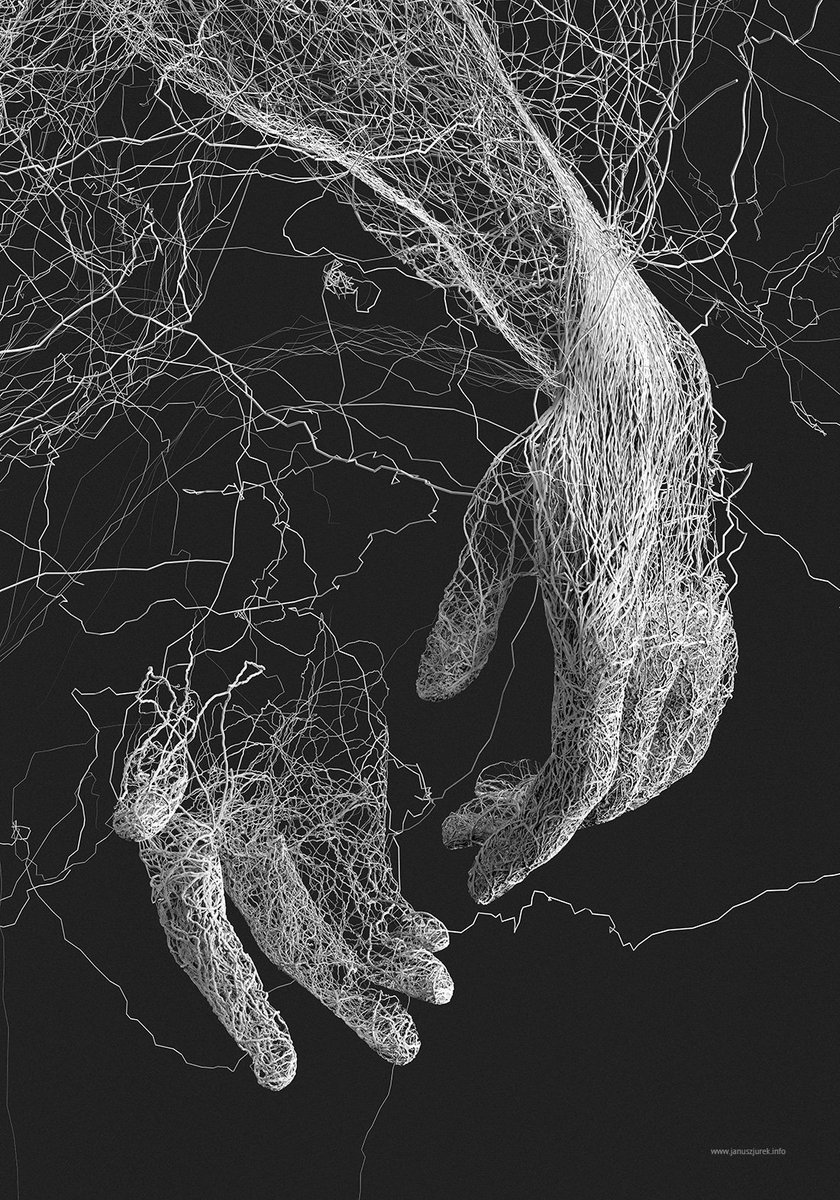
(صورة مولدة)
هناك طرق عديدة لإنشاء شبكة عصبية اصطناعية أو حتى "ذكاء اصطناعي". لكن كل هذه الأساليب محبطة ، من جانب التعقيد الذي لا أفهمه بالكامل ، جزئياً من حقيقة أن كل شيء يأتي إلى صيغ رياضية.
لا حرج في مثل هذه الأساليب ؛ فهي تساعد في حل المهام الموكلة إليها. ولكن يبدو أنني أريد حقًا كتابة دراجة.

, ?
, , .
.
, , , 1 0 . 1 0 . , , .

+- . , ? , — ? " "?

Axon guidance.
, .
:
Eph-
: , , ;
: CRMP- (CRMP1, CRMP2, CRMP3, CRMP4, CRMP5)
, , . . .
. . - , ?

