Training neural networks in pattern recognition is a long and resource-intensive process. Especially when at hand there is only an inexpensive laptop, and not a computer with a powerful graphics card. In this case, Google Colaboratory will come to the rescue , which offers to use the Tesla K80 level GPU absolutely free ( more ).
This article describes the process of preparing data, training tensorflow model in Google Colaboratory and its launch on an android device.
Data preparation
As an example, letβs try to train a neural network to recognize white dice on a black background. Accordingly, for starters, you need to create a data set sufficient for training (for now, let's stop at ~ 100 photos).

For training, we will use the Tensorflow Object Detection API . We will prepare all the data necessary for training on a laptop. We need a conda environment and dependency management manager . Installation instructions here .
Let's create an environment for work:
conda create -n object_detection_prepare pip python=3.6
And activate it:
conda activate object_detection_prepare
Set the dependencies that we need:
pip install --ignore-installed --upgrade tensorflow==1.14
pip install --ignore-installed pandas
pip install --ignore-installed Pillow
pip install lxml
conda install pyqt=5
object_detection, object_detection/images.
Google Colab , , "tcmalloc: large alloc....".
object_detection/preprocessing .
:
python ./object_detection/preprocessing/image_resize.py -i ./object_detection/images --imageWidth=800 --imageHeight=600
, 800x600 object_detection/images/resized. object_detection/images.
labelImg.
labelImg object_detection
labelImg
cd [FULL_PATH]/object_detection/labelImg
:
pyrcc5 -o libs/resources.py resources.qrc
( ):
python labelImg.py

βOpen dirβ object_detection/images , . (1, 2, 3, 4, 5, 6). ( *.xml) .
object_detection/training_demo, Google Colab .
( ) 80/20 object_detection/training_demo/images/train object_detection/training_demo/images/test.
object_detection/training_demo/annotations, . label_map.pbtxt, . :
label_map.pbtxtitem {
id: 1
name: '1'
}
item {
id: 2
name: '2'
}
item {
id: 3
name: '3'
}
item {
id: 4
name: '4'
}
item {
id: 5
name: '5'
}
item {
id: 6
name: '6'
}
, ? , TFRecord. [1].
: xml -> csv csv -> record
preprocessing :
cd [FULL_PATH]\object_detection\preprocessing
1. xml csv
:
python xml_to_csv.py -i [FULL_PATH]/object_detection/training_demo/images/train -o [FULL_PATH]/object_detection/training_demo/annotations/train_labels.csv
:
python xml_to_csv.py -i [FULL_PATH]/object_detection/training_demo/images/test -o [FULL_PATH]/object_detection/training_demo/annotations/test_labels.csv
2. csv record
:
python generate_tfrecord.py --label_map_path=[FULL_PATH]\object_detection\training_demo\annotations\label_map.pbtxt --csv_input=[FULL_PATH]\object_detection\training_demo\annotations\train_labels.csv --output_path=[FULL_PATH]\object_detection\training_demo\annotations\train.record --img_path=[FULL_PATH]\object_detection\training_demo\images\train
:
python generate_tfrecord.py --label_map_path=[FULL_PATH]\object_detection\training_demo\annotations\label_map.pbtxt --csv_input=[FULL_PATH]\object_detection\training_demo\annotations\test_labels.csv --output_path=[FULL_PATH]\object_detection\training_demo\annotations\test.record --img_path=[FULL_PATH]\object_detection\training_demo\images\test
, , .
.
ssdlite_mobilenet_v2_coco, android .
object_detection/training_demo/pre-trained-model.
-
object_detection/training_demo/pre-trained-model/ssdlite_mobilenet_v2_coco_2018_05_09
pipeline.config object_detection/training_demo/training ssdlite_mobilenet_v2_coco.config.
, :
1.
model.ssd.num_classes: 6
2. ( ), ,
train_config.batch_size: 18
train_config.num_steps: 20000
train_config.fine_tune_checkpoint:"./training_demo/pre-trained-model/ssdlite_mobilenet_v2_coco_2018_05_09/model.ckpt"
3. (object_detection/training_demo/images/train)
eval_config.num_examples: 64
4.
train_input_reader.label_map_path: "./training_demo/annotations/label_map.pbtxt"
train_input_reader.tf_record_input_reader.input_path:"./training_demo/annotations/train.record"
5.
eval_input_reader.label_map_path: "./training_demo/annotations/label_map.pbtxt"
eval_input_reader.tf_record_input_reader.input_path:"./training_demo/annotations/test.record"
, .
training_demo training_demo.zip Google Drive.
google drive Google Colab,
, .
Google Drive training_demo.zip, Get shareable link id :
drive.google.com/open?id=[YOUR_FILE_ID_HERE]
Google Colab β Google Drive.

CPU. GPU, runtime.


.
:
1. TensorFlow Models:
!git clone https://github.com/tensorflow/models.git
2. protobuf object_detection:
!apt-get -qq install libprotobuf-java protobuf-compiler
%cd ./models/research/
!protoc object_detection/protos/*.proto --python_out=.
%cd ../..
3. PYTHONPATH:
import os
os.environ['PYTHONPATH'] += ":/content/models/research/"
os.environ['PYTHONPATH'] += ":/content/models/research/slim"
os.environ['PYTHONPATH'] += ":/content/models/research/object_detection"
os.environ['PYTHONPATH'] += ":/content/models/research/object_detection/utils"
4. Google Drive PyDrive :
!pip install -U -q PyDrive
from pydrive.auth import GoogleAuth
from pydrive.drive import GoogleDrive
from google.colab import auth
from oauth2client.client import GoogleCredentials
auth.authenticate_user()
gauth = GoogleAuth()
gauth.credentials = GoogleCredentials.get_application_default()
drive = GoogleDrive(gauth)
5. ( id ) :
drive_file_id="[YOUR_FILE_ID_HERE]"
training_demo_zip = drive.CreateFile({'id': drive_file_id})
training_demo_zip.GetContentFile('training_demo.zip')
!unzip training_demo.zip
!rm training_demo.zip
6. :
!python ./models/research/object_detection/legacy/train.py --logtostderr --train_dir=./training_demo/training --pipeline_config_path=./training_demo/training/ssdlite_mobilenet_v2_coco.config
--train_dir=./training_demo/training β ,
--pipeline_config_path=./training_demo/training/ssdlite_mobilenet_v2_coco.config β
7. frozen graph, :
!python /content/models/research/object_detection/export_inference_graph.py --input_type image_tensor --pipeline_config_path /content/training_demo/training/ssdlite_mobilenet_v2_coco.config --trained_checkpoint_prefix /content/training_demo/training/model.ckpt-[CHECKPOINT_NUMBER]
--output_directory /content/training_demo/training/output_inference_graph_v1.pb
--pipeline_config_path /content/training_demo/training/ssdlite_mobilenet_v2_coco.config β
--trained_checkpoint_prefix /content/training_demo/training/model.ckpt-[CHECKPOINT_NUMBER] β , .
--output_directory /content/training_demo/training/output_inference_graph_v1.pb β
[CHECKPOINT_NUMBER], content/training_demo/training/. model.ckpt-1440.index, model.ckpt-1440.meta. 1440 β [CHECKPOINT_NUMBER] .

. ~20000 .

8. tflite.
tensorflow lite tflite. frozen graph tflite ( export_inference_graph.py):
!python /content/models/research/object_detection/export_tflite_ssd_graph.py --pipeline_config_path /content/training_demo/training/ssdlite_mobilenet_v2_coco.config --trained_checkpoint_prefix /content/training_demo/training/model.ckpt-[CHECKPOINT_NUMBER] --output_directory /content/training_demo/training/output_inference_graph_tf_lite.pb
tflite , output_inference_graph_tf_lite.pb:

Netron. .
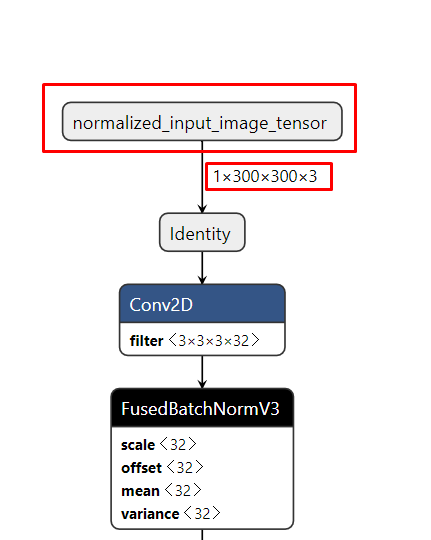

pb tflite :
!tflite_convert --output_file=/content/training_demo/training/model_q.tflite --graph_def_file=/content/training_demo/training/output_inference_graph_tf_lite_v1.pb/tflite_graph.pb --input_arrays=normalized_input_image_tensor --output_arrays='TFLite_Detection_PostProcess','TFLite_Detection_PostProcess:1','TFLite_Detection_PostProcess:2','TFLite_Detection_PostProcess:3' --input_shapes=1,300,300,3 --enable_select_tf_ops --allow_custom_ops --inference_input_type=QUANTIZED_UINT8 --inference_type=FLOAT --mean_values=128 --std_dev_values=128
--output_file=/content/training_demo/training/model_q.tflite β
--graph_def_file=/content/training_demo/training/output_inference_graph_tf_lite_v1.pb/tflite_graph.pb β frozen graph,
--input_arrays=normalized_input_image_tensor β ,
--output_arrays='TFLite_Detection_PostProcess','TFLite_Detection_PostProcess:1','TFLite_Detection_PostProcess:2','TFLite_Detection_PostProcess:3' β ,
--input_shapes=1,300,300,3 β ,
--enable_select_tf_ops β runtime TensorFlow Lite
--allow_custom_ops β TensorFlow Lite Optimizing Converter
--inference_type=FLOAT β
--inference_input_type=QUANTIZED_UINT8 β
--mean_values=128 --std_dev_values=128 β , QUANTIZED_UINT8
Google Drive:
!zip -r ./training_demo/training.zip ./training_demo/training/
training_result = drive.CreateFile({'title': 'training_result.zip'})
training_result.SetContentFile('training_demo/training.zip')
training_result.Upload()
Invalid client secrets file, google drive.
android
android object detection, kotlin CameraX. .
CameraX ImageAnalysis. ObjectDetectorAnalyzer.
:
1. YUV . RGB :
val rgbArray = convertYuvToRgb(image)
2. (, , , 300x300), Bitmap :
val rgbBitmap = getRgbBitmap(rgbArray, image.width, image.height)
val transformation = getTransformation(rotationDegrees, image.width, image.height)
Canvas(resizedBitmap).drawBitmap(rgbBitmap, transformation, null)
3. bitmap , :
ImageUtil.storePixels(resizedBitmap, inputArray)
val objects = detect(inputArray)
4. RecognitionResultOverlayView :
val scaleFactorX = measuredWidth / result.imageWidth.toFloat()
val scaleFactorY = measuredHeight / result.imageHeight.toFloat()
result.objects.forEach { obj ->
val left = obj.location.left * scaleFactorX
val top = obj.location.top * scaleFactorY
val right = obj.location.right * scaleFactorX
val bottom = obj.location.bottom * scaleFactorY
canvas.drawRect(left, top, right, bottom, boxPaint)
canvas.drawText(obj.text, left, top - 25f, textPaint)
}
, assets training_demo/training/model_q.tflite ( detect.tflite) labelmap.txt, :
SSD Mobilenet V1, 1, 0, labelOffset 1 0 collectDetectionResult ObjectDetector.
.
, Xiaomi Redmi 4X :
: