Hallo Habr! Plötzlich wurde uns klar, dass unser letzter Artikel über Elbrus vor einem Jahr herauskam. Deshalb haben wir uns entschlossen, dieses nervige Versehen zu korrigieren, weil wir dieses Thema nicht aufgegeben haben!
Eine Erkennung ohne neuronale Netze ist schwer vorstellbar. Wir werden daher darüber sprechen, wie wir 8-Bit-Grids auf Elbrus gestartet haben und was daraus entstanden ist. Im Allgemeinen ist ein Modell mit 8-Bit-Koeffizienten und -Eingaben und 32-Bit-Zwischenberechnungen äußerst beliebt. Zum Beispiel haben Google [1] und Facebook [2] eigene Implementierungen gestartet, die den Zugriff auf den Speicher optimieren, SIMD verwenden und es Ihnen ermöglichen, Berechnungen um 25% oder mehr zu beschleunigen, ohne dass die Genauigkeit merklich abnimmt (dies hängt natürlich von der Architektur des neuronalen Netzwerks und des Rechners ab, aber Sie benötigen es wurde erklärt wie cool es ist?).

Die Idee, reelle Zahlen durch ganze Zahlen zu ersetzen, um die Berechnungen zu beschleunigen, liegt in der Luft:
- . , “” , float , ;
- . - , , … , . , (SIMD). c 128- SIMD 4 float’ 16 uint8. 4 , ;
- , . — !
8- [3] . , , .
8- , 16-, . 32- . , 8- . , .
, — . (. . 1) :
— , — , — , — .
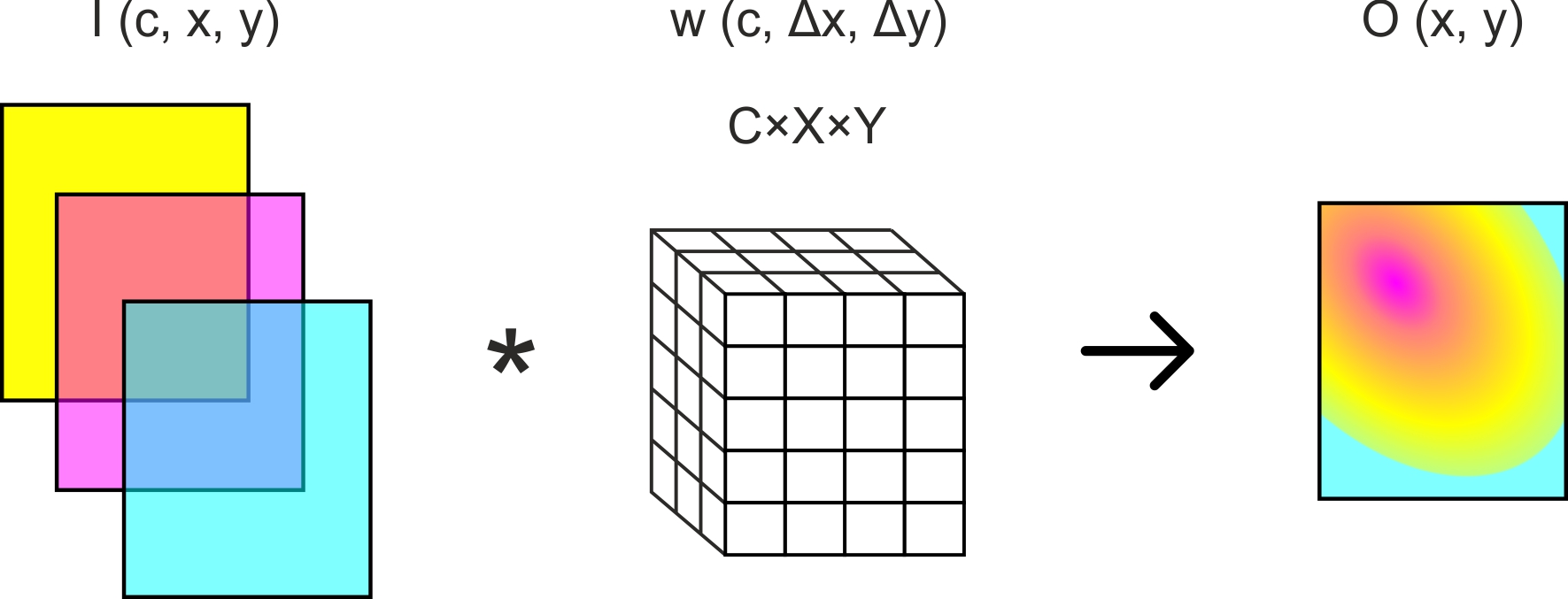
. 1. C x X x Y.
— [4]. , , . , "" — .. , . , .. (1). "" , .. ( ), ..
8- 32- .
- , , .
– , . , , — . . , , , [5-7].
6 ( ), . : - , . , , , , , . , .
. 1 (). 32 .
1. . "/" , , .
, - APB (array prefetch buffer). APB n- , . -, , APB , . APB :
, APB :
, , . , . Goto [8] . , , , . .
, , (. . 2-3). — . , .. . , APB - : , 8 ( 64- ) 2 .
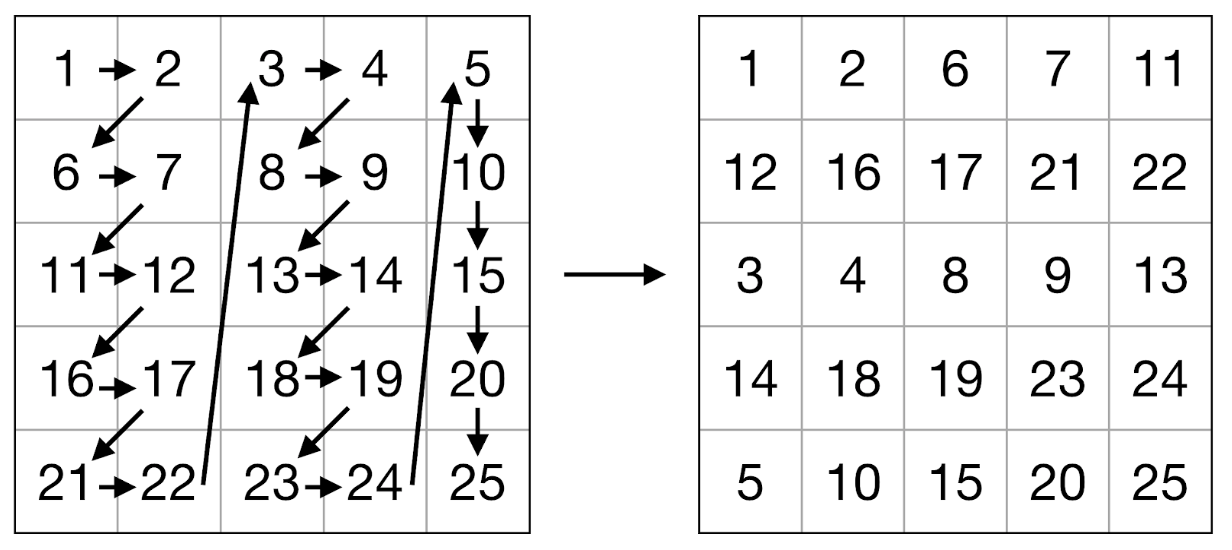
. 2. .

3. .
:
for bl_r in block_r(rhs):
packed_r <- pack_rhs(bl_r)
for bl_l in block_l(lhs):
packed_l <- pack_lhs(bl_l)
packed_res <- pack_res(bl_res)
kernel(packed_res, packed_l, packed_r)
bl_res <- unpack_res(packed_res)
lhs
rhs
— , block_l(.)
, block_r(.)
— , lhs
rhs
. pack_rhs
pack_lhs
, pack_res
— , unpack_res
— . kernel
.
kernel
:
for j in {0, ..., cols / nr}
{dst0, dst1} <-
for i in {0, ..., rows / mr}
for k in {0, ..., depth / 2}
bl_r <-
bl_l <-
lhs <- pshufb(zero, bl_l, 0x0901080009010800LL)
rhs0 <- punpcklbh(zero, bl_r)
rhs1 <- punpckhbh(zero, bl_r)
dst0 <- dst0 + pmaddh(rhs0, lhs)
dst1 <- dst1 + pmaddh(rhs1, lhs);
//
pshufb
— , ( ), punpckhbh
— , 16- , punpcklbh
— , 16- , pmaddh
— , 16- .
. nr mr = 12 8.
, 8 48 . 8 3, 14 , — 48 +, 8 .
2 8- -4, EML. N = 10^6
.
2. EML .
, -4 . , .
8- , x86 ARM, . , , , . 8- . , , ( , x86 ARM), : 6 64- , (64 128 ) 2 6 .
, 8- 32- . 3 (-4), 4 5 (-8 -8).
? , . , “” , . - , . , , / .
- 8- ? , , , - ( ).
P.S.
Limonova E. E., Neyman-Zade M. I., Arlazarov V. L. Special aspects of matrix operation implementations for low-precision neural network model on the Elbrus platform // . . — 2020. — . 13. — № 1. — . 118-128. — DOI: 10.14529/mmp200109.
- https://github.com/google/gemmlowp
- https://engineering.fb.com/ml-applications/qnnpack/
- Vanhoucke, Vincent, Andrew Senior, and Mark Z. Mao. "Improving the speed of neural networks on CPUs." (2011).
- K. Chellapilla, S. Puri, P. Simard. High Performance Convolutional Neural Networks for Document Processing // Tenth International Workshop on Frontiers in Handwriting Recognition. – Universite de Rennes, 1 Oct 2006. – La Baule (France). – 2006.
- .., . ., . . “”. – .: , – 2013. – 272 c.
- , .. / .. , .. , .. // - . – .: - “” . . .. . – 2015. – No4 (8). – cc. 64-68.
- Limonova E. E., Skoryukina N. S., Neyman-Zade M. I. Fast Hamming Distance Computation for 2D Art Recognition on VLIW-Architecture in Case of Elbrus Platform // ICMV 2018 / SPIE. 2019. . 11041. ISSN 0277-786X. ISBN 978-15-10627-48-2. 2019. . 11041. 110411N. DOI: 10.1117/12.2523101
- Goto, K. Anatomy of high-performance matrix multiplication / K. Goto, R.A. Geijn // ACM Transactions on Mathematical Software (TOMS) – 2008. – 34(3). – p.12.