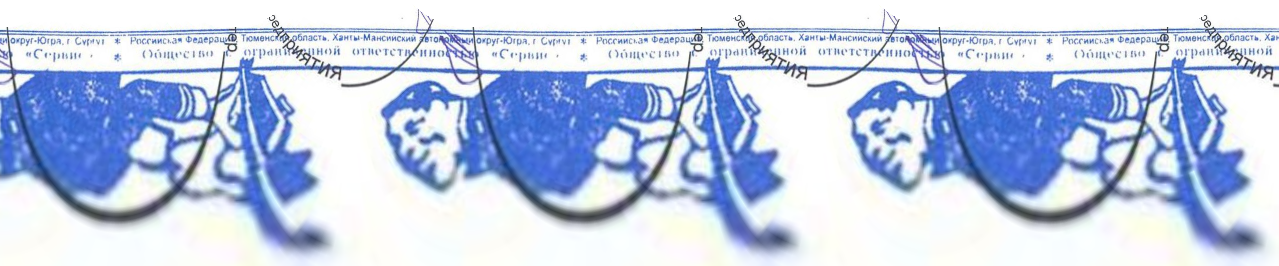
Not so long ago, we were faced with the task of finding and extracting stamps from documents. What for? For example, to verify the presence of seals in contracts from two parties (parties to the contract). We in the bins already had a prototype for finding them, written in OpenCV, but it was damp. We decided to dig out this relic, shake off the dust from it and make a working solution on its basis.
Most of the techniques described here can be applied outside the search for stamps. For instance:
- color segmentation;
- search for round objects / circles;
- image conversion to polar coordinate system;
- intersection of objects, Intersection over Union (IoU, Jacquard coefficient).
As a result, we had two options: to solve using neural networks or to resurrect a prototype on OpenCV. Why did we decide to take OpenCV? The answer is at the end of the article.
Code examples will be presented in Python and C #. For Python, you need the opencv-python and numpy packages , for C # you need OpenCvSharp and opencv .
Hope you are familiar with the basic OpenCV algorithms. I wonβt dive deep into them, since most of them deserve a separate, detailed article, but Iβll leave links with the mat. part of all thirsty.
, ( HLS-), . - , , .
. β .
, ? .
. / , , -, , .
, , python . ( , , ). ( Intersection over Union), .
:
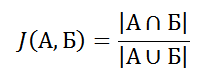
:
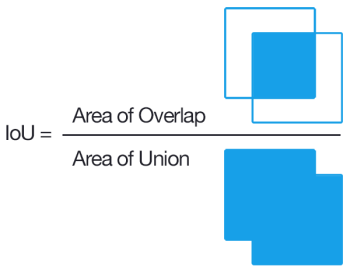
, , , , - . , , , :
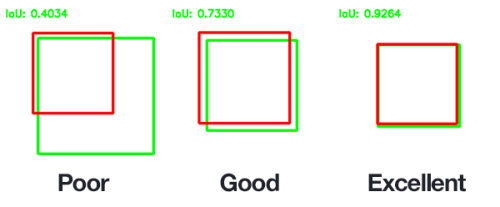
( 0.6) , F1- ( β ).
IoUimport sys
from collections import namedtuple
Metrics = namedtuple("metrics", ["tp", "fp", "fn"])
def bb_intersection_over_union(a, b):
x_a = max(a[0], b[0])
y_a = max(a[1], b[1])
x_b = min(a[2], b[2])
y_b = min(a[3], b[3])
inter_area = max(0, x_b - x_a + 1) * max(0, y_b - y_a + 1)
a_area = (a[2] - a[0] + 1) * (a[3] - a[1] + 1)
b_area = (b[2] - b[0] + 1) * (b[3] - b[1] + 1)
iou = inter_area / float(a_area + b_area - inter_area)
return iou
def same_stamp(a, b):
""", """
iou = bb_intersection_over_union(a, b)
print(f'iou: {iou}')
return iou > 0.6
def compare_stamps(extracted, mapped):
"""
:param extracted: , .
:param mapped: () .
"""
tp = []
fp = []
fn = list(mapped)
for stamp in extracted:
for check in fn:
if same_stamp(stamp, check):
tp.append(check)
fn.remove(check)
break
else:
fp.append(stamp)
return Metrics(len(tp), len(fp), len(fn))
def compare(file, sectors):
"""
.
:param file: .
:param sectors: .
"""
print(f'file: {file}')
try:
stamps = extract_stamps(file)
metrics = compare_stamps(stamps, [ss for ss in sectors if 'stamp' in ss['tags']])
return file, metrics
except:
print(sys.exc_info())
return file, Metrics(0, 0, 0)
if __name__ == '__main__':
file_metrics = {}
for file, sectors in dataset.items():
file_metrics[file] = compare(file, sectors)
total_metrics = Metrics(*(sum(x) for x in zip(*file_metrics.values())))
precision = total_metrics.tp / (total_metrics.tp + total_metrics.fp) if total_metrics.tp > 0 else 0
recall = total_metrics.tp / (total_metrics.tp + total_metrics.fn) if total_metrics.tp > 0 else 0
f1 = 2 * precision * recall / (precision + recall) if (precision + recall) > 0 else 0
print('precision\trecall\tf1')
print('{:.4f}\t{:.4f}\t{:.4f}'.format(precision * 100, recall * 100, f1 * 100).replace('.', ','))
print('tp\tfp\tfn')
print('{}\t{}\t{}'.format(total_metrics.tp, total_metrics.fp, total_metrics.fn))
print('tp\tfp\tfn')
for file in dataset.keys():
metric = file_metrics.get(file)
print(f'{metric.tp}\t{metric.fp}\t{metric.fn}')
print(f'precision: {precision}, recall: {recall}, f1: {f1}, {total_metrics}')
HLS-
() - . , . ( ):
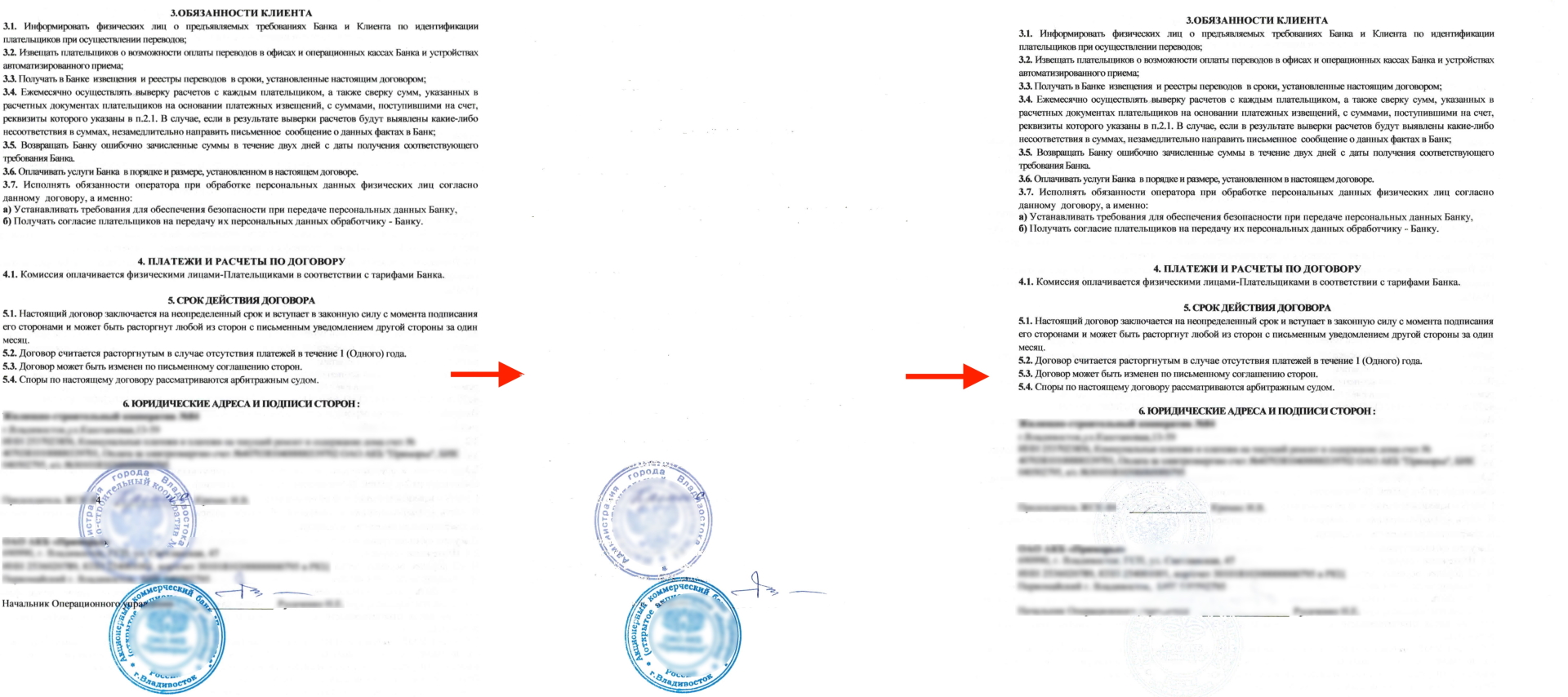
.
HLS . Hue, Lightness, Saturation, , , . :
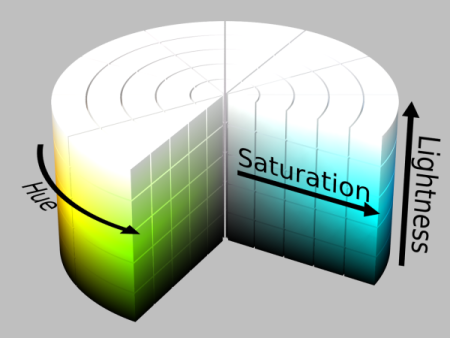
? - . , ~180 ~280.
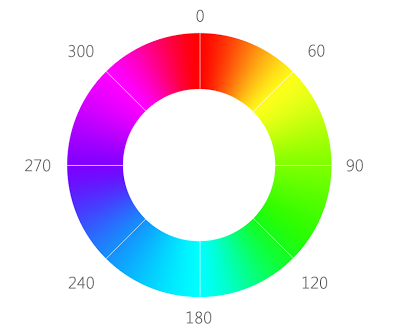
, - , , .
(Python)def colored_mask(img, threshold = -1):
denoised = cv2.medianBlur(img, 3)
cv2.imwrite('denoised.bmp', denoised)
gray = cv2.cvtColor(denoised, cv2.COLOR_BGR2GRAY)
cv2.imwrite('gray.bmp', gray)
adaptiveThreshold = threshold if threshold >= 0 else cv2.mean(img)[0]
color = cv2.cvtColor(denoised, cv2.COLOR_BGR2HLS)
mask = cv2.inRange(color, (0, int(adaptiveThreshold / 6), 60), (180, adaptiveThreshold, 255))
dst = cv2.bitwise_and(gray, gray, mask=mask)
cv2.imwrite('colors_mask.bmp', dst)
return dst
(C#)private void ColoredMask(Mat src, Mat dst, double threshold = -1)
{
using (var gray = new Mat())
using (var color = new Mat())
using (var mask = new Mat())
using (var denoised = new Mat())
{
Cv2.MedianBlur(src, denoised, 3);
denoised.Save("colors_denoised.bmp");
Cv2.CvtColor(denoised, gray, ColorConversionCodes.BGR2GRAY);
gray.Save("colors_gray.bmp");
var adaptiveThreshold = threshold < 0 ? src.Mean()[0] : threshold;
Cv2.CvtColor(denoised, color, ColorConversionCodes.BGR2HLS);
Cv2.InRange(color, new Scalar(0, adaptiveThreshold / 6, 60), new Scalar(180, adaptiveThreshold, 255), mask);
Cv2.BitwiseAnd(gray, gray, dst, mask);
dst.Save("colors_mask.bmp");
}
}
:
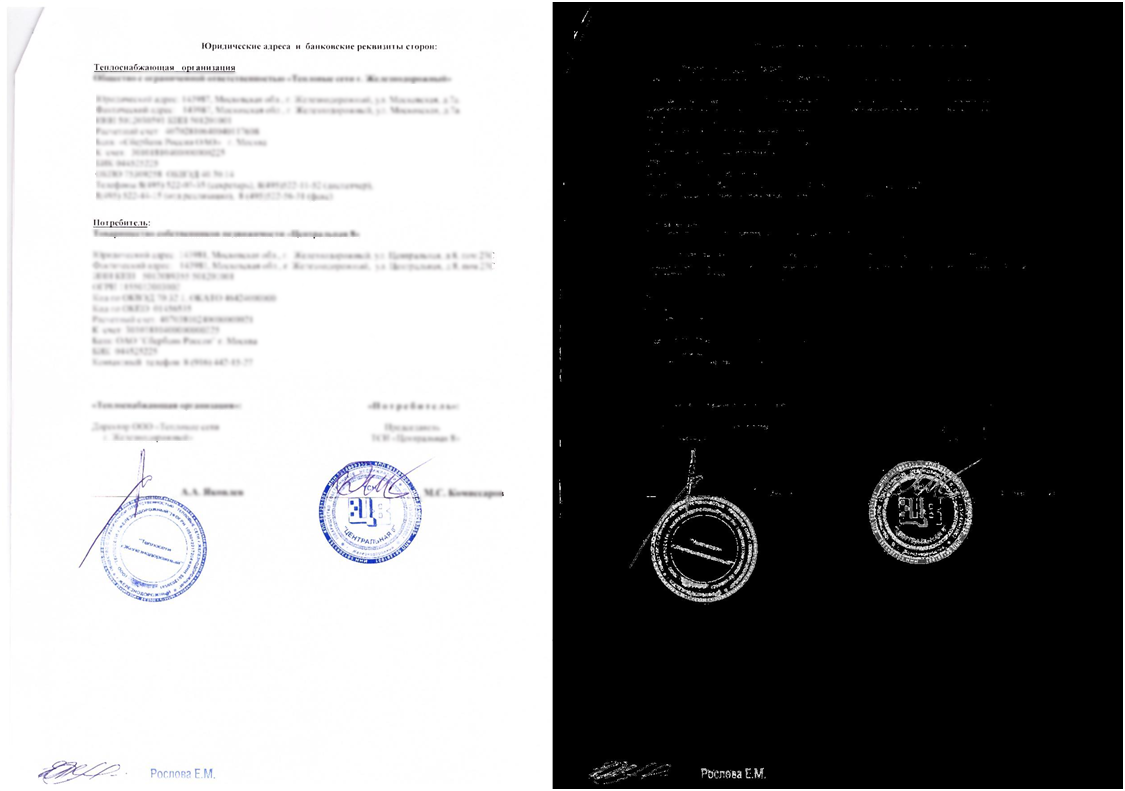
, HLS OpenCV 0 360, 0 180. β uint8/uchar/byte, 0 255, 360 2.
, , ? , , β β.
, . , ( ) , , β . , .
, , . OpenCV , , . , , .
(Python)
circles = cv2.HoughCircles(mask, cv2.HOUGH_GRADIENT, 1, 20, param1, param2, minRadius, maxRadius)
circles = np.uint16(np.around(circles))
cimg = cv2.cvtColor(img,cv2.COLOR_GRAY2BGR)
for i in circles[0,:]:
cv2.circle(cimg,(i[0],i[1]),i[2],(165,25,165),2)
(C#)
var houghCircles = Cv2.HoughCircles(morphed, HoughMethods.Gradient, 1, max, cannyEdgeThreshold, houghThreshold, min, max);
foreach (var circle in houghCircles)
Cv2.Circle(morphed, circle.Center, (int)circle.Radius, new Scalar(165, 25, 165), 2);
:
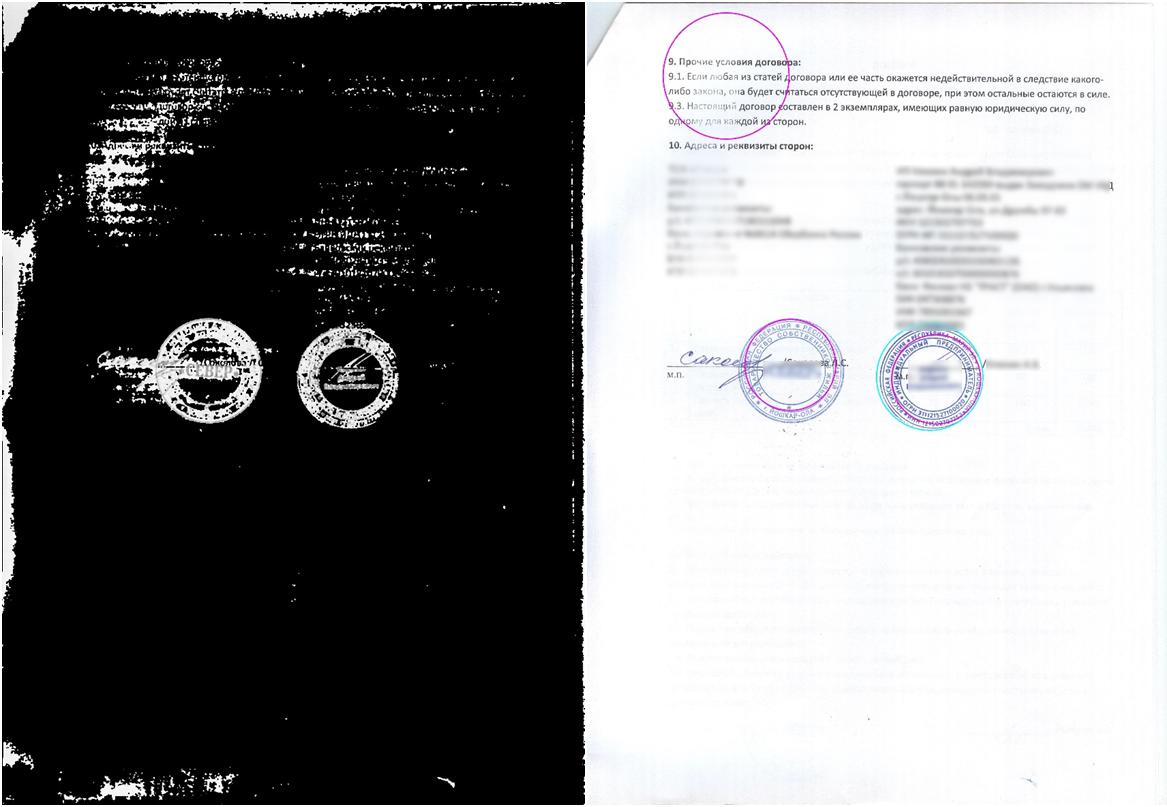
, , . , , . , , , . (- ββ - , - ).
- . .
, , . ββ , , . β , .
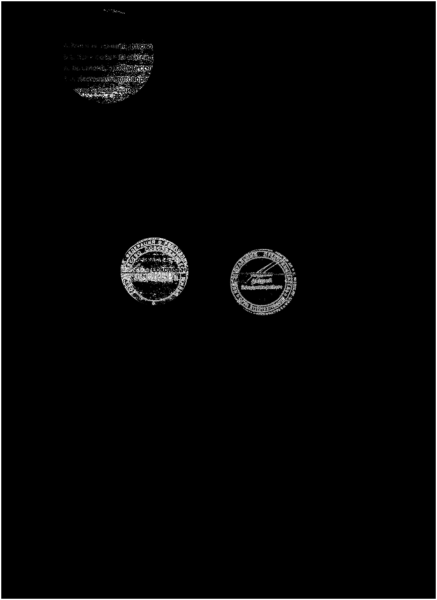
, , , . . , . ββ .
(Python)def equals(first, second, epsilon):
diff = cv2.subtract(first, second)
nonZero = cv2.countNonZero(diff)
area = first.size * epsilon
return nonZero <= area
for i in circles[0, :]:
empty = np.zeros((256, 256, 1), dtype="uint8")
cv2.circle(empty, (i[0], i[1]), i[2], (255, 255, 255), -1)
crop = img * (empty.astype(img.dtype))
cv2.imwrite('crop.bmp', crop)
if not equals(crop, empty, threshold):
result.append(i)
(C#)foreach (var circle in circles)
{
var x = (int) circle.Center.X;
var y = (int) circle.Center.Y;
var radius = (int) Math.Floor(circle.Radius);
using (var empty = Mat.Zeros(src.Rows, src.Cols, MatType.CV_8UC1))
using (var mask = empty.ToMat())
using (var crop = new Mat())
{
Cv2.Circle(mask, x, y, radius, Scalar.White, -1);
src.CopyTo(crop, mask);
crop.Save("crop.bmp");
if (!MatEquals(crop, empty, threshold))
result.Add(circle);
}
}
private bool MatEquals(Mat a, Mat b, double epsilon = 0.0)
{
var notEquals = a.NotEquals(b);
var nonZero = Cv2.CountNonZero(notEquals);
var area = a.Rows * epsilon * a.Cols;
return nonZero <= area;
}
. , , , - ?
, β , β (minor) (major) ( , , ).
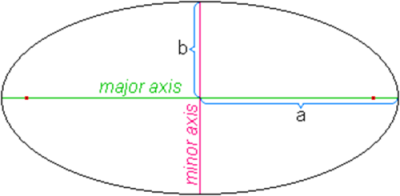
: , , , .
() , , voila β .
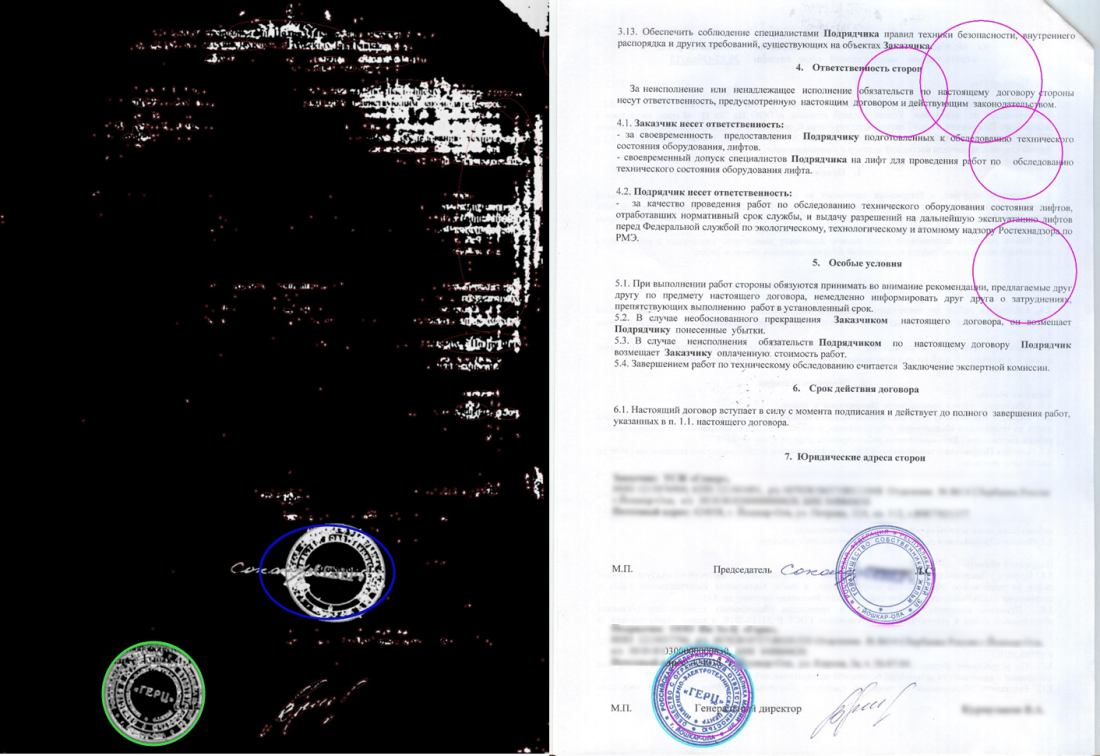
β , β , β , β , .
(Python)segments = []
contours, hierarchy = cv2.findContours(img, cv2.RETR_EXTERNAL, cv2.CHAIN_APPROX_SIMPLE)
for contour in contours:
if contour.shape[0] < 5:
continue
ellipse = cv2.fitEllipse(contour)
width = ellipse[1][0]
height = ellipse[1][1]
minor = min(width, height)
major = max(width, height)
if minor / 2 > minorMin and major / 2 < majorMax:
r1 = math.fabs(1 - math.fabs(major - minor) / max(minor, major))
cv2.ellipse(src, ellipse, (255, 0, 0), 3)
if r1 > roundness:
segments.append((ellipse[0], major / 2))
cv2.ellipse(src, ellipse, (0, 255, 0), 3)
else: cv2.ellipse(src, ellipse, (0, 0, 255), 1)
cv2.imwrite('test_res.bmp', src)
(Python)def distance(p1, p2):
return math.sqrt(math.pow(p2[0] - p1[0], 2) + math.pow(p2[1] - p1[1], 2))
def isNested(inner, outer, epsilon):
distance = distance(inner[0], outer[0])
radius = outer[1] * epsilon
return distance < radius and inner[1] < radius - distance
nested = []
for i in inner:
for o in outer:
if (isNested(i, o, 1.3)):
if (distance(i[0], i[1]) < 30 and i[1] / o[1] > 0.75):
nested.append(i)
else: nested.append(o)
(C#)Cv2.FindContours(src, out var contours, hierarchy, RetrievalModes.External, ContourApproximationModes.ApproxSimple);
foreach (var contour in contours)
{
if (contour.Height < 5)
continue;
var ellipse = Cv2.FitEllipse(contour);
var minor = Math.Min(ellipse.Size.Width, ellipse.Size.Height);
var major = Math.Max(ellipse.Size.Width, ellipse.Size.Height);
if (minor / 2 > minorSize && major / 2 < majorSize)
{
var r1 = Math.Abs(1 - Math.Abs(major - minor) / Math.Max(minor, major));
if (r1 > roundness)
{
var circle = new CircleSegment(ellipse.Center, major / 2);
segments.Add(circle);
}
}
contour.Dispose();
}
}
(C#)private bool IsCirclesNested(CircleSegment inner, CircleSegment outer, double epsilon)
{
var distance = inner.Center.DistanceTo(outer.Center);
var secondRadius = outer.Radius * epsilon;
return distance < secondRadius && inner.Radius < secondRadius - distance;
}
var nested = new List<CircleSegment>();
foreach (var i in inner)
foreach (var o in outer)
{
if (IsCirclesNested(i, o, 1.3))
{
if (i.Center.DistanceTo(o.Center) < 30 &&
i.Radius / o.Radius > 0.75)
nested.Add(i);
else nested.Add(o);
}
}
return outer.Union(inner).Except(nested).ToList();
, , , . . β , .
β β, , - . β β? ββ . ? , - . :
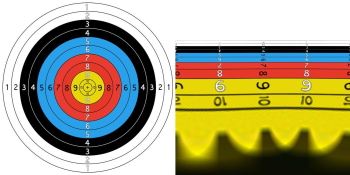
, , : , , . :
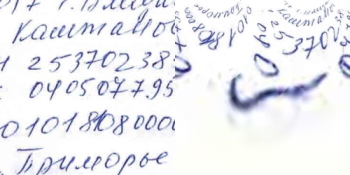
β X Y , X, . , , , .
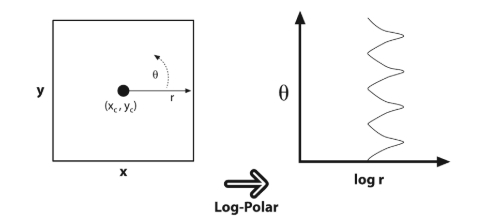
β , , ( ). , , ( , , , ).
, , . ( ) 90 .
(Python)maxRadius = math.sqrt(math.pow(src.shape[0], 2) + math.pow(src.shape[1], 2)) / 2
magnitude = src.shape[0] / math.log(maxRadius)
center = (src.shape[0] / 2, src.shape[1] / 2)
polar = cv2.logPolar(src, center, magnitude, cv2.INTER_AREA)
(C#)var maxRadius = Math.Sqrt(Math.Pow(stampImage.Width, 2) + Math.Pow(stampImage.Height, 2)) / 2;
var magnitude = stampImage.Width / Math.Log(maxRadius);
var center = new Point2f(stampImage.Width / 2, stampImage.Height / 2);
Cv2.LogPolar(stampImage, cartesianImage, center, magnitude, InterpolationFlags.Area);
, , OpenCVβ . , , , , OpenCV. , .
:
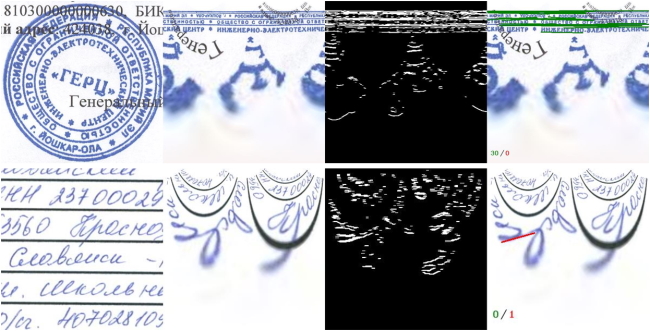
, , 90 , .
(Python)
sobel = cv2.Sobel(polar, cv2.CV_16S, 1, 0)
kernel = cv2.getStructuringElement(shape=cv2.MORPH_RECT, ksize=(1, 5))
img = cv2.convertScaleAbs(sobel)
img = cv2.cvtColor(img, cv2.COLOR_BGR2GRAY)
img = cv2.threshold(img, 0, 255, cv2.THRESH_BINARY + cv2.THRESH_OTSU)[1]
img = cv2.morphologyEx(img, cv2.MORPH_OPEN, kernel)
img = cv2.morphologyEx(img, cv2.MORPH_CLOSE, kernel)
def is_vertical(img_src, line):
tolerance = 10
coords = line[0]
angle = math.atan2(coords[3] - coords[1], coords[2] - coords[0]) * 180.0 / math.pi
edge = img_src.shape[0] * 0.66
out_of_bounds = coords[0] < edge and coords[2] < edge
return math.fabs(90 - math.fabs(angle)) <= tolerance and not out_of_bounds
lines = cv2.HoughLinesP(img, 1, math.pi / 180, 15, img.shape[0] / 5, 10)
vertical = [line for line in lines if is_vertical(img, line)]
correct_lines = len(vertical)
(C#)
using (var sobel = new Mat())
using (var kernel = Cv2.GetStructuringElement(MorphShapes.Rect, new Size(1, 5)))
{
Cv2.Sobel(img, sobel, MatType.CV_16S, 1, 0);
Cv2.ConvertScaleAbs(sobel, img);
Cv2.Threshold(img, img, 0, 255, ThresholdTypes.Binary | ThresholdTypes.Otsu);
Cv2.MorphologyEx(img, img, MorphTypes.Open, kernel);
Cv2.MorphologyEx(img, img, MorphTypes.Close, kernel);
}
bool AlmostVerticalLine(LineSegmentPoint line)
{
const int tolerance = 10;
var angle = Math.Atan2(line.P2.Y - line.P1.Y, line.P2.X - line.P1.X) * 180.0 / Math.PI;
var edge = edges.Width * 0.66;
var outOfBounds = line.P1.X < edge && line.P2.X < edge;
return Math.Abs(90 - Math.Abs(angle)) <= tolerance && !outOfBounds;
}
var lines = Cv2.HoughLinesP(img, 1, Math.PI / 180, 15, img.Width / 5, 10);
var correctLinesCount = lines.Count(AlmostVerticalLine);
, 85%. :
, β β ?β. :
, , , . OpenCV , . U-Net, OpenCV , .