Neural networks are quite popular. Their main advantage is that they are able to generalize rather complex data on which other algorithms show low quality. But what if the quality of the neural network is still unsatisfactory?
And here ensembles come to the rescue ...
What are ensembles
An ensemble of machine learning algorithms is the use of several (not necessarily different) models instead of one. That is, we first train each model, and then combine their predictions. It turns out that our models together form one more complex (in terms of generalizing ability - the ability to "understand" data) model, which is often called a metamodel . Most often, the metamodel is no longer trained on our initial data sample, but on the predictions of other models. It seems to take into account the experience of all models, and this helps to reduce errors.
Plan
- First we look at one simple PyTorch model and get its quality.
- Then we will assemble several models using Scikit-learn and learn how to achieve a higher level
One single model
, Digits Sklearn, :
from sklearn.datasets import load_digits
import numpy as np
import matplotlib.pyplot as plt
x, y = load_digits(n_class=10, return_X_y=True)
, :
print(x.shape)
>>> (1797, 64)
print(np.unique(y))
>>> array([0, 1, 2, 3, 4, 5, 6, 7, 8, 9])
index = 0
print(y[index])
plt.imshow(x[index].reshape(8, 8), cmap="Greys")
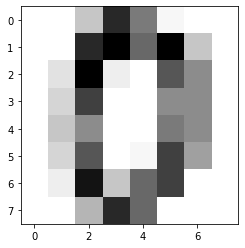
x[index]
>>> array([ 0., 0., 5., 13., 9., 1., 0., 0., 0., 0., 13., 15., 10.,
15., 5., 0., 0., 3., 15., 2., 0., 11., 8., 0., 0., 4.,
12., 0., 0., 8., 8., 0., 0., 5., 8., 0., 0., 9., 8.,
0., 0., 4., 11., 0., 1., 12., 7., 0., 0., 2., 14., 5.,
10., 12., 0., 0., 0., 0., 6., 13., 10., 0., 0., 0.])
, 0 15. , :
from sklearn.model_selection import train_test_split
from sklearn.preprocessing import StandardScaler
x_train, x_test, y_train, y_test = train_test_split(x, y, test_size=0.2,
shuffle=True, random_state=42)
print(x_train.shape)
>>> (1437, 64)
print(x_test.shape)
>>> (360, 64)
StandardScaler: -1 1. , — score score' :
scaler = StandardScaler()
scaler.fit(x_train)
:
x_train_scaled = scaler.transform(x_train)
x_test_scaled = scaler.transform(x_test)
Torch'!
import torch
from torch.utils.data import TensorDataset, DataLoader
import torch.nn as nn
import torch.nn.functional as F
from torch.optim import Adam
:
class SimpleCNN(nn.Module):
def __init__(self):
super(SimpleCNN, self).__init__()
self.conv1 = nn.Conv2d(in_channels=1, out_channels=3, kernel_size=5, stride=1, padding=2)
self.conv1_s = nn.Conv2d(in_channels=3, out_channels=3, kernel_size=5, stride=2, padding=2)
self.conv2 = nn.Conv2d(in_channels=3, out_channels=6, kernel_size=3, stride=1, padding=1)
self.conv2_s = nn.Conv2d(in_channels=6, out_channels=6, kernel_size=3, stride=2, padding=1)
self.conv3 = nn.Conv2d(in_channels=6, out_channels=10, kernel_size=3, stride=1, padding=1)
self.conv3_s = nn.Conv2d(in_channels=10, out_channels=10, kernel_size=3, stride=2, padding=1)
self.flatten = nn.Flatten()
self.fc1 = nn.Linear(10, 10)
def forward(self, x):
x = F.relu(self.conv1(x))
x = F.relu(self.conv1_s(x))
x = F.relu(self.conv2(x))
x = F.relu(self.conv2_s(x))
x = F.relu(self.conv3(x))
x = F.relu(self.conv3_s(x))
x = self.flatten(x)
x = self.fc1(x)
x = F.softmax(x)
return x
:
batch_size = 64
learning_rate = 1e-3
epochs = 200
, PyTorch:
x_train_tensor = torch.tensor(x_train_scaled.reshape(-1, 1, 8, 8).astype(np.float32))
x_test_tensor = torch.tensor(x_test_scaled.reshape(-1, 1, 8, 8).astype(np.float32))
y_train_tensor = torch.tensor(y_train.astype(np.long))
y_test_tensor = torch.tensor(y_test.astype(np.long))
PyTorch , — . — , X y ( ), DataLoader — , <X, y> 64 :
train_dataset = TensorDataset(x_train_tensor, y_train_tensor)
train_loader = DataLoader(train_dataset, batch_size=batch_size)
test_dataset = TensorDataset(x_test_tensor, y_test_tensor)
test_loader = DataLoader(test_dataset, batch_size=batch_size)
, :
simple_cnn = SimpleCNN().cuda()
criterion = nn.CrossEntropyLoss()
optimizer = Adam(simple_cnn.parameters(), lr=learning_rate)
:
for epoch in range(epochs):
simple_cnn.train()
train_samples_count = 0
true_train_samples_count = 0
running_loss = 0
for batch in train_loader:
x_data = batch[0].cuda()
y_data = batch[1].cuda()
y_pred = simple_cnn(x_data)
loss = criterion(y_pred, y_data)
optimizer.zero_grad()
loss.backward()
optimizer.step()
running_loss += loss.item()
y_pred = y_pred.argmax(dim=1, keepdim=False)
true_classified = (y_pred == y_data).sum().item()
true_train_samples_count += true_classified
train_samples_count += len(x_data)
train_accuracy = true_train_samples_count / train_samples_count
print(f"[{epoch}] train loss: {running_loss}, accuracy: {round(train_accuracy, 4)}")
simple_cnn.eval()
test_samples_count = 0
true_test_samples_count = 0
running_loss = 0
for batch in test_loader:
x_data = batch[0].cuda()
y_data = batch[1].cuda()
y_pred = simple_cnn(x_data)
loss = criterion(y_pred, y_data)
loss.backward()
running_loss += loss.item()
y_pred = y_pred.argmax(dim=1, keepdim=False)
true_classified = (y_pred == y_data).sum().item()
true_test_samples_count += true_classified
test_samples_count += len(x_data)
test_accuracy = true_test_samples_count / test_samples_count
print(f"[{epoch}] test loss: {running_loss}, accuracy: {round(test_accuracy, 4)}")
,- Torch' , . . 20 .
[180] train loss: 40.52328181266785, accuracy: 0.6966
[180] test loss: 10.813781499862671, accuracy: 0.6583
[181] train loss: 40.517325043678284, accuracy: 0.6966
[181] test loss: 10.811877608299255, accuracy: 0.6611
[182] train loss: 40.517088294029236, accuracy: 0.6966
[182] test loss: 10.814386487007141, accuracy: 0.6611
[183] train loss: 40.515315651893616, accuracy: 0.6966
[183] test loss: 10.812204122543335, accuracy: 0.6611
[184] train loss: 40.5108939409256, accuracy: 0.6966
[184] test loss: 10.808713555335999, accuracy: 0.6639
[185] train loss: 40.50885498523712, accuracy: 0.6966
[185] test loss: 10.80833113193512, accuracy: 0.6639
[186] train loss: 40.50892996788025, accuracy: 0.6966
[186] test loss: 10.809209108352661, accuracy: 0.6639
[187] train loss: 40.508036971092224, accuracy: 0.6966
[187] test loss: 10.806900978088379, accuracy: 0.6667
[188] train loss: 40.507275462150574, accuracy: 0.6966
[188] test loss: 10.79791784286499, accuracy: 0.6611
[189] train loss: 40.50368785858154, accuracy: 0.6966
[189] test loss: 10.799399137496948, accuracy: 0.6667
[190] train loss: 40.499858379364014, accuracy: 0.6966
[190] test loss: 10.795265793800354, accuracy: 0.6611
[191] train loss: 40.498780846595764, accuracy: 0.6966
[191] test loss: 10.796114206314087, accuracy: 0.6639
[192] train loss: 40.497228503227234, accuracy: 0.6966
[192] test loss: 10.790620803833008, accuracy: 0.6639
[193] train loss: 40.44325613975525, accuracy: 0.6973
[193] test loss: 10.657087206840515, accuracy: 0.7
[194] train loss: 39.62049174308777, accuracy: 0.7495
[194] test loss: 10.483307123184204, accuracy: 0.7222
[195] train loss: 39.24516046047211, accuracy: 0.7613
[195] test loss: 10.462445378303528, accuracy: 0.7278
[196] train loss: 39.16947162151337, accuracy: 0.762
[196] test loss: 10.488057255744934, accuracy: 0.7222
[197] train loss: 39.196797251701355, accuracy: 0.7634
[197] test loss: 10.502906918525696, accuracy: 0.7222
[198] train loss: 39.395434617996216, accuracy: 0.7537
[198] test loss: 10.354896545410156, accuracy: 0.7472
[199] train loss: 39.331292152404785, accuracy: 0.7509
[199] test loss: 10.367400050163269, accuracy: 0.7389
, : .
, : 74% . Not great, not terrible.
!
Bagging. : , , . : "" , - . .
sklearn ensembles
, . — , BaggingClassifier
:
import sklearn
from sklearn.ensemble import BaggingClassifier
. sklearn, — , sklearn.base.BaseEstimator
. , . fit
( ), predict_proba
, ( , ), predict
( ), .
, "" .
. , , .
-, ( 2 — get_params
set_params
), , . , net_type
, __init__
net_type
.
-, , , ( sklearn , ) copy
, deepcopy
. , , () , , .
class PytorchModel(sklearn.base.BaseEstimator):
def __init__(self, net_type, net_params, optim_type, optim_params, loss_fn,
input_shape, batch_size=32, accuracy_tol=0.02, tol_epochs=10,
cuda=True):
self.net_type = net_type
self.net_params = net_params
self.optim_type = optim_type
self.optim_params = optim_params
self.loss_fn = loss_fn
self.input_shape = input_shape
self.batch_size = batch_size
self.accuracy_tol = accuracy_tol
self.tol_epochs = tol_epochs
self.cuda = cuda
: fit
. , — , Loss, . : , ? . , 200 ( ). , . — , accuracy . , accuracy . , (tol_epochs
) ( accuracy , accuracy_tol
).
def fit(self, X, y):
self.net = self.net_type(**self.net_params)
if self.cuda:
self.net = self.net.cuda()
self.optim = self.optim_type(self.net.parameters(), **self.optim_params)
uniq_classes = np.sort(np.unique(y))
self.classes_ = uniq_classes
X = X.reshape(-1, *self.input_shape)
x_tensor = torch.tensor(X.astype(np.float32))
y_tensor = torch.tensor(y.astype(np.long))
train_dataset = TensorDataset(x_tensor, y_tensor)
train_loader = DataLoader(train_dataset, batch_size=self.batch_size,
shuffle=True, drop_last=False)
last_accuracies = []
epoch = 0
keep_training = True
while keep_training:
self.net.train()
train_samples_count = 0
true_train_samples_count = 0
for batch in train_loader:
x_data, y_data = batch[0], batch[1]
if self.cuda:
x_data = x_data.cuda()
y_data = y_data.cuda()
y_pred = self.net(x_data)
loss = self.loss_fn(y_pred, y_data)
self.optim.zero_grad()
loss.backward()
self.optim.step()
y_pred = y_pred.argmax(dim=1, keepdim=False)
true_classified = (y_pred == y_data).sum().item()
true_train_samples_count += true_classified
train_samples_count += len(x_data)
train_accuracy = true_train_samples_count / train_samples_count
last_accuracies.append(train_accuracy)
if len(last_accuracies) > self.tol_epochs:
last_accuracies.pop(0)
if len(last_accuracies) == self.tol_epochs:
accuracy_difference = max(last_accuracies) - min(last_accuracies)
if accuracy_difference <= self.accuracy_tol:
keep_training = False
. — , Loss, - :
def predict_proba(self, X, y=None):
X = X.reshape(-1, *self.input_shape)
x_tensor = torch.tensor(X.astype(np.float32))
if y:
y_tensor = torch.tensor(y.astype(np.long))
else:
y_tensor = torch.zeros(len(X), dtype=torch.long)
test_dataset = TensorDataset(x_tensor, y_tensor)
test_loader = DataLoader(test_dataset, batch_size=self.batch_size,
shuffle=False, drop_last=False)
self.net.eval()
predictions = []
for batch in test_loader:
x_data, y_data = batch[0], batch[1]
if self.cuda:
x_data = x_data.cuda()
y_data = y_data.cuda()
y_pred = self.net(x_data)
predictions.append(y_pred.detach().cpu().numpy())
predictions = np.concatenate(predictions)
return predictions
:
def predict(self, X, y=None):
predictions = self.predict_proba(X, y)
predictions = predictions.argmax(axis=1)
return predictions
class PytorchModel(sklearn.base.BaseEstimator):
def __init__(self, net_type, net_params, optim_type, optim_params, loss_fn,
input_shape, batch_size=32, accuracy_tol=0.02, tol_epochs=10,
cuda=True):
self.net_type = net_type
self.net_params = net_params
self.optim_type = optim_type
self.optim_params = optim_params
self.loss_fn = loss_fn
self.input_shape = input_shape
self.batch_size = batch_size
self.accuracy_tol = accuracy_tol
self.tol_epochs = tol_epochs
self.cuda = cuda
def fit(self, X, y):
self.net = self.net_type(**self.net_params)
if self.cuda:
self.net = self.net.cuda()
self.optim = self.optim_type(self.net.parameters(), **self.optim_params)
uniq_classes = np.sort(np.unique(y))
self.classes_ = uniq_classes
X = X.reshape(-1, *self.input_shape)
x_tensor = torch.tensor(X.astype(np.float32))
y_tensor = torch.tensor(y.astype(np.long))
train_dataset = TensorDataset(x_tensor, y_tensor)
train_loader = DataLoader(train_dataset, batch_size=self.batch_size,
shuffle=True, drop_last=False)
last_accuracies = []
epoch = 0
keep_training = True
while keep_training:
self.net.train()
train_samples_count = 0
true_train_samples_count = 0
for batch in train_loader:
x_data, y_data = batch[0], batch[1]
if self.cuda:
x_data = x_data.cuda()
y_data = y_data.cuda()
y_pred = self.net(x_data)
loss = self.loss_fn(y_pred, y_data)
self.optim.zero_grad()
loss.backward()
self.optim.step()
y_pred = y_pred.argmax(dim=1, keepdim=False)
true_classified = (y_pred == y_data).sum().item()
true_train_samples_count += true_classified
train_samples_count += len(x_data)
train_accuracy = true_train_samples_count / train_samples_count
last_accuracies.append(train_accuracy)
if len(last_accuracies) > self.tol_epochs:
last_accuracies.pop(0)
if len(last_accuracies) == self.tol_epochs:
accuracy_difference = max(last_accuracies) - min(last_accuracies)
if accuracy_difference <= self.accuracy_tol:
keep_training = False
def predict_proba(self, X, y=None):
X = X.reshape(-1, *self.input_shape)
x_tensor = torch.tensor(X.astype(np.float32))
if y:
y_tensor = torch.tensor(y.astype(np.long))
else:
y_tensor = torch.zeros(len(X), dtype=torch.long)
test_dataset = TensorDataset(x_tensor, y_tensor)
test_loader = DataLoader(test_dataset, batch_size=self.batch_size,
shuffle=False, drop_last=False)
self.net.eval()
predictions = []
for batch in test_loader:
x_data, y_data = batch[0], batch[1]
if self.cuda:
x_data = x_data.cuda()
y_data = y_data.cuda()
y_pred = self.net(x_data)
predictions.append(y_pred.detach().cpu().numpy())
predictions = np.concatenate(predictions)
return predictions
def predict(self, X, y=None):
predictions = self.predict_proba(X, y)
predictions = predictions.argmax(axis=1)
return predictions
, :
base_model = PytorchModel(net_type=SimpleCNN, net_params=dict(), optim_type=Adam,
optim_params={"lr": 1e-3}, loss_fn=nn.CrossEntropyLoss(),
input_shape=(1, 8, 8), batch_size=32, accuracy_tol=0.02,
tol_epochs=10, cuda=True)
base_model.fit(x_train_scaled, y_train)
preds = base_model.predict(x_test_scaled)
true_classified = (preds == y_test).sum()
test_accuracy = true_classified / len(y_test)
print(f"Test accuracy: {test_accuracy}")
>>> Test accuracy: 0.7361111111111112
.
meta_classifier = BaggingClassifier(base_estimator=base_model, n_estimators=10)
meta_classifier.fit(x_train_scaled.reshape(-1, 64), y_train)
>>> BaggingClassifier(
base_estimator=PytorchModel(accuracy_tol=0.02, batch_size=32,
cuda=True, input_shape=(1, 8, 8),
loss_fn=CrossEntropyLoss(),
net_params={},
net_type=<class '__main__.SimpleCNN'>,
optim_params={'lr': 0.001},
optim_type=<class 'torch.optim.adam.Adam'>,
tol_epochs=10),
bootstrap=True, bootstrap_features=False, max_features=1.0,
max_samples=1.0, n_estimators=10, n_jobs=None,
oob_score=False, random_state=None, verbose=0,
warm_start=False)
score
accuracy :
print(meta_classifier.score(x_test_scaled.reshape(-1, 64), y_test))
>>> 0.95
, . . . , , ( "" - ).
?
-, . , Loss' - .
-, Boosting. Bagging' , , . ( ) . sklearn, Loss - .
, . , "" . , .
, . — - , , , , , .
. , Data science' . Computer vision, . ( — !) — FARADAY Lab. — , .
c: