Neuronale Netze sind sehr beliebt. Ihr Hauptvorteil besteht darin, dass sie ziemlich komplexe Daten verallgemeinern können, bei denen andere Algorithmen eine geringe Qualität aufweisen. Was aber, wenn die Qualität des neuronalen Netzes immer noch unbefriedigend ist?
Und hier kommen Ensembles zur Rettung ...
Was sind Ensembles?
Ein Ensemble von Algorithmen für maschinelles Lernen ist die Verwendung mehrerer (nicht unbedingt unterschiedlicher) Modelle anstelle eines. Das heißt, wir trainieren zuerst jedes Modell und kombinieren dann ihre Vorhersagen. Es stellt sich heraus, dass unsere Modelle zusammen ein komplexeres Modell bilden (in Bezug auf die Verallgemeinerungsfähigkeit - die Fähigkeit, Daten zu "verstehen"), das oft als Metamodell bezeichnet wird . Am häufigsten wird das Metamodell nicht anhand unserer anfänglichen Datenstichprobe, sondern anhand der Vorhersagen anderer Modelle trainiert. Es scheint die Erfahrung aller Modelle zu berücksichtigen, und dies hilft, Fehler zu reduzieren.
Planen
- Zuerst schauen wir uns ein einfaches PyTorch-Modell an und erhalten dessen Qualität.
- Dann werden wir mehrere Modelle mit Scikit-learn zusammenbauen und lernen, wie man ein höheres Level erreicht
Ein einziges Modell
, Digits Sklearn, :
from sklearn.datasets import load_digits
import numpy as np
import matplotlib.pyplot as plt
x, y = load_digits(n_class=10, return_X_y=True)
, :
print(x.shape)
>>> (1797, 64)
print(np.unique(y))
>>> array([0, 1, 2, 3, 4, 5, 6, 7, 8, 9])
index = 0
print(y[index])
plt.imshow(x[index].reshape(8, 8), cmap="Greys")
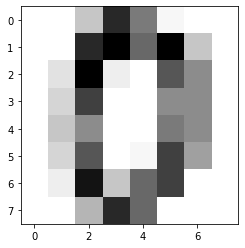
x[index]
>>> array([ 0., 0., 5., 13., 9., 1., 0., 0., 0., 0., 13., 15., 10.,
15., 5., 0., 0., 3., 15., 2., 0., 11., 8., 0., 0., 4.,
12., 0., 0., 8., 8., 0., 0., 5., 8., 0., 0., 9., 8.,
0., 0., 4., 11., 0., 1., 12., 7., 0., 0., 2., 14., 5.,
10., 12., 0., 0., 0., 0., 6., 13., 10., 0., 0., 0.])
, 0 15. , :
from sklearn.model_selection import train_test_split
from sklearn.preprocessing import StandardScaler
x_train, x_test, y_train, y_test = train_test_split(x, y, test_size=0.2,
shuffle=True, random_state=42)
print(x_train.shape)
>>> (1437, 64)
print(x_test.shape)
>>> (360, 64)
StandardScaler: -1 1. , — score score' :
scaler = StandardScaler()
scaler.fit(x_train)
:
x_train_scaled = scaler.transform(x_train)
x_test_scaled = scaler.transform(x_test)
Torch'!
import torch
from torch.utils.data import TensorDataset, DataLoader
import torch.nn as nn
import torch.nn.functional as F
from torch.optim import Adam
:
class SimpleCNN(nn.Module):
def __init__(self):
super(SimpleCNN, self).__init__()
self.conv1 = nn.Conv2d(in_channels=1, out_channels=3, kernel_size=5, stride=1, padding=2)
self.conv1_s = nn.Conv2d(in_channels=3, out_channels=3, kernel_size=5, stride=2, padding=2)
self.conv2 = nn.Conv2d(in_channels=3, out_channels=6, kernel_size=3, stride=1, padding=1)
self.conv2_s = nn.Conv2d(in_channels=6, out_channels=6, kernel_size=3, stride=2, padding=1)
self.conv3 = nn.Conv2d(in_channels=6, out_channels=10, kernel_size=3, stride=1, padding=1)
self.conv3_s = nn.Conv2d(in_channels=10, out_channels=10, kernel_size=3, stride=2, padding=1)
self.flatten = nn.Flatten()
self.fc1 = nn.Linear(10, 10)
def forward(self, x):
x = F.relu(self.conv1(x))
x = F.relu(self.conv1_s(x))
x = F.relu(self.conv2(x))
x = F.relu(self.conv2_s(x))
x = F.relu(self.conv3(x))
x = F.relu(self.conv3_s(x))
x = self.flatten(x)
x = self.fc1(x)
x = F.softmax(x)
return x
:
batch_size = 64
learning_rate = 1e-3
epochs = 200
, PyTorch:
x_train_tensor = torch.tensor(x_train_scaled.reshape(-1, 1, 8, 8).astype(np.float32))
x_test_tensor = torch.tensor(x_test_scaled.reshape(-1, 1, 8, 8).astype(np.float32))
y_train_tensor = torch.tensor(y_train.astype(np.long))
y_test_tensor = torch.tensor(y_test.astype(np.long))
PyTorch , — . — , X y ( ), DataLoader — , <X, y> 64 :
train_dataset = TensorDataset(x_train_tensor, y_train_tensor)
train_loader = DataLoader(train_dataset, batch_size=batch_size)
test_dataset = TensorDataset(x_test_tensor, y_test_tensor)
test_loader = DataLoader(test_dataset, batch_size=batch_size)
, :
simple_cnn = SimpleCNN().cuda()
criterion = nn.CrossEntropyLoss()
optimizer = Adam(simple_cnn.parameters(), lr=learning_rate)
:
for epoch in range(epochs):
simple_cnn.train()
train_samples_count = 0
true_train_samples_count = 0
running_loss = 0
for batch in train_loader:
x_data = batch[0].cuda()
y_data = batch[1].cuda()
y_pred = simple_cnn(x_data)
loss = criterion(y_pred, y_data)
optimizer.zero_grad()
loss.backward()
optimizer.step()
running_loss += loss.item()
y_pred = y_pred.argmax(dim=1, keepdim=False)
true_classified = (y_pred == y_data).sum().item()
true_train_samples_count += true_classified
train_samples_count += len(x_data)
train_accuracy = true_train_samples_count / train_samples_count
print(f"[{epoch}] train loss: {running_loss}, accuracy: {round(train_accuracy, 4)}")
simple_cnn.eval()
test_samples_count = 0
true_test_samples_count = 0
running_loss = 0
for batch in test_loader:
x_data = batch[0].cuda()
y_data = batch[1].cuda()
y_pred = simple_cnn(x_data)
loss = criterion(y_pred, y_data)
loss.backward()
running_loss += loss.item()
y_pred = y_pred.argmax(dim=1, keepdim=False)
true_classified = (y_pred == y_data).sum().item()
true_test_samples_count += true_classified
test_samples_count += len(x_data)
test_accuracy = true_test_samples_count / test_samples_count
print(f"[{epoch}] test loss: {running_loss}, accuracy: {round(test_accuracy, 4)}")
,- Torch' , . . 20 .
[180] train loss: 40.52328181266785, accuracy: 0.6966
[180] test loss: 10.813781499862671, accuracy: 0.6583
[181] train loss: 40.517325043678284, accuracy: 0.6966
[181] test loss: 10.811877608299255, accuracy: 0.6611
[182] train loss: 40.517088294029236, accuracy: 0.6966
[182] test loss: 10.814386487007141, accuracy: 0.6611
[183] train loss: 40.515315651893616, accuracy: 0.6966
[183] test loss: 10.812204122543335, accuracy: 0.6611
[184] train loss: 40.5108939409256, accuracy: 0.6966
[184] test loss: 10.808713555335999, accuracy: 0.6639
[185] train loss: 40.50885498523712, accuracy: 0.6966
[185] test loss: 10.80833113193512, accuracy: 0.6639
[186] train loss: 40.50892996788025, accuracy: 0.6966
[186] test loss: 10.809209108352661, accuracy: 0.6639
[187] train loss: 40.508036971092224, accuracy: 0.6966
[187] test loss: 10.806900978088379, accuracy: 0.6667
[188] train loss: 40.507275462150574, accuracy: 0.6966
[188] test loss: 10.79791784286499, accuracy: 0.6611
[189] train loss: 40.50368785858154, accuracy: 0.6966
[189] test loss: 10.799399137496948, accuracy: 0.6667
[190] train loss: 40.499858379364014, accuracy: 0.6966
[190] test loss: 10.795265793800354, accuracy: 0.6611
[191] train loss: 40.498780846595764, accuracy: 0.6966
[191] test loss: 10.796114206314087, accuracy: 0.6639
[192] train loss: 40.497228503227234, accuracy: 0.6966
[192] test loss: 10.790620803833008, accuracy: 0.6639
[193] train loss: 40.44325613975525, accuracy: 0.6973
[193] test loss: 10.657087206840515, accuracy: 0.7
[194] train loss: 39.62049174308777, accuracy: 0.7495
[194] test loss: 10.483307123184204, accuracy: 0.7222
[195] train loss: 39.24516046047211, accuracy: 0.7613
[195] test loss: 10.462445378303528, accuracy: 0.7278
[196] train loss: 39.16947162151337, accuracy: 0.762
[196] test loss: 10.488057255744934, accuracy: 0.7222
[197] train loss: 39.196797251701355, accuracy: 0.7634
[197] test loss: 10.502906918525696, accuracy: 0.7222
[198] train loss: 39.395434617996216, accuracy: 0.7537
[198] test loss: 10.354896545410156, accuracy: 0.7472
[199] train loss: 39.331292152404785, accuracy: 0.7509
[199] test loss: 10.367400050163269, accuracy: 0.7389
, : .
, : 74% . Not great, not terrible.
!
Bagging. : , , . : "" , - . .
sklearn ensembles
, . — , BaggingClassifier
:
import sklearn
from sklearn.ensemble import BaggingClassifier
. sklearn, — , sklearn.base.BaseEstimator
. , . fit
( ), predict_proba
, ( , ), predict
( ), .
, "" .
. , , .
-, ( 2 — get_params
set_params
), , . , net_type
, __init__
net_type
.
-, , , ( sklearn , ) copy
, deepcopy
. , , () , , .
class PytorchModel(sklearn.base.BaseEstimator):
def __init__(self, net_type, net_params, optim_type, optim_params, loss_fn,
input_shape, batch_size=32, accuracy_tol=0.02, tol_epochs=10,
cuda=True):
self.net_type = net_type
self.net_params = net_params
self.optim_type = optim_type
self.optim_params = optim_params
self.loss_fn = loss_fn
self.input_shape = input_shape
self.batch_size = batch_size
self.accuracy_tol = accuracy_tol
self.tol_epochs = tol_epochs
self.cuda = cuda
: fit
. , — , Loss, . : , ? . , 200 ( ). , . — , accuracy . , accuracy . , (tol_epochs
) ( accuracy , accuracy_tol
).
def fit(self, X, y):
self.net = self.net_type(**self.net_params)
if self.cuda:
self.net = self.net.cuda()
self.optim = self.optim_type(self.net.parameters(), **self.optim_params)
uniq_classes = np.sort(np.unique(y))
self.classes_ = uniq_classes
X = X.reshape(-1, *self.input_shape)
x_tensor = torch.tensor(X.astype(np.float32))
y_tensor = torch.tensor(y.astype(np.long))
train_dataset = TensorDataset(x_tensor, y_tensor)
train_loader = DataLoader(train_dataset, batch_size=self.batch_size,
shuffle=True, drop_last=False)
last_accuracies = []
epoch = 0
keep_training = True
while keep_training:
self.net.train()
train_samples_count = 0
true_train_samples_count = 0
for batch in train_loader:
x_data, y_data = batch[0], batch[1]
if self.cuda:
x_data = x_data.cuda()
y_data = y_data.cuda()
y_pred = self.net(x_data)
loss = self.loss_fn(y_pred, y_data)
self.optim.zero_grad()
loss.backward()
self.optim.step()
y_pred = y_pred.argmax(dim=1, keepdim=False)
true_classified = (y_pred == y_data).sum().item()
true_train_samples_count += true_classified
train_samples_count += len(x_data)
train_accuracy = true_train_samples_count / train_samples_count
last_accuracies.append(train_accuracy)
if len(last_accuracies) > self.tol_epochs:
last_accuracies.pop(0)
if len(last_accuracies) == self.tol_epochs:
accuracy_difference = max(last_accuracies) - min(last_accuracies)
if accuracy_difference <= self.accuracy_tol:
keep_training = False
. — , Loss, - :
def predict_proba(self, X, y=None):
X = X.reshape(-1, *self.input_shape)
x_tensor = torch.tensor(X.astype(np.float32))
if y:
y_tensor = torch.tensor(y.astype(np.long))
else:
y_tensor = torch.zeros(len(X), dtype=torch.long)
test_dataset = TensorDataset(x_tensor, y_tensor)
test_loader = DataLoader(test_dataset, batch_size=self.batch_size,
shuffle=False, drop_last=False)
self.net.eval()
predictions = []
for batch in test_loader:
x_data, y_data = batch[0], batch[1]
if self.cuda:
x_data = x_data.cuda()
y_data = y_data.cuda()
y_pred = self.net(x_data)
predictions.append(y_pred.detach().cpu().numpy())
predictions = np.concatenate(predictions)
return predictions
:
def predict(self, X, y=None):
predictions = self.predict_proba(X, y)
predictions = predictions.argmax(axis=1)
return predictions
class PytorchModel(sklearn.base.BaseEstimator):
def __init__(self, net_type, net_params, optim_type, optim_params, loss_fn,
input_shape, batch_size=32, accuracy_tol=0.02, tol_epochs=10,
cuda=True):
self.net_type = net_type
self.net_params = net_params
self.optim_type = optim_type
self.optim_params = optim_params
self.loss_fn = loss_fn
self.input_shape = input_shape
self.batch_size = batch_size
self.accuracy_tol = accuracy_tol
self.tol_epochs = tol_epochs
self.cuda = cuda
def fit(self, X, y):
self.net = self.net_type(**self.net_params)
if self.cuda:
self.net = self.net.cuda()
self.optim = self.optim_type(self.net.parameters(), **self.optim_params)
uniq_classes = np.sort(np.unique(y))
self.classes_ = uniq_classes
X = X.reshape(-1, *self.input_shape)
x_tensor = torch.tensor(X.astype(np.float32))
y_tensor = torch.tensor(y.astype(np.long))
train_dataset = TensorDataset(x_tensor, y_tensor)
train_loader = DataLoader(train_dataset, batch_size=self.batch_size,
shuffle=True, drop_last=False)
last_accuracies = []
epoch = 0
keep_training = True
while keep_training:
self.net.train()
train_samples_count = 0
true_train_samples_count = 0
for batch in train_loader:
x_data, y_data = batch[0], batch[1]
if self.cuda:
x_data = x_data.cuda()
y_data = y_data.cuda()
y_pred = self.net(x_data)
loss = self.loss_fn(y_pred, y_data)
self.optim.zero_grad()
loss.backward()
self.optim.step()
y_pred = y_pred.argmax(dim=1, keepdim=False)
true_classified = (y_pred == y_data).sum().item()
true_train_samples_count += true_classified
train_samples_count += len(x_data)
train_accuracy = true_train_samples_count / train_samples_count
last_accuracies.append(train_accuracy)
if len(last_accuracies) > self.tol_epochs:
last_accuracies.pop(0)
if len(last_accuracies) == self.tol_epochs:
accuracy_difference = max(last_accuracies) - min(last_accuracies)
if accuracy_difference <= self.accuracy_tol:
keep_training = False
def predict_proba(self, X, y=None):
X = X.reshape(-1, *self.input_shape)
x_tensor = torch.tensor(X.astype(np.float32))
if y:
y_tensor = torch.tensor(y.astype(np.long))
else:
y_tensor = torch.zeros(len(X), dtype=torch.long)
test_dataset = TensorDataset(x_tensor, y_tensor)
test_loader = DataLoader(test_dataset, batch_size=self.batch_size,
shuffle=False, drop_last=False)
self.net.eval()
predictions = []
for batch in test_loader:
x_data, y_data = batch[0], batch[1]
if self.cuda:
x_data = x_data.cuda()
y_data = y_data.cuda()
y_pred = self.net(x_data)
predictions.append(y_pred.detach().cpu().numpy())
predictions = np.concatenate(predictions)
return predictions
def predict(self, X, y=None):
predictions = self.predict_proba(X, y)
predictions = predictions.argmax(axis=1)
return predictions
, :
base_model = PytorchModel(net_type=SimpleCNN, net_params=dict(), optim_type=Adam,
optim_params={"lr": 1e-3}, loss_fn=nn.CrossEntropyLoss(),
input_shape=(1, 8, 8), batch_size=32, accuracy_tol=0.02,
tol_epochs=10, cuda=True)
base_model.fit(x_train_scaled, y_train)
preds = base_model.predict(x_test_scaled)
true_classified = (preds == y_test).sum()
test_accuracy = true_classified / len(y_test)
print(f"Test accuracy: {test_accuracy}")
>>> Test accuracy: 0.7361111111111112
.
meta_classifier = BaggingClassifier(base_estimator=base_model, n_estimators=10)
meta_classifier.fit(x_train_scaled.reshape(-1, 64), y_train)
>>> BaggingClassifier(
base_estimator=PytorchModel(accuracy_tol=0.02, batch_size=32,
cuda=True, input_shape=(1, 8, 8),
loss_fn=CrossEntropyLoss(),
net_params={},
net_type=<class '__main__.SimpleCNN'>,
optim_params={'lr': 0.001},
optim_type=<class 'torch.optim.adam.Adam'>,
tol_epochs=10),
bootstrap=True, bootstrap_features=False, max_features=1.0,
max_samples=1.0, n_estimators=10, n_jobs=None,
oob_score=False, random_state=None, verbose=0,
warm_start=False)
score
accuracy :
print(meta_classifier.score(x_test_scaled.reshape(-1, 64), y_test))
>>> 0.95
, . . . , , ( "" - ).
?
-, . , Loss' - .
-, Boosting. Bagging' , , . ( ) . sklearn, Loss - .
, . , "" . , .
, . — - , , , , , .
. , Data science' . Computer vision, . ( — !) — FARADAY Lab. — , .
c: