In diesem Artikel werde ich über sechs Tools sprechen, die Ihren Pandas-Code erheblich beschleunigen können. Ich habe die Tools nach einem Prinzip zusammengestellt - einfache Integration in die vorhandene Codebasis. Installieren Sie für die meisten Tools einfach das Modul und fügen Sie ein paar Codezeilen hinzu.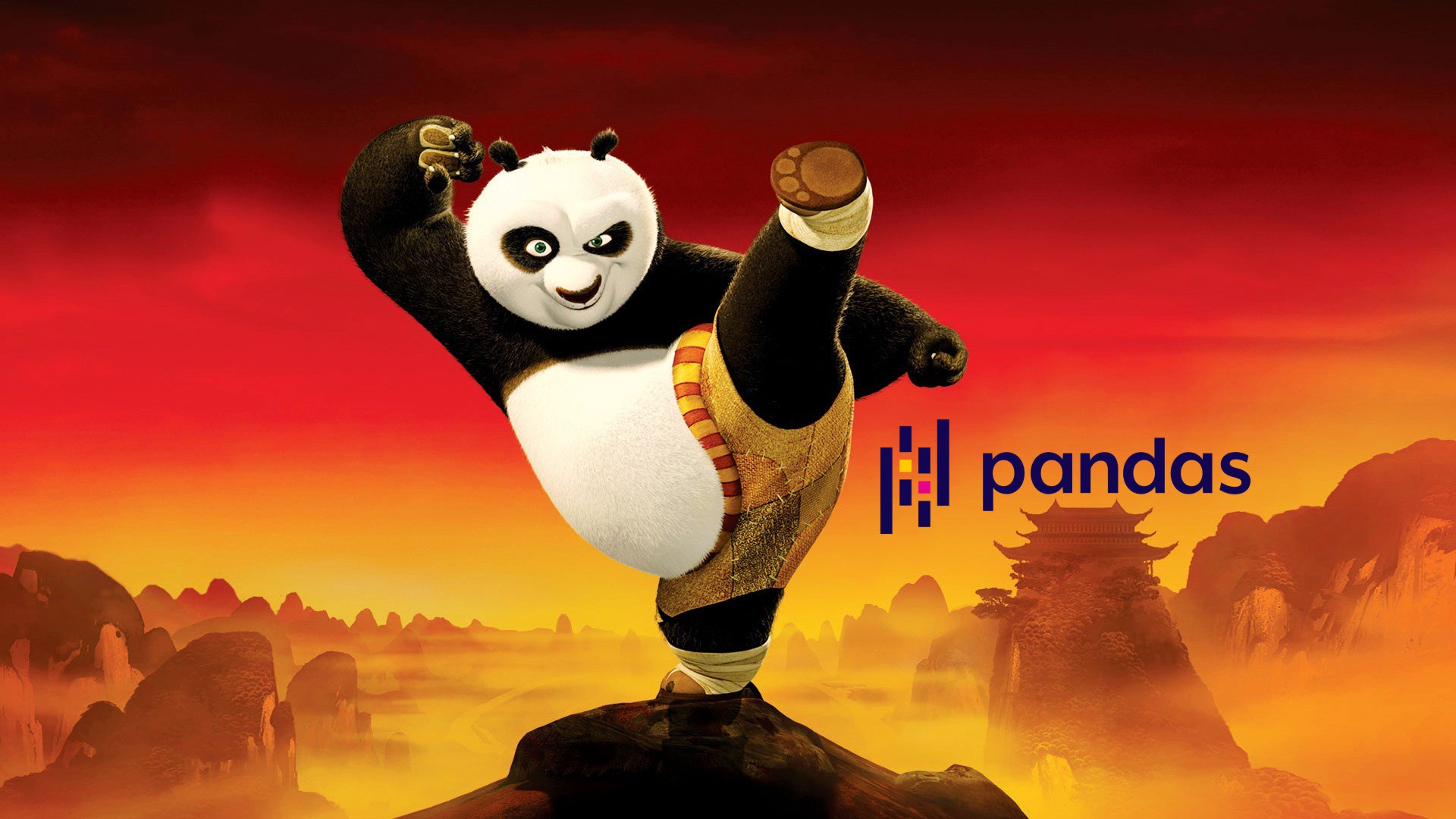
Pandas API, , . , , , .
, Spark DataFlow. :
1:
- Numba
- Multiprocessing
- Pandarallel
Numba
Python. Numba — JIT , , Numpy, Pandas. , .
— , - apply
.
import numpy as np
import numba
df = pd.DataFrame(np.random.randint(0,100,size=(100000, 4)),columns=['a', 'b', 'c', 'd'])
def multiply(x):
return x * 5
@numba.vectorize
def multiply_numba(x):
return x * 5
, . . .
In [1]: %timeit df['new_col'] = df['a'].apply(multiply)
23.9 ms ± 1.93 ms per loop (mean ± std. dev. of 7 runs, 10 loops each)
In [2]: %timeit df['new_col'] = df['a'] * 5
545 µs ± 21.4 µs per loop (mean ± std. dev. of 7 runs, 1000 loops each)
In [3]: %timeit df['new_col'] = multiply_numba(df['a'].to_numpy())
329 µs ± 2.37 µs per loop (mean ± std. dev. of 7 runs, 1000 loops each)
~70 ! , , Pandas , . :
def square_mean(row):
row = np.power(row, 2)
return np.mean(row)
@numba.njit
def square_mean_numba(arr):
res = np.empty(arr.shape[0])
arr = np.power(arr, 2)
for i in range(arr.shape[0]):
res[i] = np.mean(arr[i])
return res
:
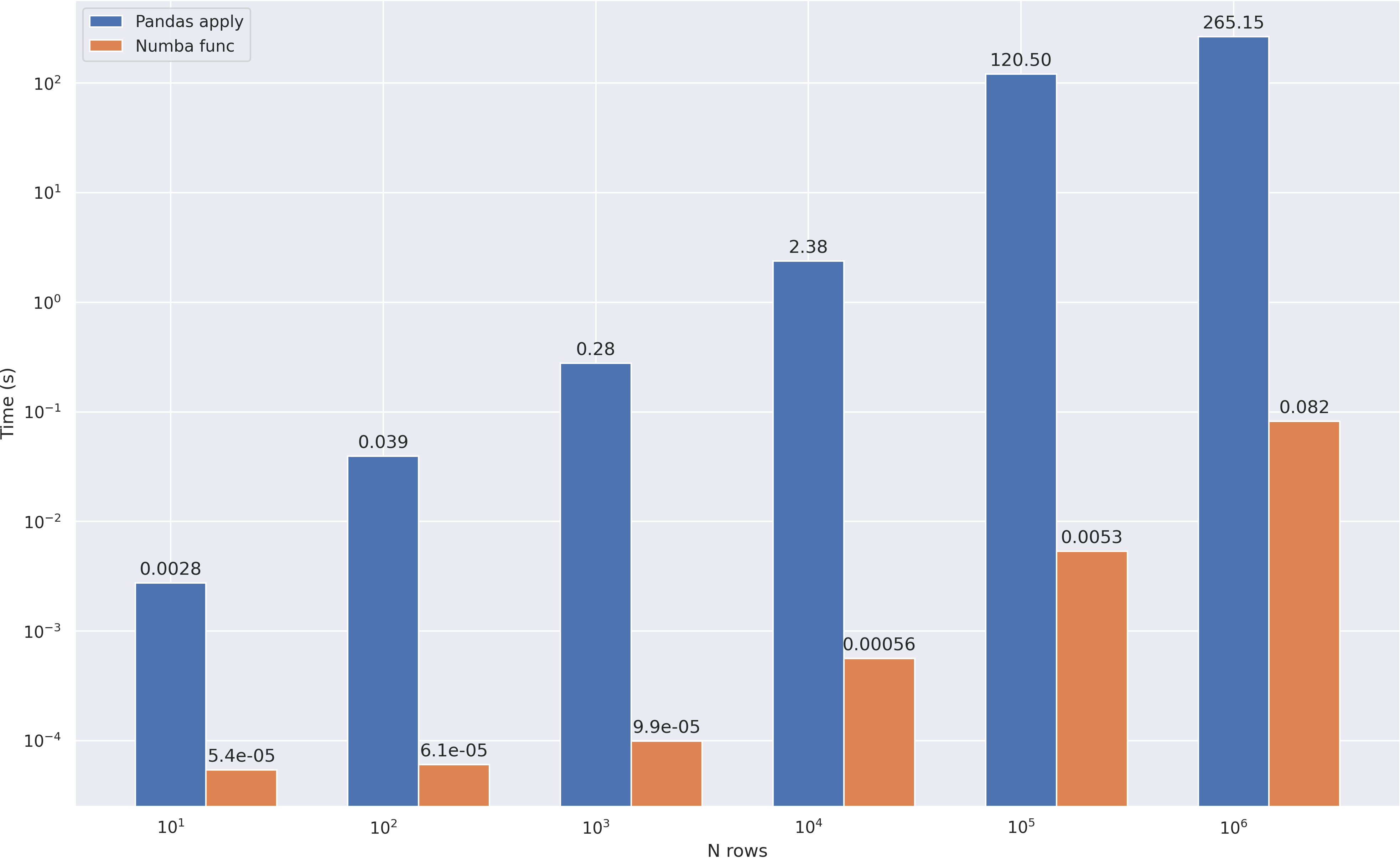
Multiprocessing
, , , . - , python.
. . , apply
:
df = pd.read_csv('abcnews-date-text.csv', header=0)
df = pd.concat([df] * 10)
df.head()
def mean_word_len(line):
for i in range(6):
words = [len(i) for i in line.split()]
res = sum(words) / len(words)
return res
def compute_avg_word(df):
return df['headline_text'].apply(mean_word_len)
:
from multiprocessing import Pool
n_cores = 4
pool = Pool(n_cores)
def apply_parallel(df, func):
df_split = np.array_split(df, n_cores)
df = pd.concat(pool.map(func, df_split))
return df
:

Pandarallel
Pandarallel — pandas, . , , + progress bar ;) , pandarallel.

. , . pandarallel — :
from pandarallel import pandarallel
pandarallel.initialize()
, — apply
parallel_aply
:
df['headline_text'].parallel_apply(mean_word_len)
:

- overhead 0.5 .
parallel_apply
, . 1 , , . - , , , 2-3 .
- Pandarallel
parallel_apply
(groupby
), .
, , . / , API progress bar.
To be continued
In diesem Teil haben wir uns zwei ziemlich einfache Ansätze zur Pandas-Optimierung angesehen - mithilfe der JIT-Kompilierung und der Aufgabenparallelisierung. Im nächsten Teil werde ich über interessantere und komplexere Tools sprechen, aber ich schlage vor, dass Sie die Tools selbst testen, um sicherzustellen, dass sie effektiv sind.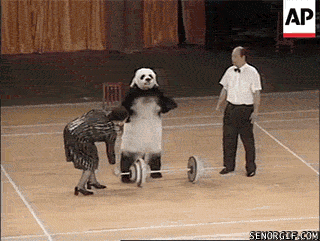
PS: Vertrauen, aber überprüfen - den gesamten im Artikel verwendeten Code (Benchmarks und Grafiken) habe ich auf github gepostet