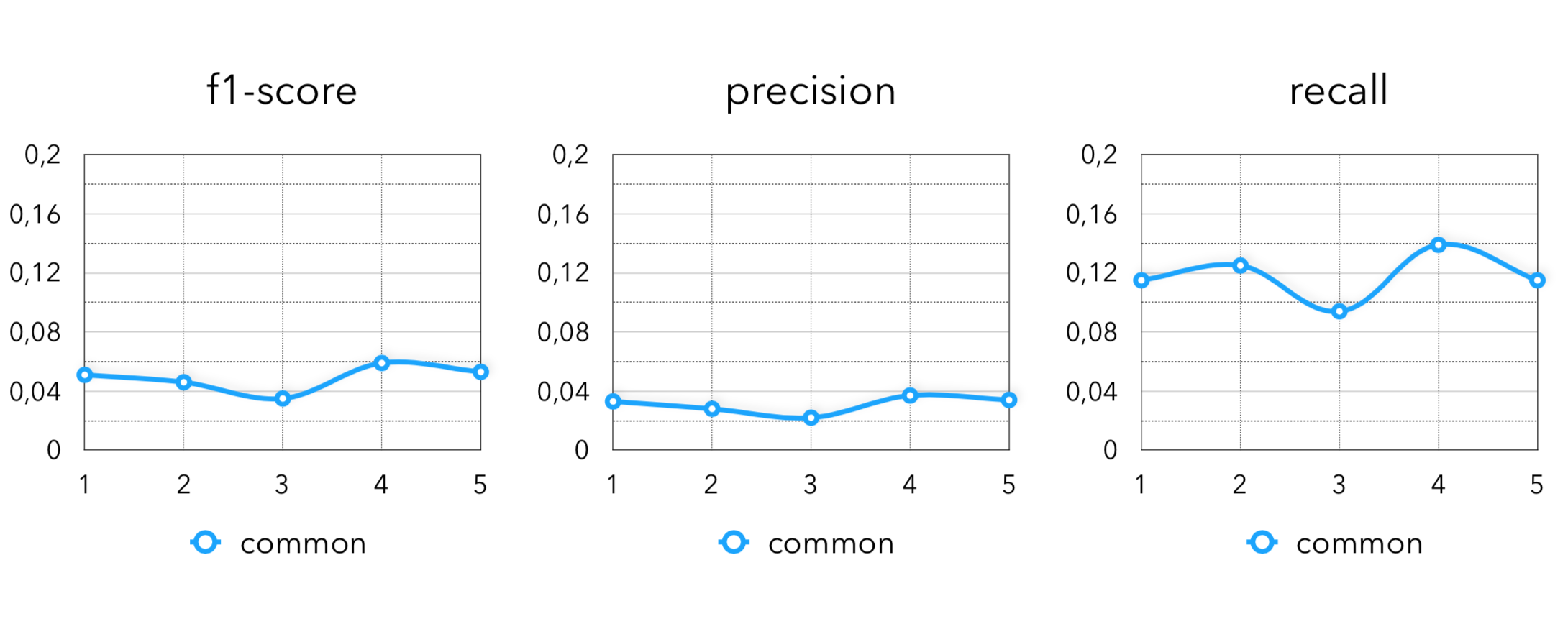
:
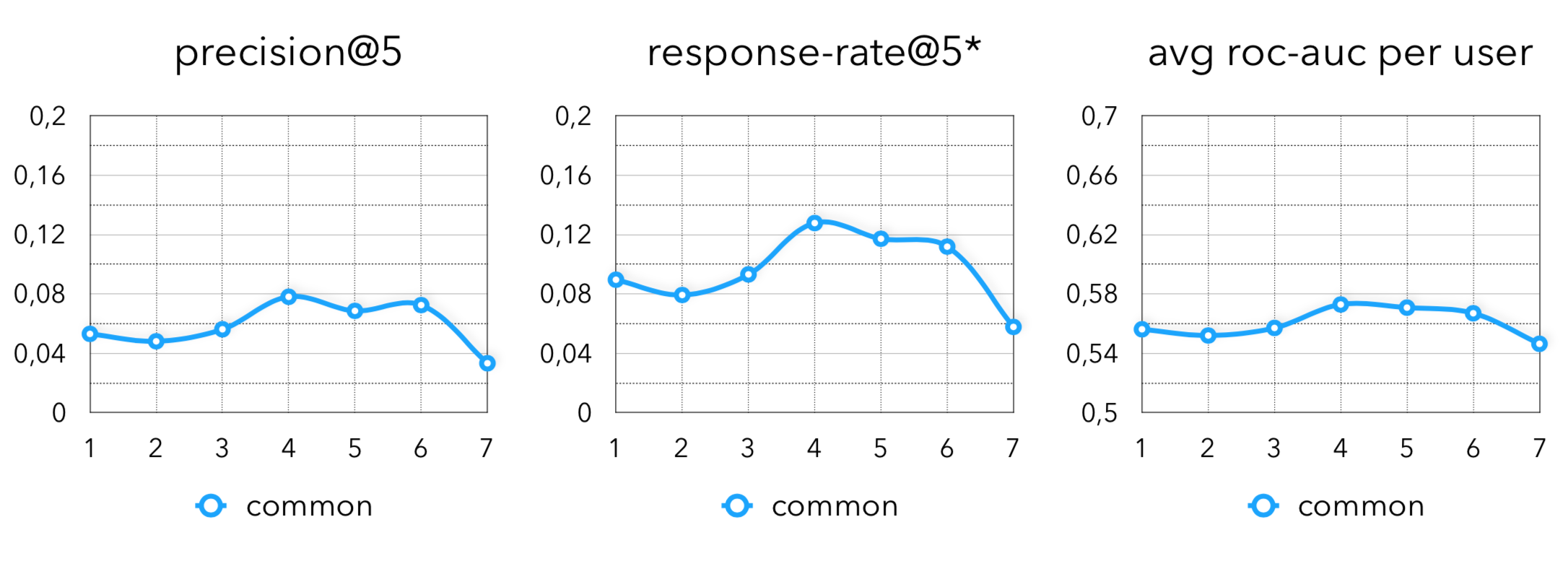
, . , , , , β , β. , , :
- .
- , .
- .
- /.
- ( ).
- , , (Word2Vec, DSSM, etc.).
, . , K ( 1-3 ), K . , :
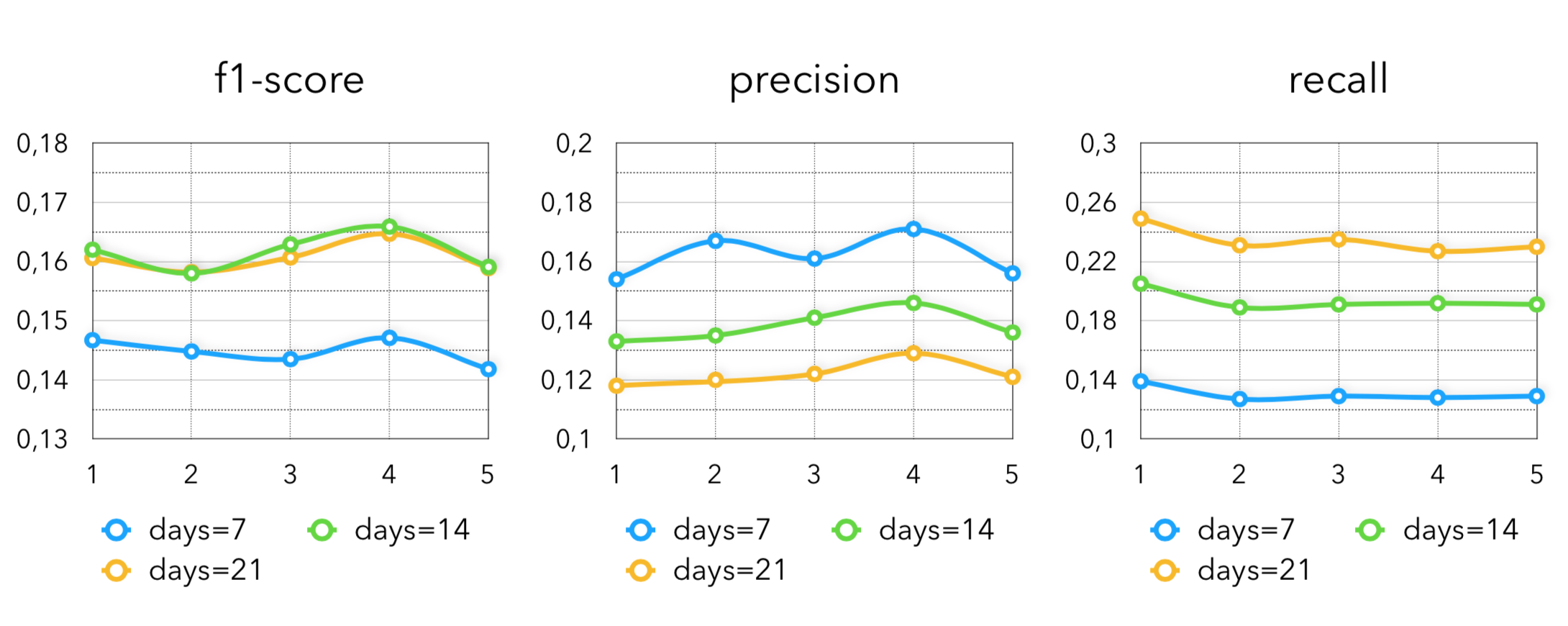
, , , recall precision . β 2 β.
,
, β β , , , - . . , ALS, , . β β , , / , , , , , . 2 :

k β , 14 .
, , .
, - . , //. , - . β , k . , . :
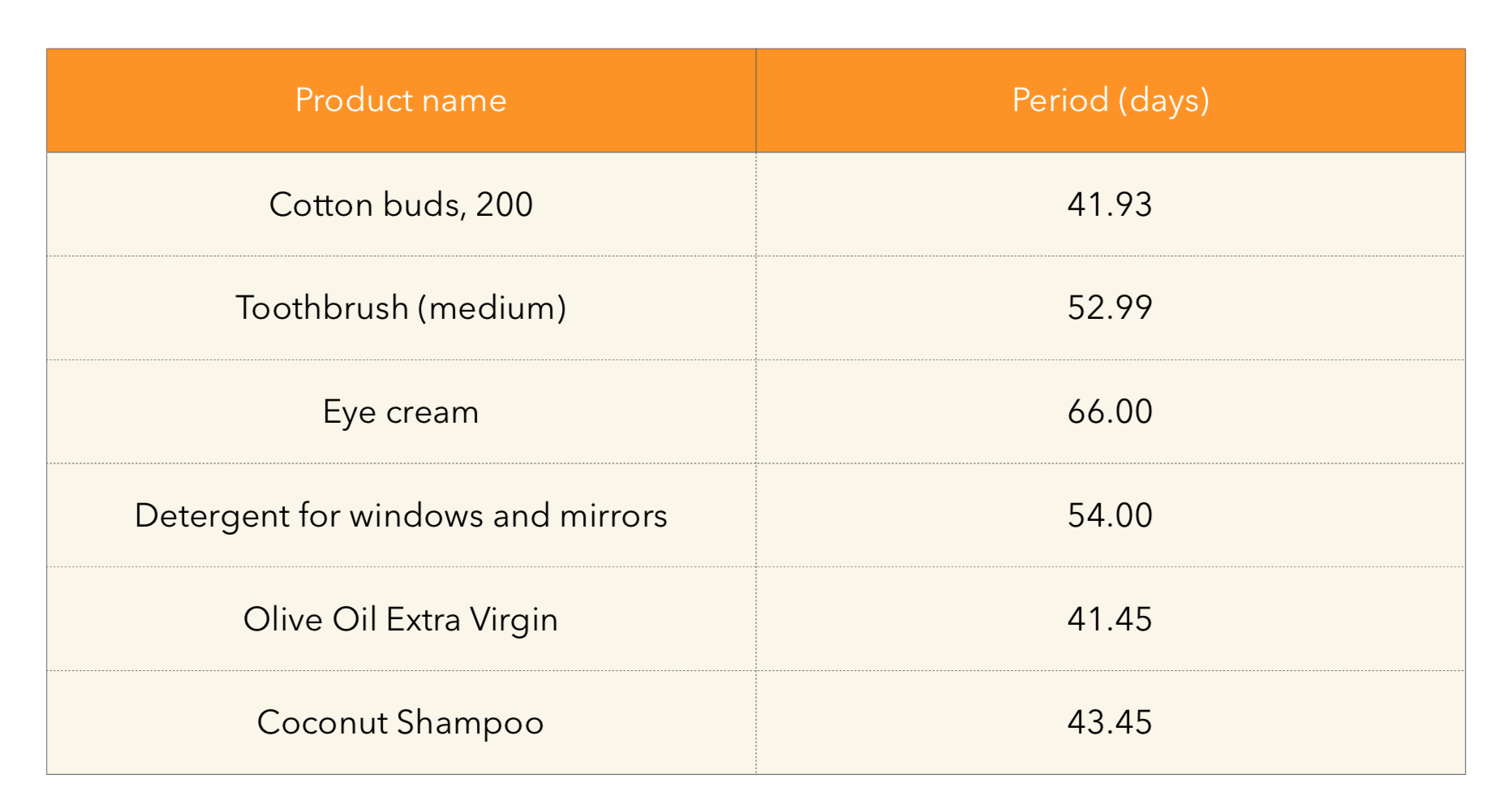
, , , . :
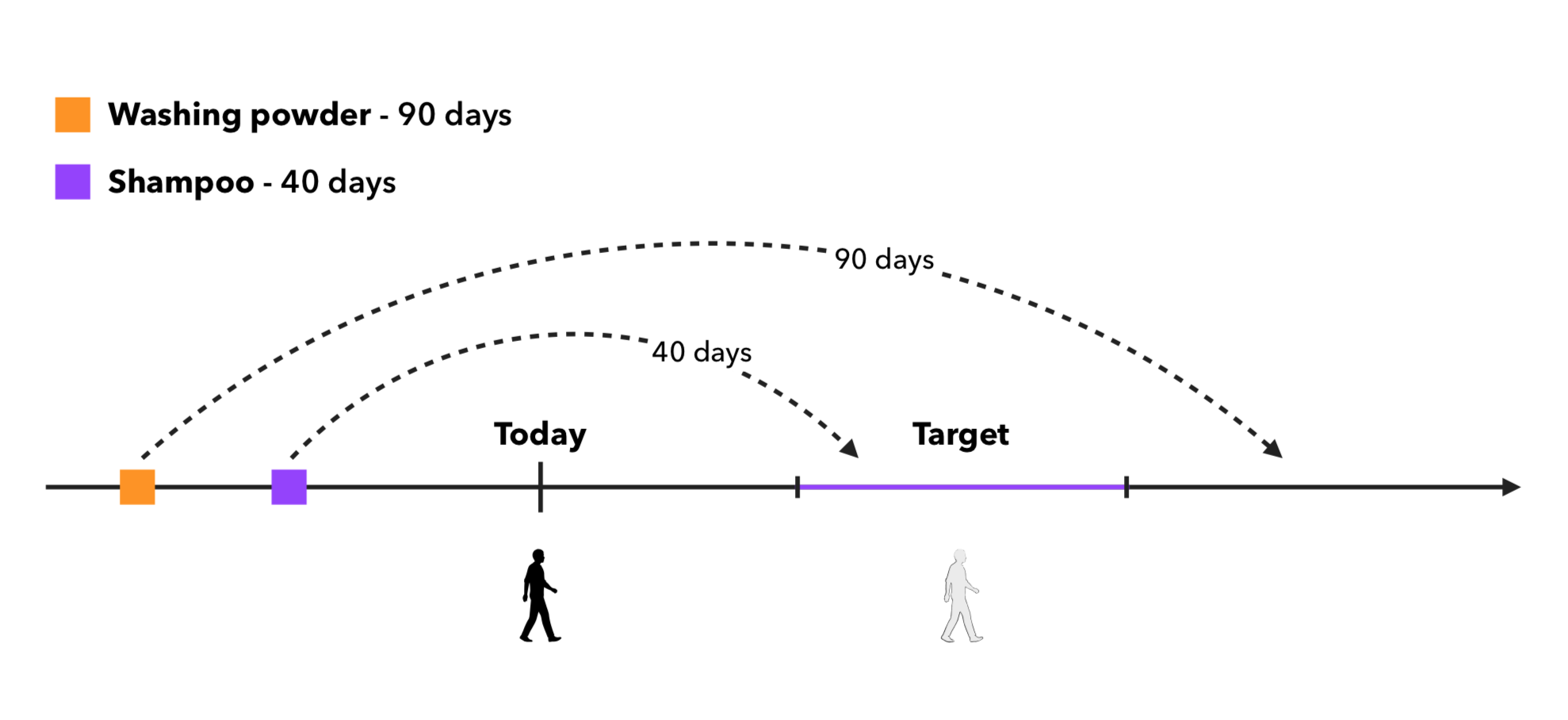
2 , :
- , , K .
- , .
, :

ft β , K ( K=5)
tm β ,
, (0, 0) recall precision, . , , k , .
β , . -k ββ / ββ /. ββ ββ . . , , , . . :
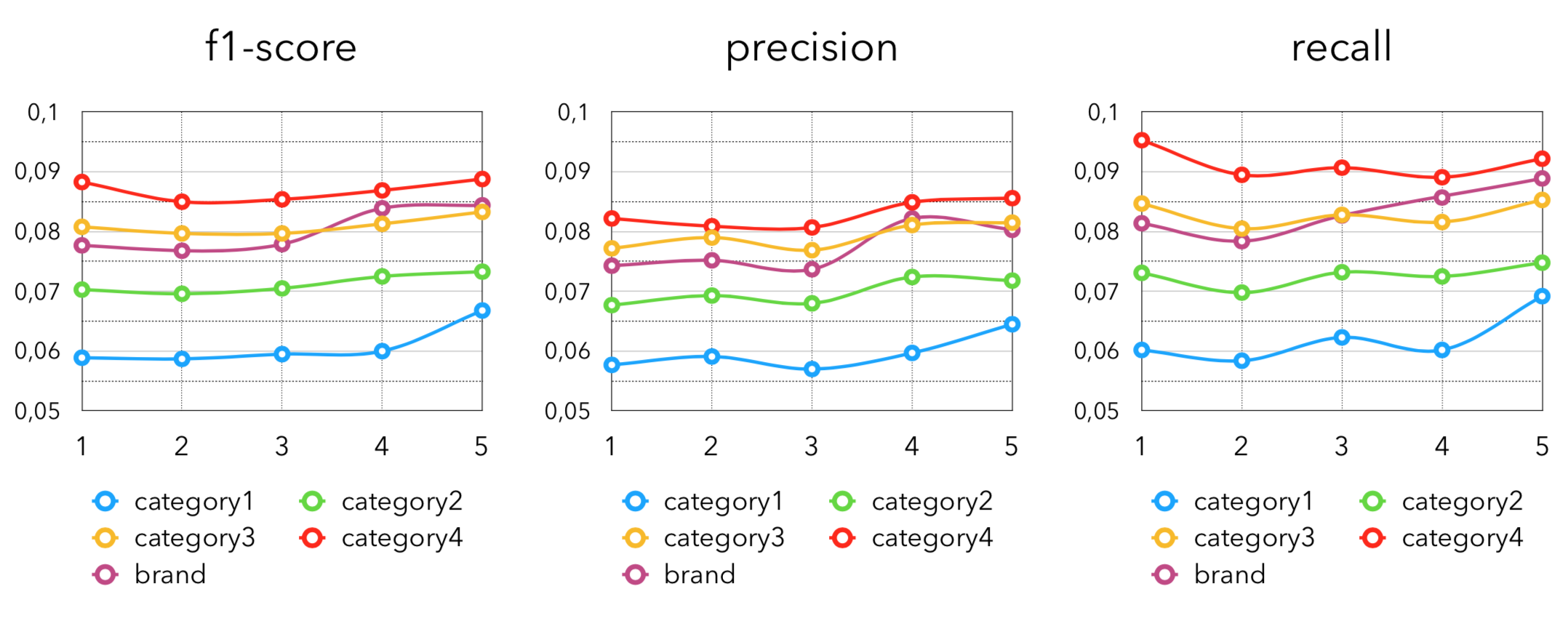
βcategoryβ .
, , , ββ .
, β . 2 , [ i]-[ j], j > i, . producti-productj , producti . -k , . :
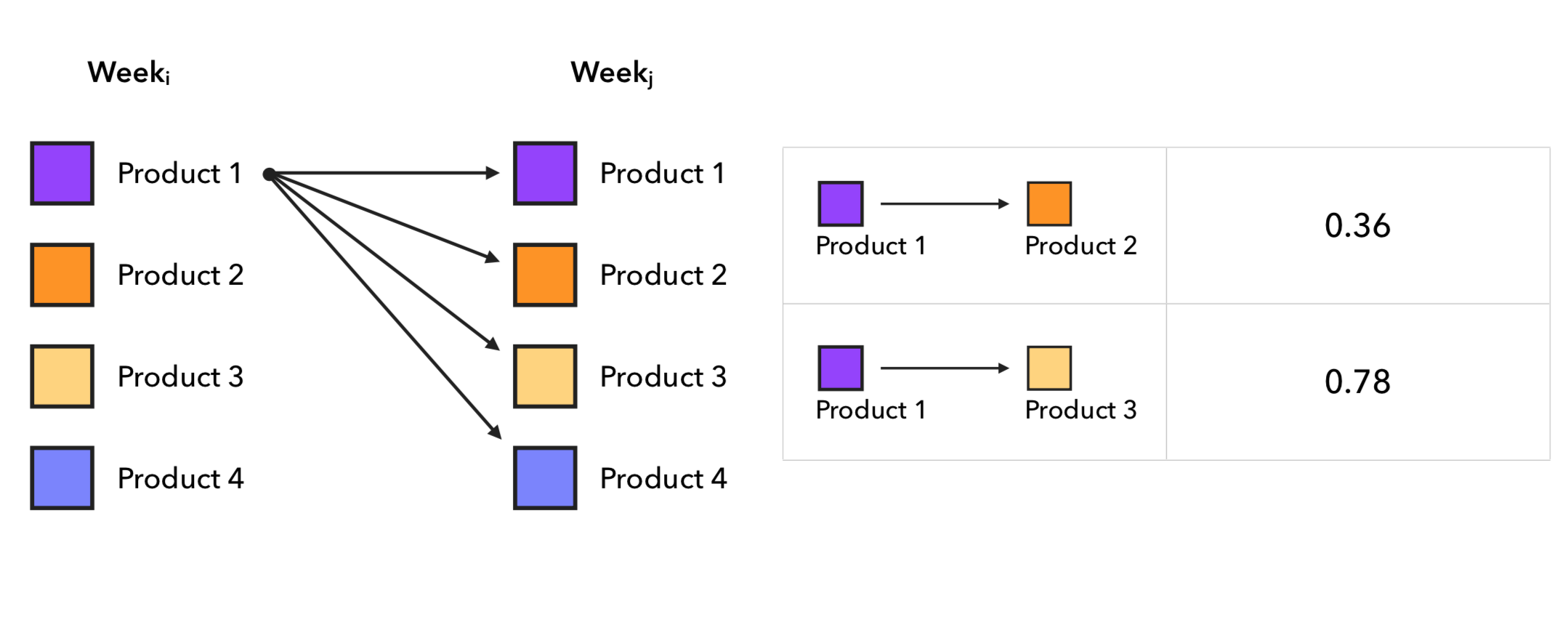
:
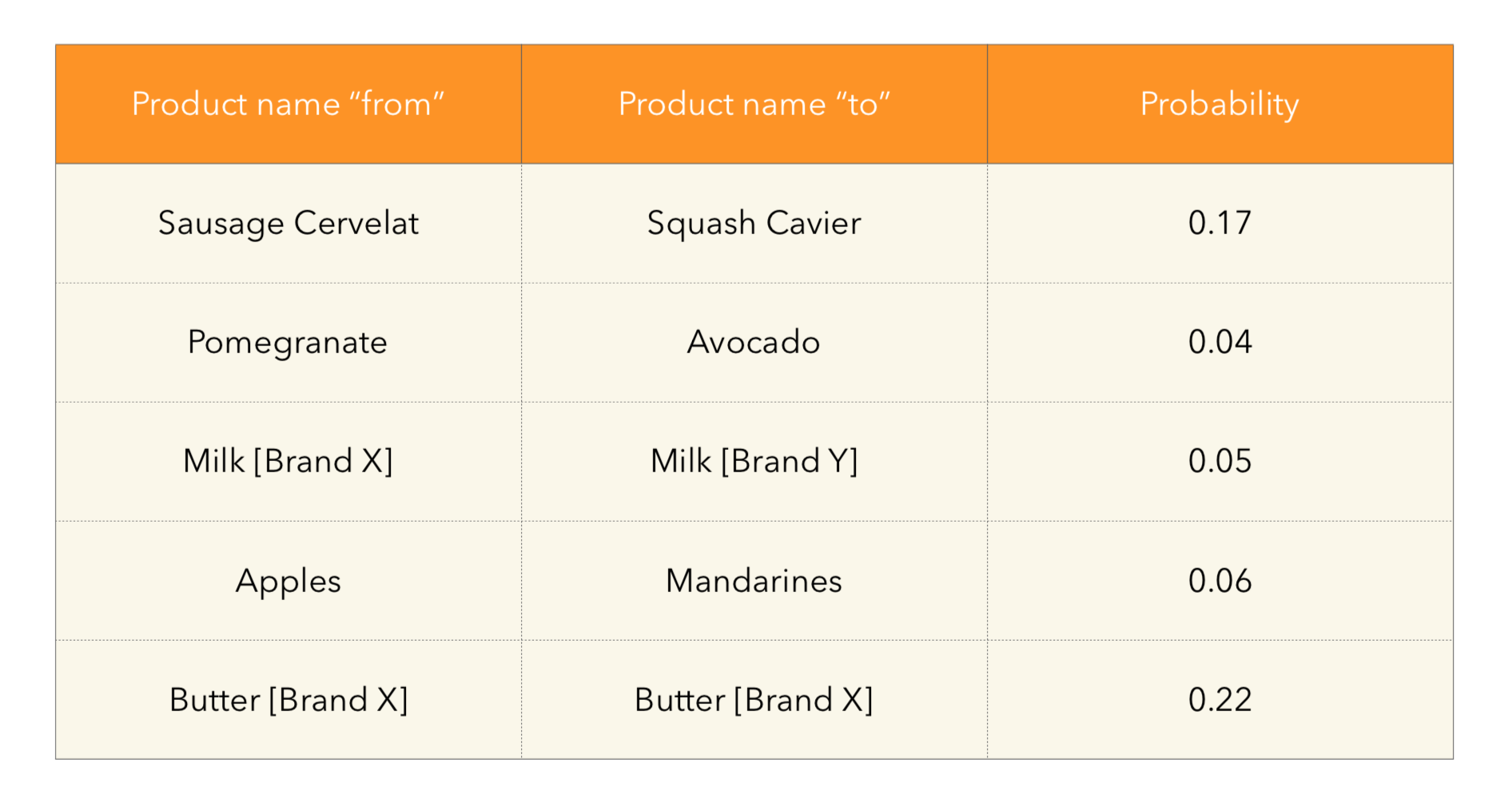
, : , , . , , , .
:

k β , .
, k=4. 4 .
, ,
β , . 3:
- ALS
- Word2Vec (Item2Vec )
- DSSM
ALS , , . Word2Vec, gensim. , , . , , ββ ( ). ecommerce , Ozon. DSSM . Microsoft, , research paper. :
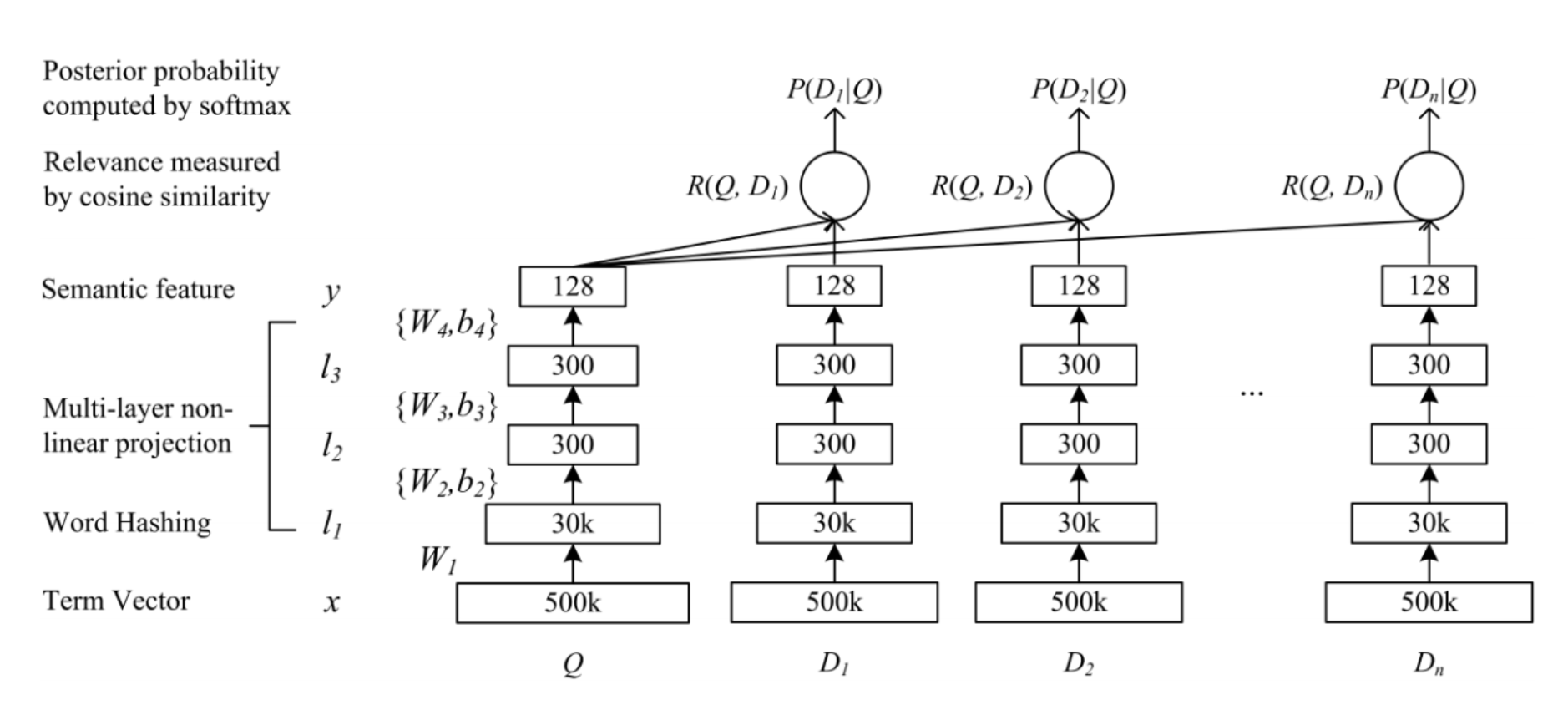
Q β query, , D[i] β document, -. , . (multilayer perceptron). , .
, , β . :

β ALS DSSM , Word2Vec . 3 :
- , , .
- .
- TF-IDF .
, , , , . 1, , Β½, β
, .:
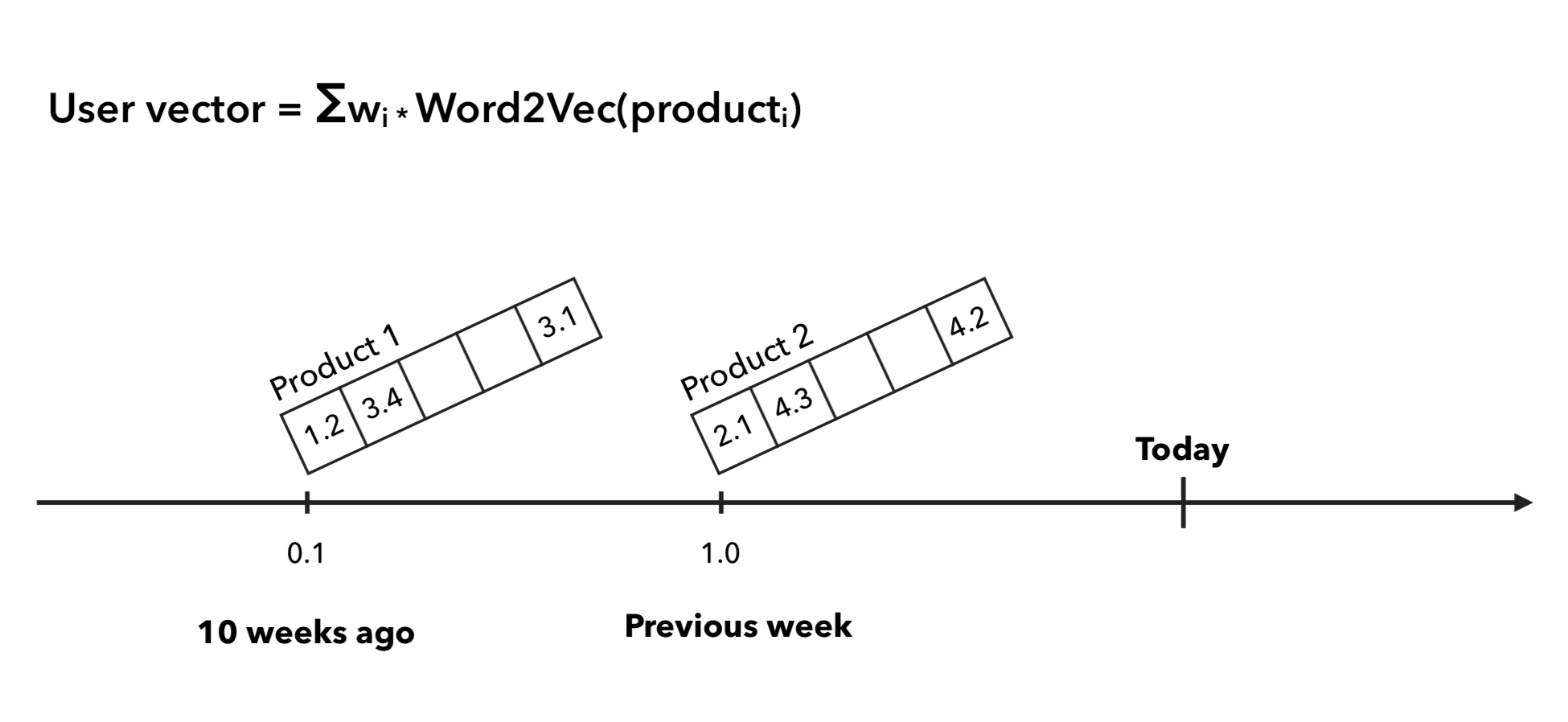
TF-IDF , TF-IDF , , , , β . , . :
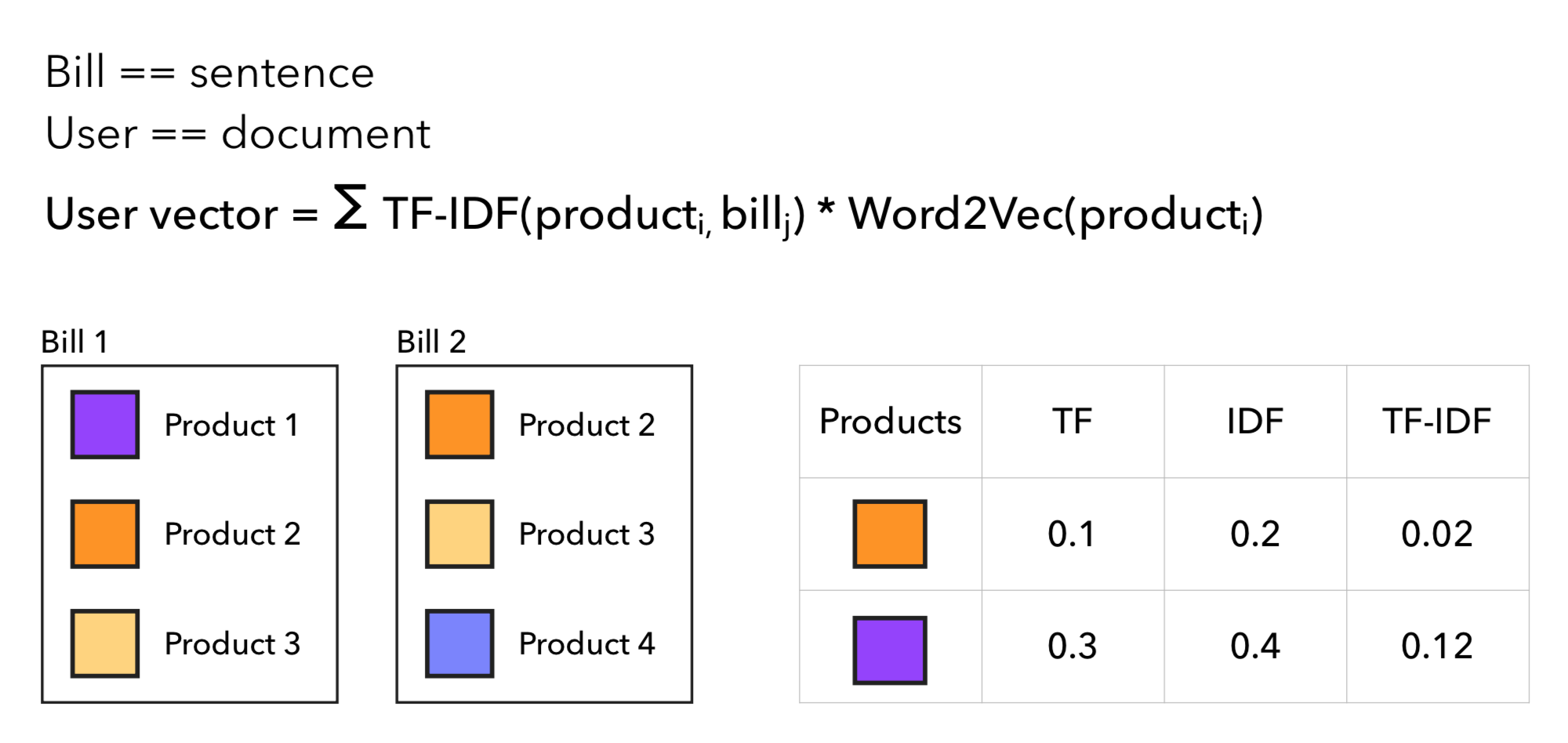
. ALS:
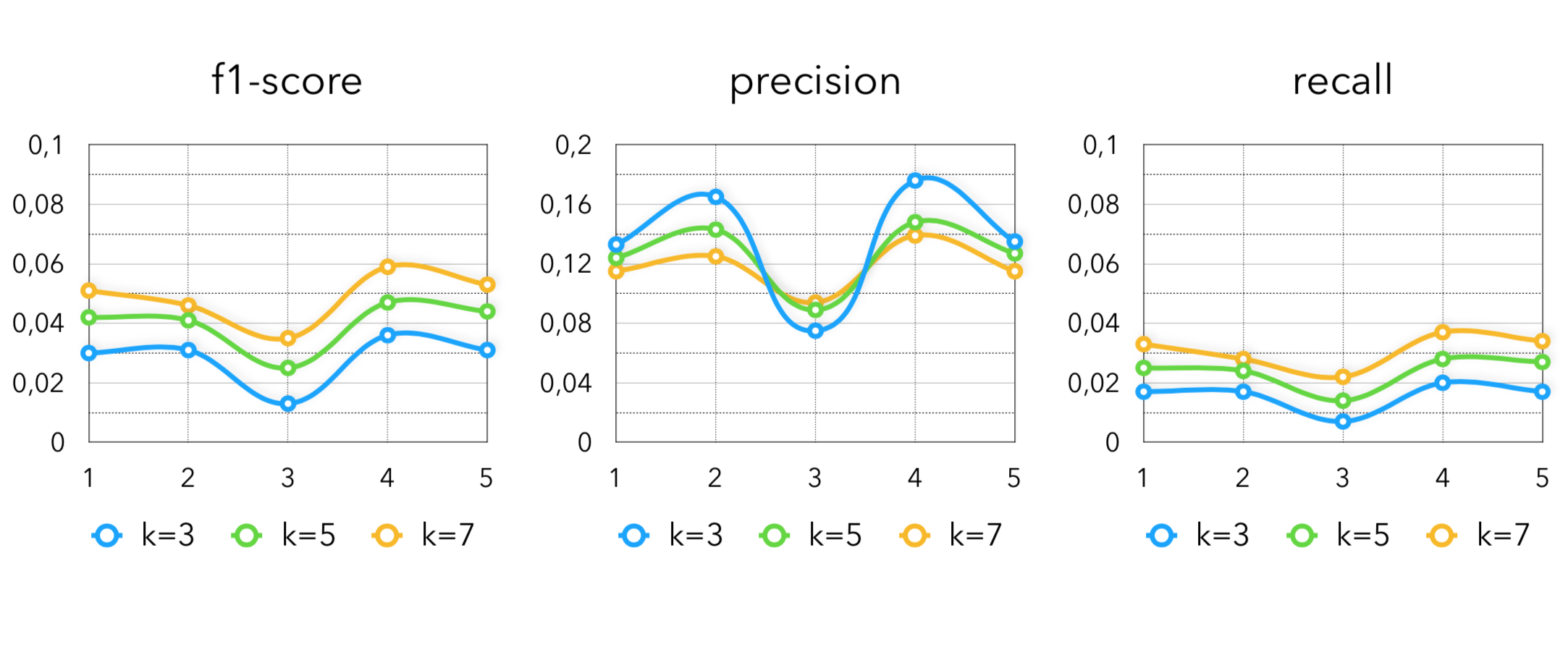
Item2Vec :
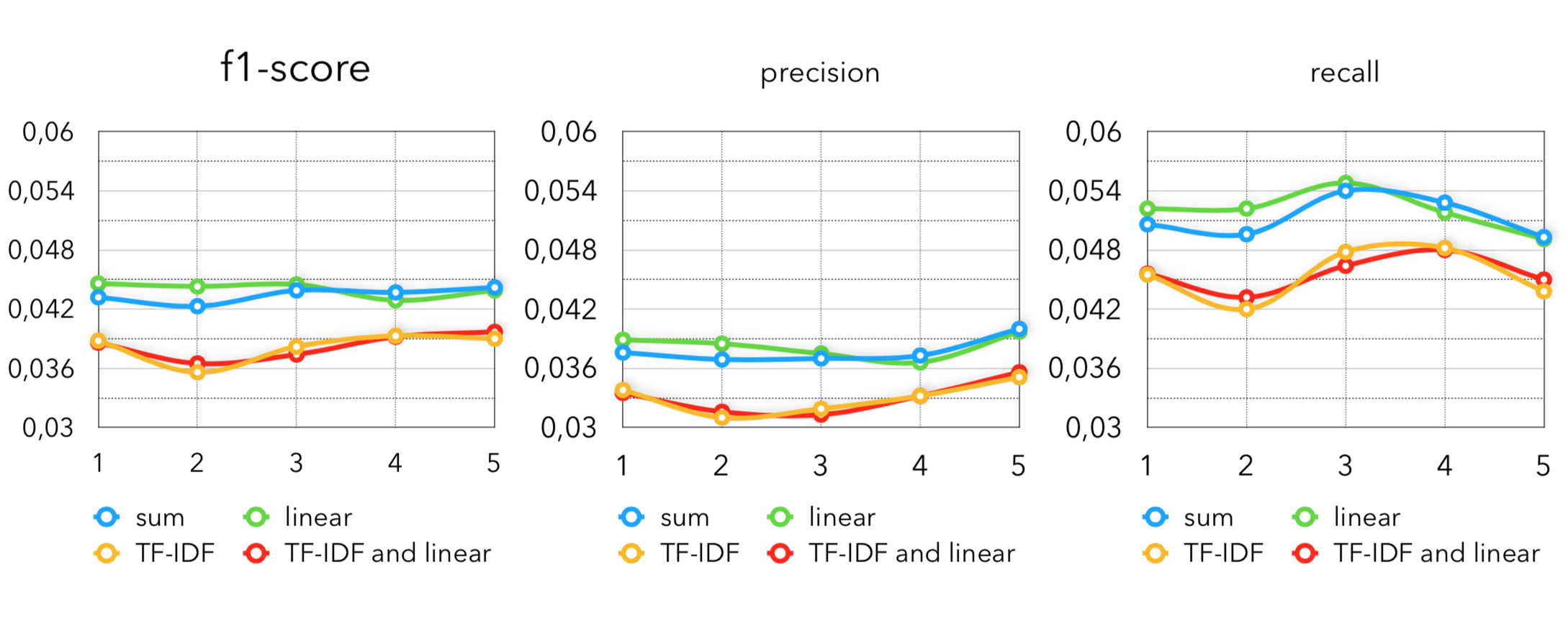
, baseline. , k . , , 50-70 .
DSSM:

?
, , ? ? :
- - . , , , .
- ?
, : , . , , , β β β β.
, , : (recall). , , . .
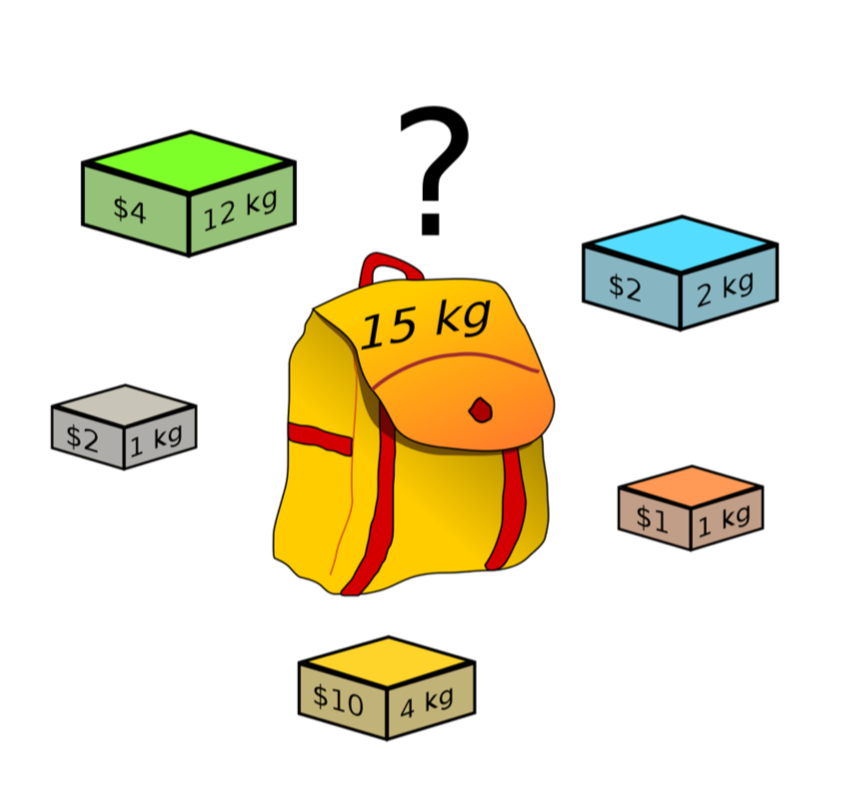
β , recall β . 2 , :
, Β«, Β» , . , β β . , . , .
:

:
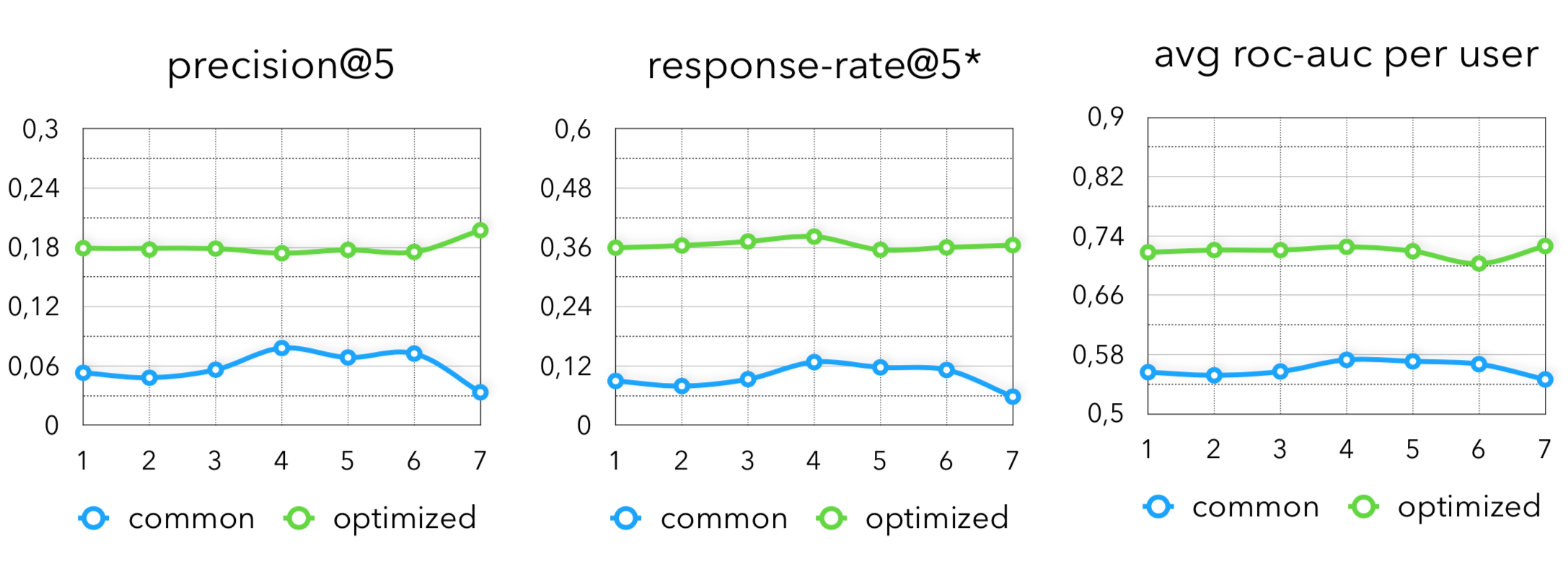
, , . , , , . , . , , :
- / .
- .
- .
, , . , . . , :
- / .
- , . , -.
- , , .
:
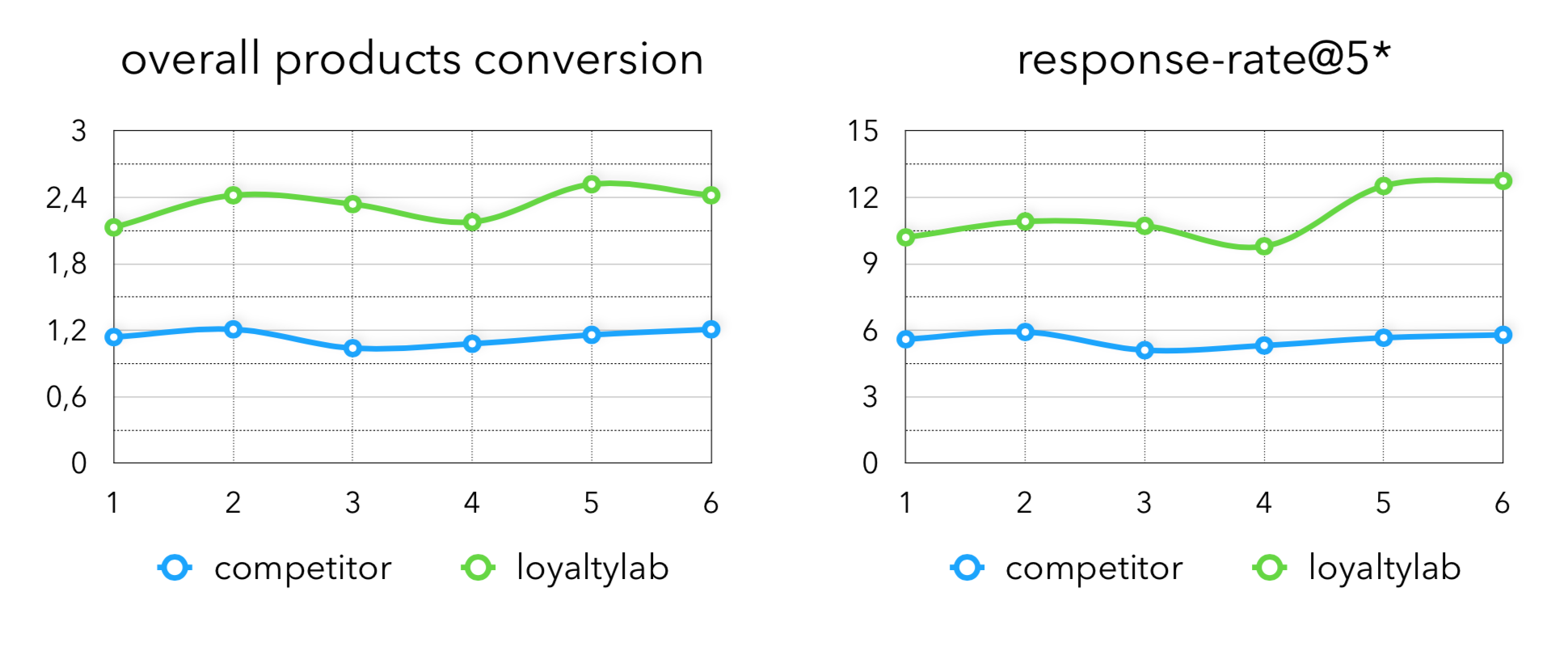
overall products conversion β , .
. , , , . , , .
, , , , β 3-4%:
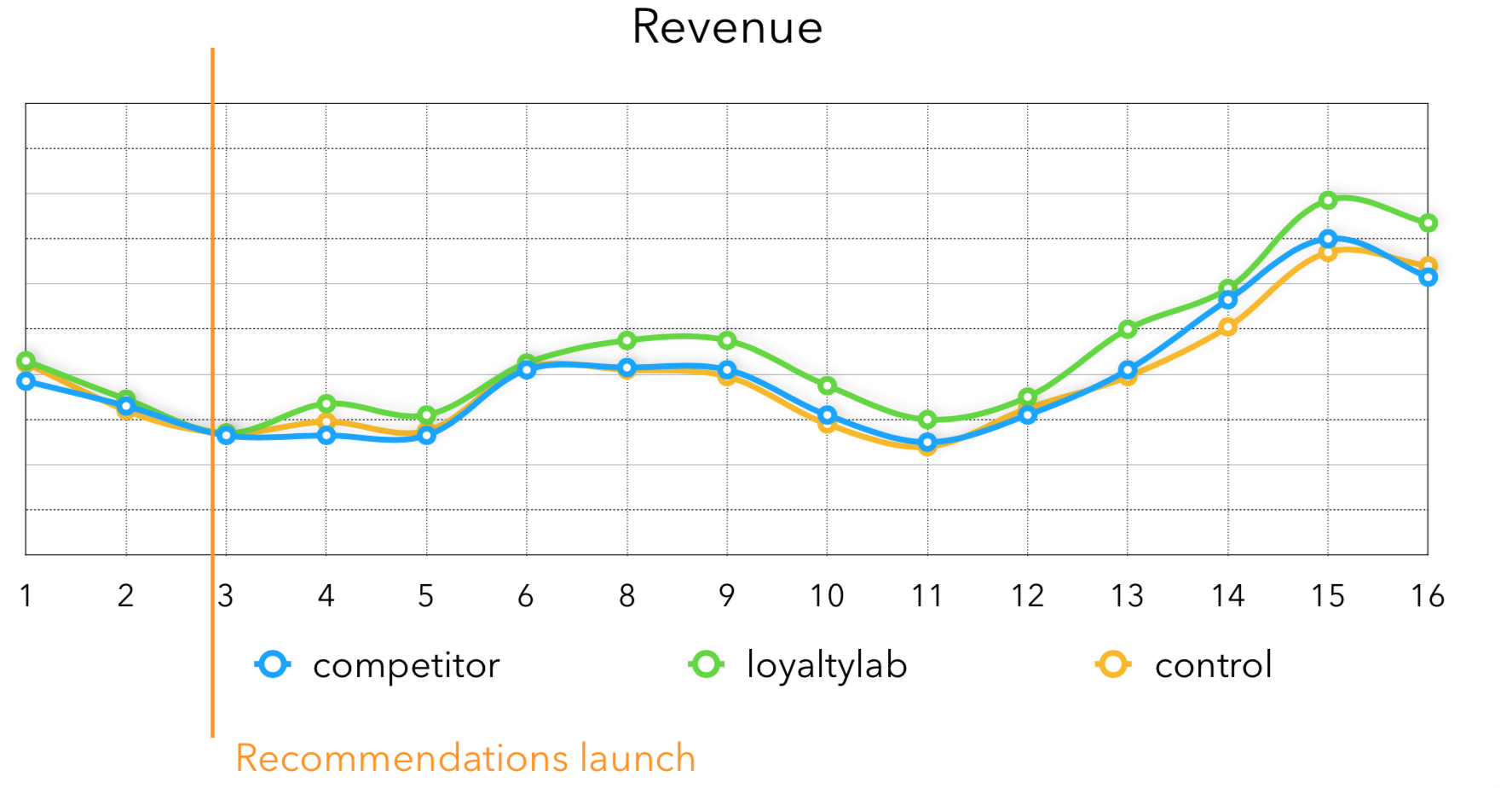
, , realtime , . , .
, . telegram. AI/ telegram β welcome :)