( )
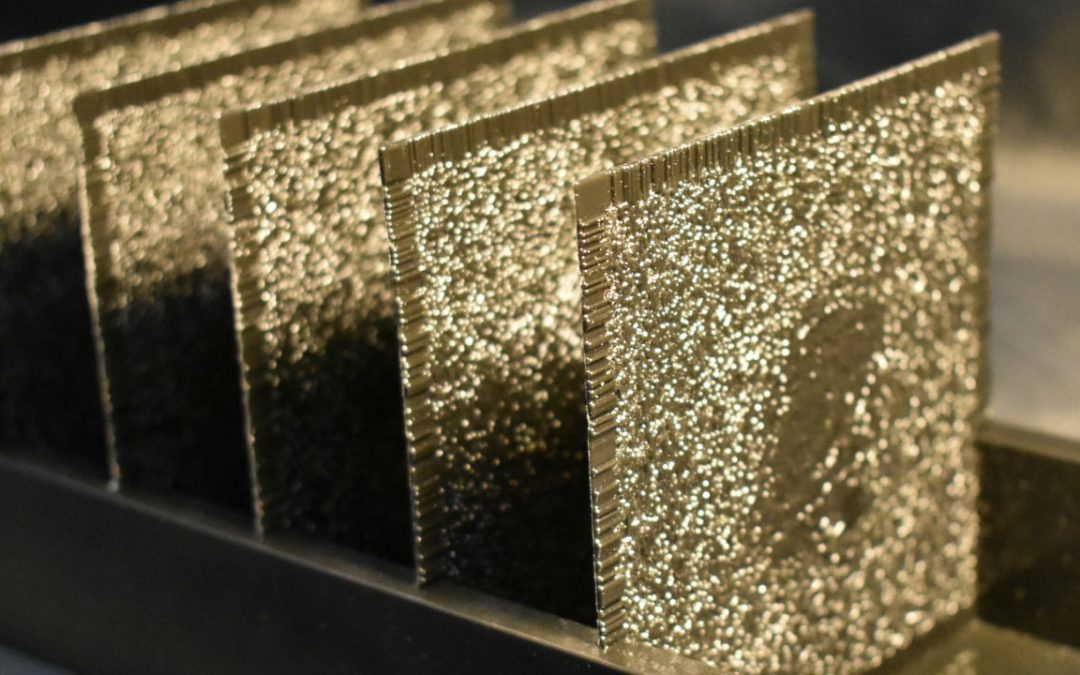
( 3 — )
, , , , , - .
, , — . — - , .
. , .
.
« » , . , , , , .
. , , « » . : . . , ? « » . ? ? ? ? , . , , . , , ? ? ? , ? , «» , . .
?
1950- , , ( «GA»), , , Adaptation in Natural and Artificial Systems GA. , « ».
, . , . , . Java, .

« » : , , ( ). , , , , , , .
. , : . , — .
«to be or not to be that is the question» ( «to be or not to be: that is the question»). 39 . , , — 1 27. — 1 27 * 27. , , :
1 66,555,937,033,867,822,607,895,549,241,096,482,953,017,615,834,735,226,163.
— , 9 719 096 182 010 563 073 122 (253 593 605 017 ). ( 13 750 000 000 .)
, , , , ( ) "to be or not to be that is the question". .
, , , , , . , .
, . , , :
. , . , , .
().
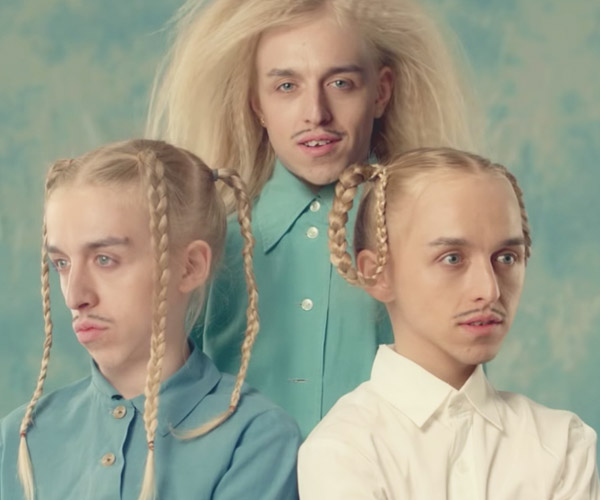
, -, . , , , : , , , . - . , .
(). , . « ». , , . , , , . « » . , , . , , , , . , «» ( , ) « ». — , «to be or not to be».
—
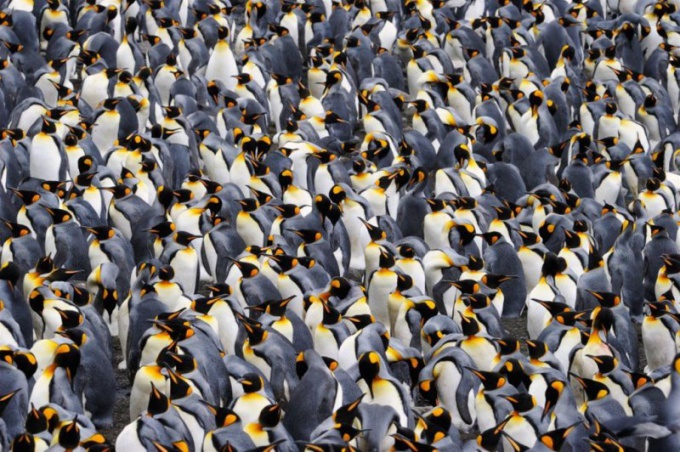
, , . , ( ). . cat :
hug
rid
won
, , , . , . , , , , "c" , "a" , "a" . , , , ( ).
, — .
, , . .

( () ())

( () ())
. , , . , ANSI genes.
— — . , +- , .
—
— ,
.
1.
, , , . fitness, fit.
(Fitness function). , . , . , , .
, . cat car:
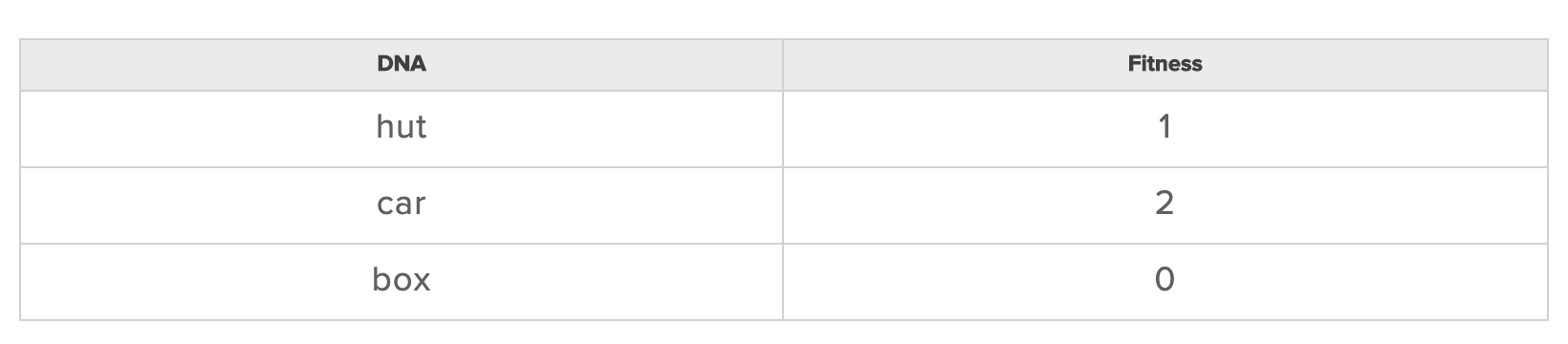
2. ( )
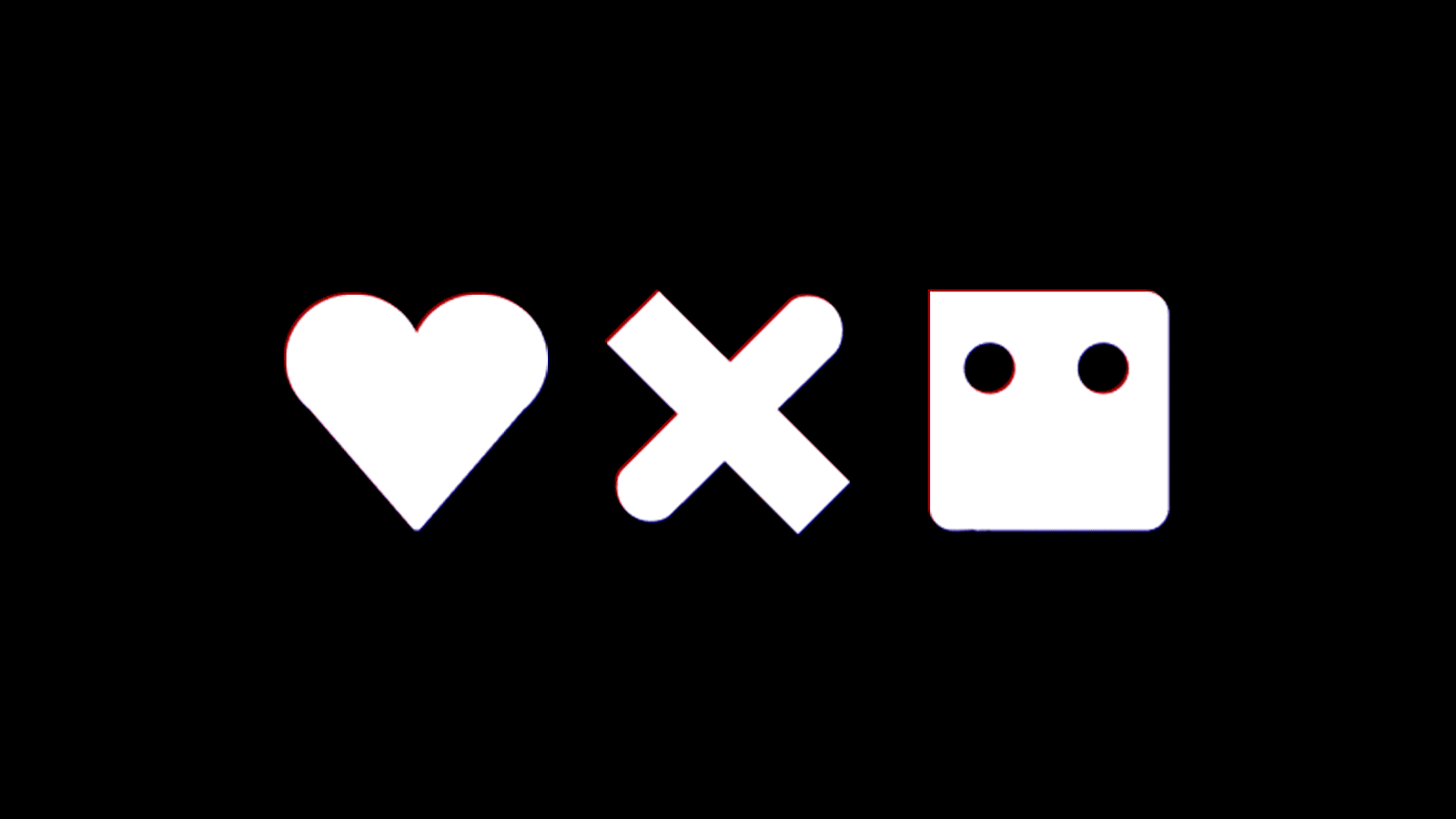
, .
. , : " ? ".
, . ( ) , , . , 500 1000. , . , . 500 , 501 ?
, « » ( «»). , , , :
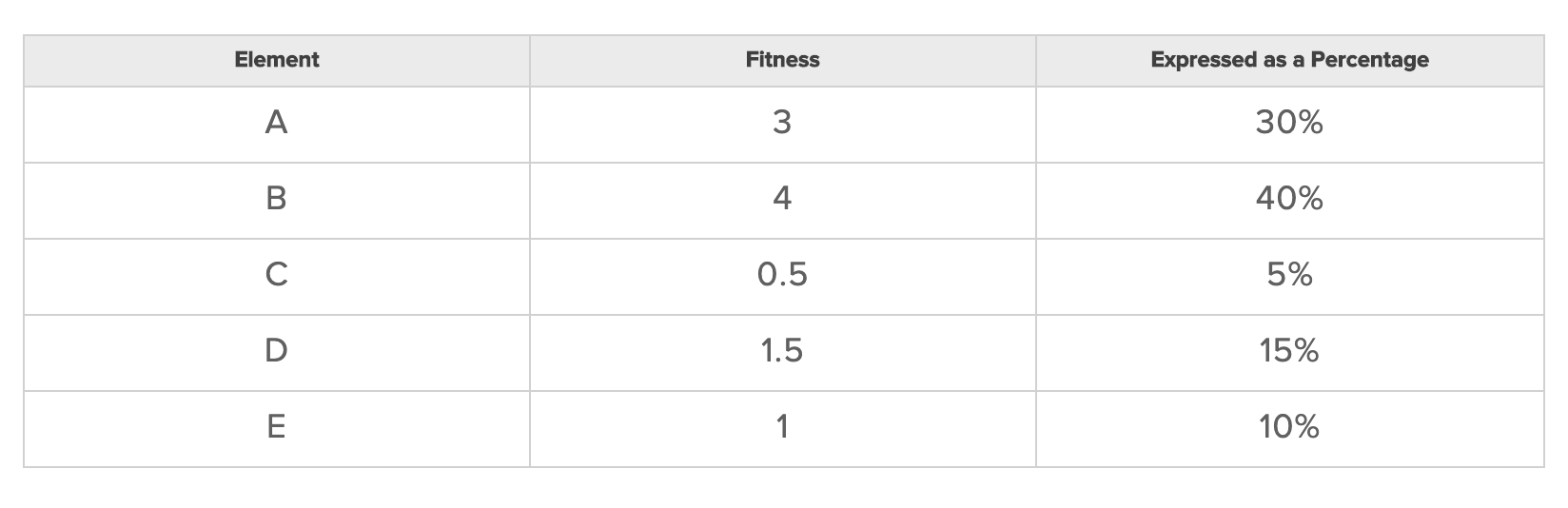
:
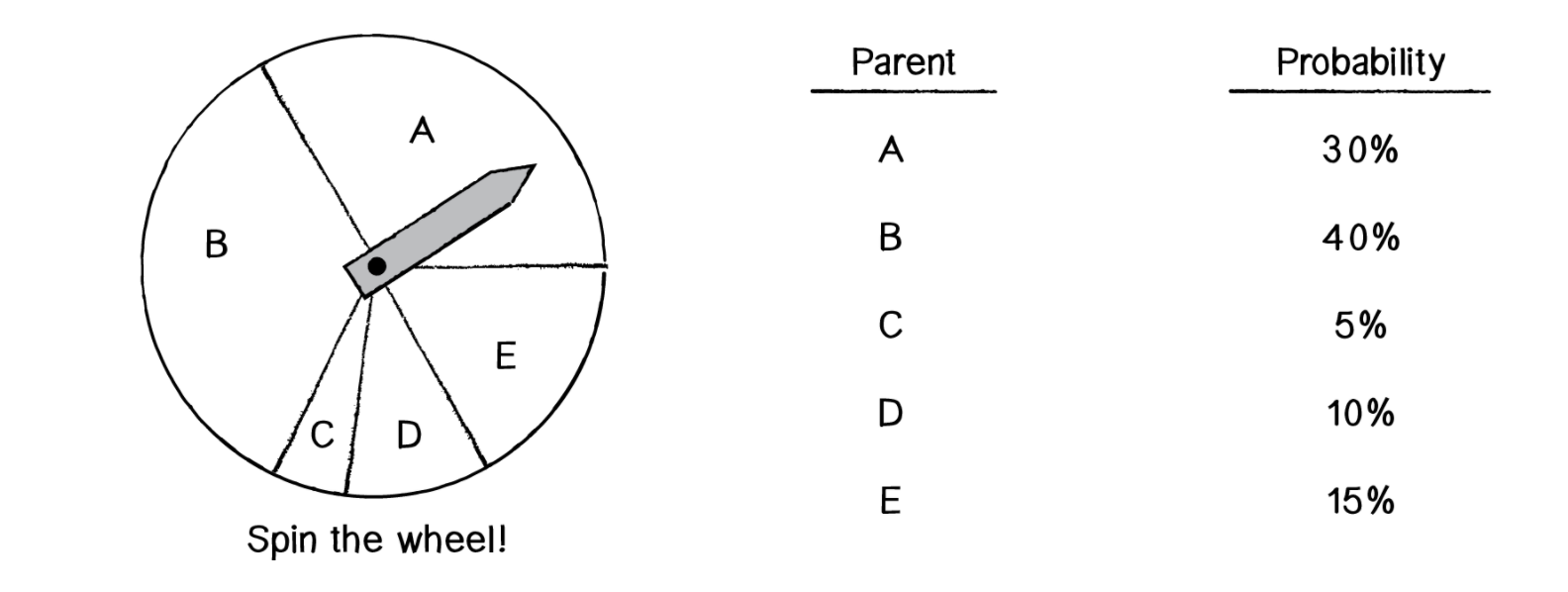
, B . , C . . "to be or not to be":
A: to be or not to go
B: to be or not to pi
C: xxxxxxxxxxxxxxxxbe
B , C . .
, . . .
—
. 5 . :
1. (Crossover)
. , , .
A: FORK
B: PLAY
. , ( 50/50) — A B, :
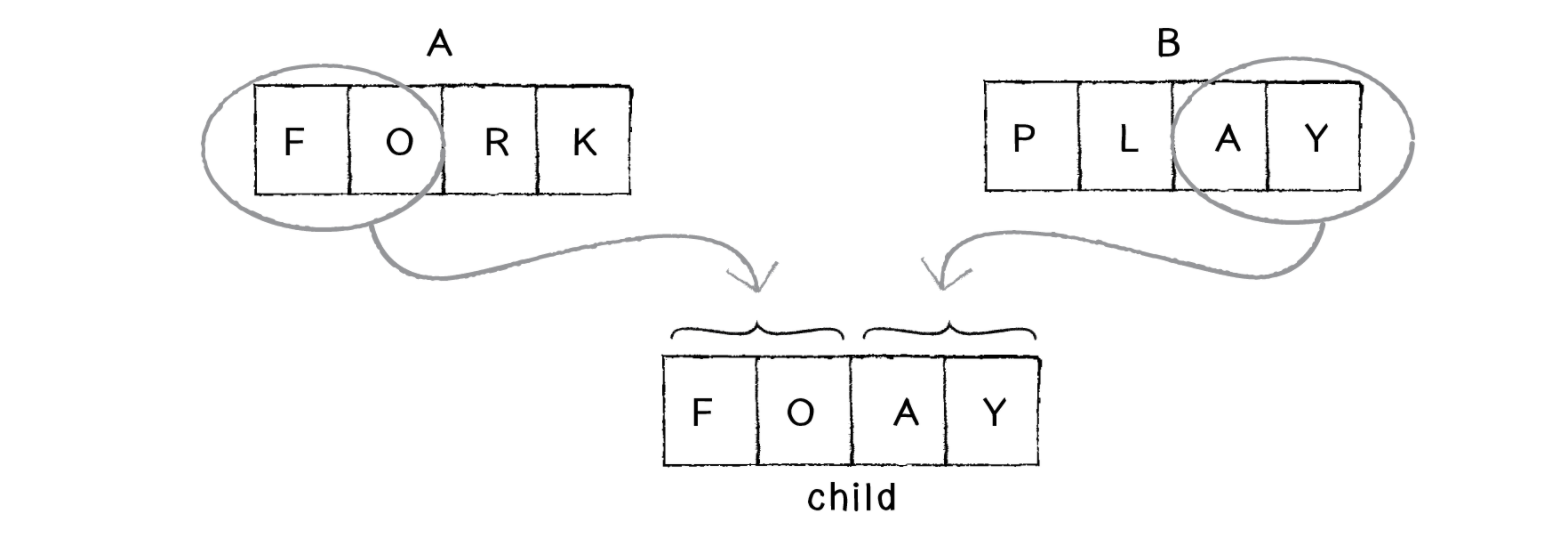
. :
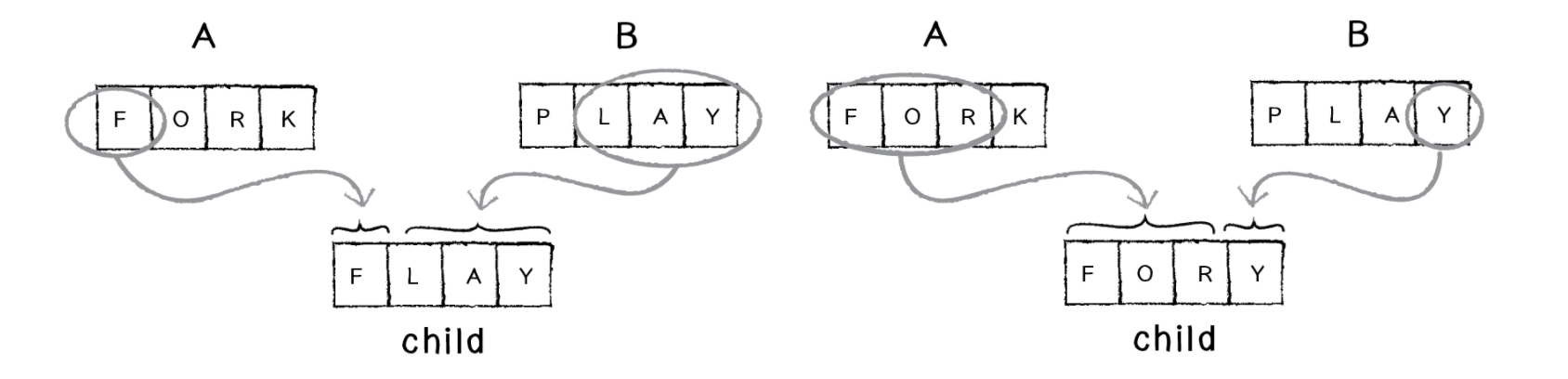
:

, . . , -. .
2.
— . , . . . , , . . .
. FORY , 1%, , FORY 1% . 96% . , :

, , "" .
:
SETUP:
1: , N . .
LOOP:
2: , .
3: . N :
4. 2.
. , .
Python. , JavaScript.
JS , . Node.js. , , . :)
DNA:
const random = require('./random');
class DNA {
constructor(length, dna = null) {
this.length = length;
this.genes = dna ? dna.genes : this.getRandomGenes(this.length);
this.fitness = 0;
}
getRandomGenes(length) {
return new Array(length)
.fill(0)
.map(() => random.getRandomInt(32, 128));
}
updateLength(length) {
if (length <= this.genes) {
throw 'Error';
}
this.genes = [...this.genes, this.getRandomGenes(length - this.genes.length)];
}
}
module.exports = DNA;
DNA , , .
getRandomInt 32 128 . ANSI .
function getRandomInt(min, max) {
min = Math.ceil(min);
max = Math.floor(max);
return Math.floor(Math.random() * (max - min)) + min;
}
module.exports = {
random: Math.random,
getRandomInt
}
DNA.js genes. .
. , , .
initPopulation() {
this.population = new Array(this.n)
.fill(0)
.map(() => new this.dna(this.target.length));
this.population.forEach((dna) => dna.fitness = this.evaluateFitness(dna));
}
n, . . , .
evaluateFitness(dna) {
return (dna.genes.reduce((acc, current, index) => {
if (current === this.target.codePointAt(index)) {
return acc + 1;
}
return acc;
}, 0) / this.target.length);
}
, . this.target
. , .
getMatingPool() {
let matingPool = [];
this.population.forEach((dna) => {
const n = parseInt(dna.fitness * 100);
matingPool = [...matingPool, ...(new Array(n).fill(dna))];
});
return matingPool;
}
, . , . , .
findRandomParent(matingPool) {
return matingPool[random.getRandomInt(0, matingPool.length)];
}
crossover(dna1, dna2) {
const child = new this.dna(this.target.length);
const midpoint = random.getRandomInt(0, dna1.genes.length);
for (let i = 0; i < dna1.genes.length; i++) {
if (i > midpoint) {
child.genes[i] = dna1.genes[i];
}
else {
child.genes[i] = dna2.genes[i];
}
}
return child;
}
, , .
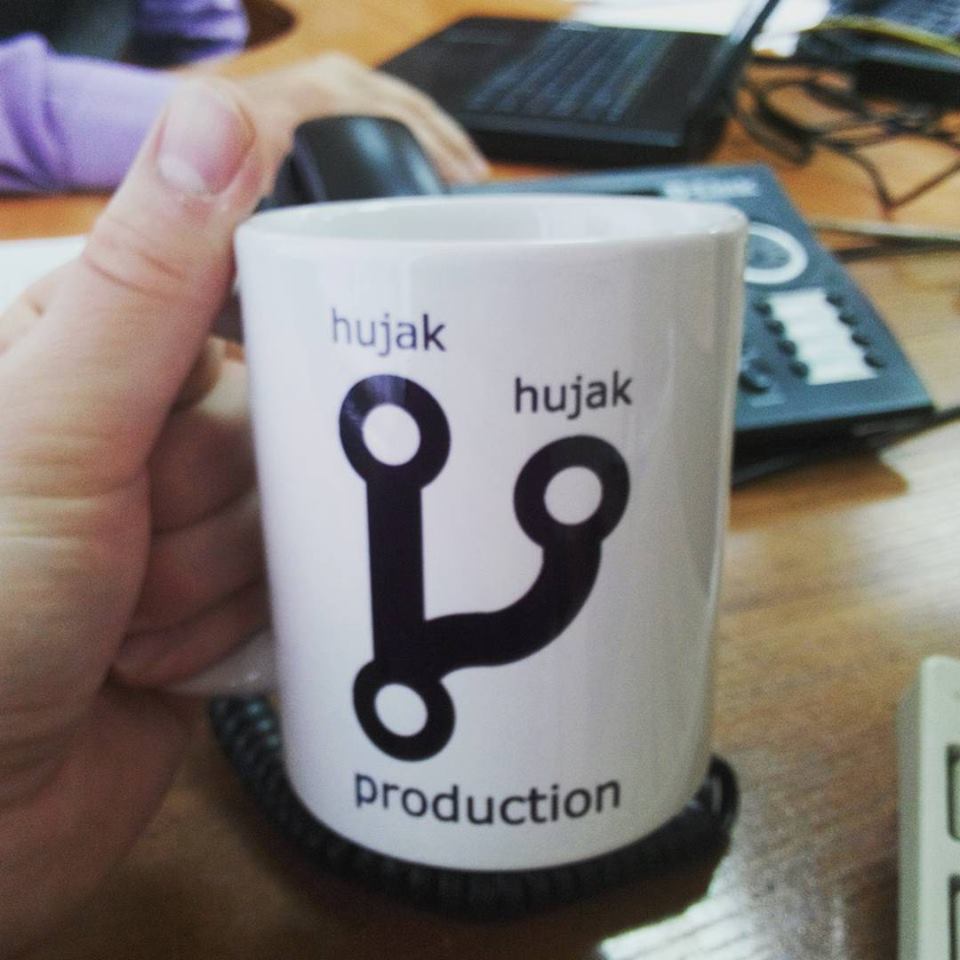
:
mutation(dna) {
const mutatedDNA = new this.dna(this.target.length, dna);
for (let i = 0; i < dna.genes.length; i++) {
if (random.random() < this.mutationRate) {
mutatedDNA.genes[i] = random.getRandomInt(32, 128);
}
}
return mutatedDNA;
}
, .
:
const random = require('./random');
class Population {
constructor(target, dna, n = 100, mutationRate = 0.01, adaptive = false, adaptiveThreshold = 0.6, cutLength = 5) {
this.endTarget = target;
this.adaptive = adaptive;
this.adaptiveThreshold = adaptiveThreshold;
this.cutLength = cutLength;
this.target = this.adaptive ? target.substr(0, this.cutLength) : target;
this.dna = dna;
this.n = n;
this.mutationRate = mutationRate;
this.population = []
this.populationInfo = {
generationCount: 0
};
this.initPopulation();
}
initPopulation() {
this.population = new Array(this.n)
.fill(0)
.map(() => new this.dna(this.target.length));
this.population.forEach((dna) => dna.fitness = this.evaluateFitness(dna));
}
updateDNA(target) {
this.target = target;
this.population.forEach((dna) => {
dna.updateLength(target.length);
});
}
getPopulationInfo() {
let statsFitness1 = 0;
return {
generationCount: this.populationInfo.generationCount,
averageFitness: this.population
.reduce((acc, current) => {
if (this.target === String.fromCharCode.apply(this, current.genes)) {
statsFitness1 += 1;
}
return acc + current.fitness
}, 0) / this.population.length,
statsFitness1
}
}
getMatingPool() {
let matingPool = [];
this.population.forEach((dna) => {
const n = parseInt(dna.fitness * 100);
matingPool = [...matingPool, ...(new Array(n).fill(dna))];
});
return matingPool;
}
evaluateFitness(dna) {
return (dna.genes.reduce((acc, current, index) => {
if (current === this.target.codePointAt(index)) {
return acc + 1;
}
return acc;
}, 0) / this.target.length);
}
findRandomParent(matingPool) {
return matingPool[random.getRandomInt(0, matingPool.length)];
}
nextGeneration() {
let matingPool = this.getMatingPool();
this.population.forEach((_, index) => {
const parentA = this.findRandomParent(matingPool);
let parentB = null;
while (!parentB || (parentB === parentA)) {
parentB = this.findRandomParent(matingPool);
}
let child = this.crossover(parentA, parentB);
child = this.mutation(child);
child.fitness = this.evaluateFitness(child)
this.population[index] = child;
});
this.populationInfo.generationCount += 1;
if (this.adaptive && this.target !== this.endTarget) {
if (this.getPopulationInfo().averageFitness >= this.adaptiveThreshold) {
this.updateDNA(this.endTarget.substr(0, this.target.length + this.cutLength));
}
}
}
crossover(dna1, dna2) {
const child = new this.dna(this.target.length);
const midpoint = random.getRandomInt(0, dna1.genes.length);
for (let i = 0; i < dna1.genes.length; i++) {
if (i > midpoint) {
child.genes[i] = dna1.genes[i];
}
else {
child.genes[i] = dna2.genes[i];
}
}
return child;
}
mutation(dna) {
const mutatedDNA = new this.dna(this.target.length, dna);
for (let i = 0; i < dna.genes.length; i++) {
if (random.random() < this.mutationRate) {
mutatedDNA.genes[i] = random.getRandomInt(32, 128);
}
}
return mutatedDNA;
}
}
module.exports = Population;
, . , . Node.js WebGL , , -, — .
blessed-contrib .
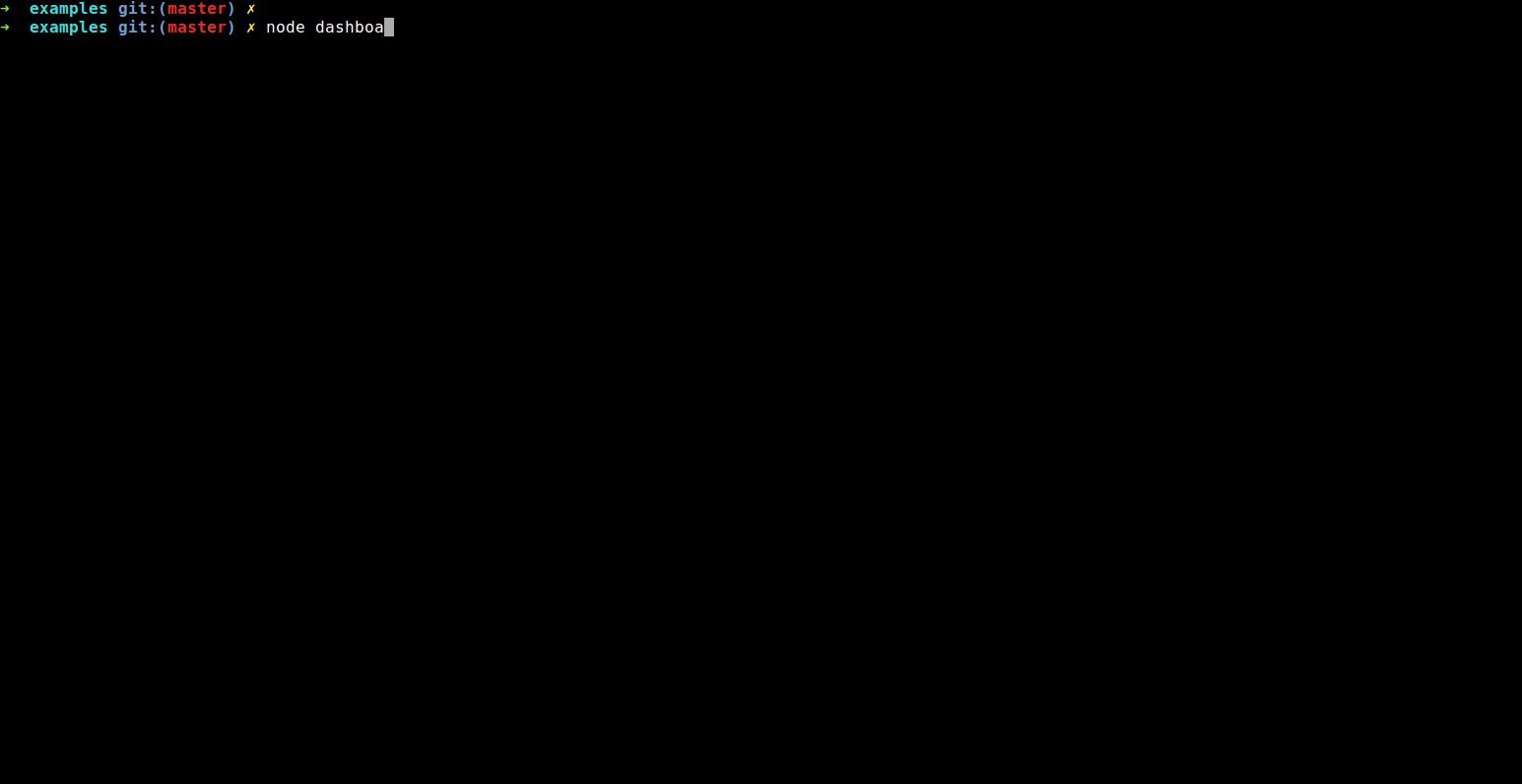
, . , .
,
const blessed = require('blessed');
const contrib = require('blessed-contrib');
class Log {
constructor(rows, cols) {
this.lines = [];
this.screen = blessed.screen();
this.grid = new contrib.grid({
rows,
cols,
screen: this.screen
});
this.screen.on('resize', () => {
this.lines.forEach((line) => {
line.emit('attach');
})
});
this.screen.key(['escape', 'q', 'C-c'], function(ch, key) {
return process.exit(0);
});
}
addLine(x, label, row, col) {
const line = this.grid.set(row, col, 1, 1, contrib.line, {
label,
style: {
line: "yellow",
text: "green",
baseline: "black"
},
xLabelPadding: 3,
xPadding: 5
});
this.screen.append(line);
line.setData([{
x: x,
y: x.map(v => v * 100)
}]);
return this.lines.push(line) - 1;
}
updateLineData(index, x) {
this.lines[index]
.setData([{
x,
y: x
}]
);
}
render() {
this.screen.render();
}
}
module.exports = Log;
, , 100% .
getPopulationInfo() {
let statsFitness1 = 0;
return {
generationCount: this.populationInfo.generationCount,
averageFitness: this.population
.reduce((acc, current) => {
if (this.target === String.fromCharCode.apply(this, current.genes)) {
statsFitness1 += 1;
}
return acc + current.fitness
}, 0) / this.population.length,
statsFitness1
}
}
, .
const DNA = require('./DNA');
const Population = require('./Population');
const Log = require('./Log');
const contrib = require('blessed-contrib');
const log = new Log(2, 2);
const statsFitnessAverageIndex = log.addLine([], 'Fitness', 0, 0);
const statsFitness1Index = log.addLine([], 'Fitness 1', 0, 1);
const screenLog = log.grid.set(1, 1, 1, 1, contrib.log, {
label: 'logs'
});
const TARGET = 'Hello Habr!';
const GENERATIONS = 1000;
const POPULATION_NUMBER = 150;
const MUTATION_RATE = 0.01;
const population = new Population(TARGET, DNA, POPULATION_NUMBER, MUTATION_RATE);
const statsFitnessAverage = [];
const statsFitness1 = [];
, .
...
function main() {
population.nextGeneration();
const stats = population.getPopulationInfo();
statsFitnessAverage.push(stats.averageFitness);
statsFitness1.push(stats.statsFitness1);
}
. , .
function update() {
log.updateLineData(statsFitnessAverageIndex, statsFitnessAverage);
log.updateLineData(statsFitness1Index, statsFitness1);
}
function sync() {
for (let i = 0; i < GENERATIONS; i++) {
main();
}
update();
log.render();
}
sync();
screenLog.log(`${population.endTarget} : ${population.target}`)
sync
. , :
function async() {
setInterval(() => {
main();
update();
log.render();
}, 10)
}
:
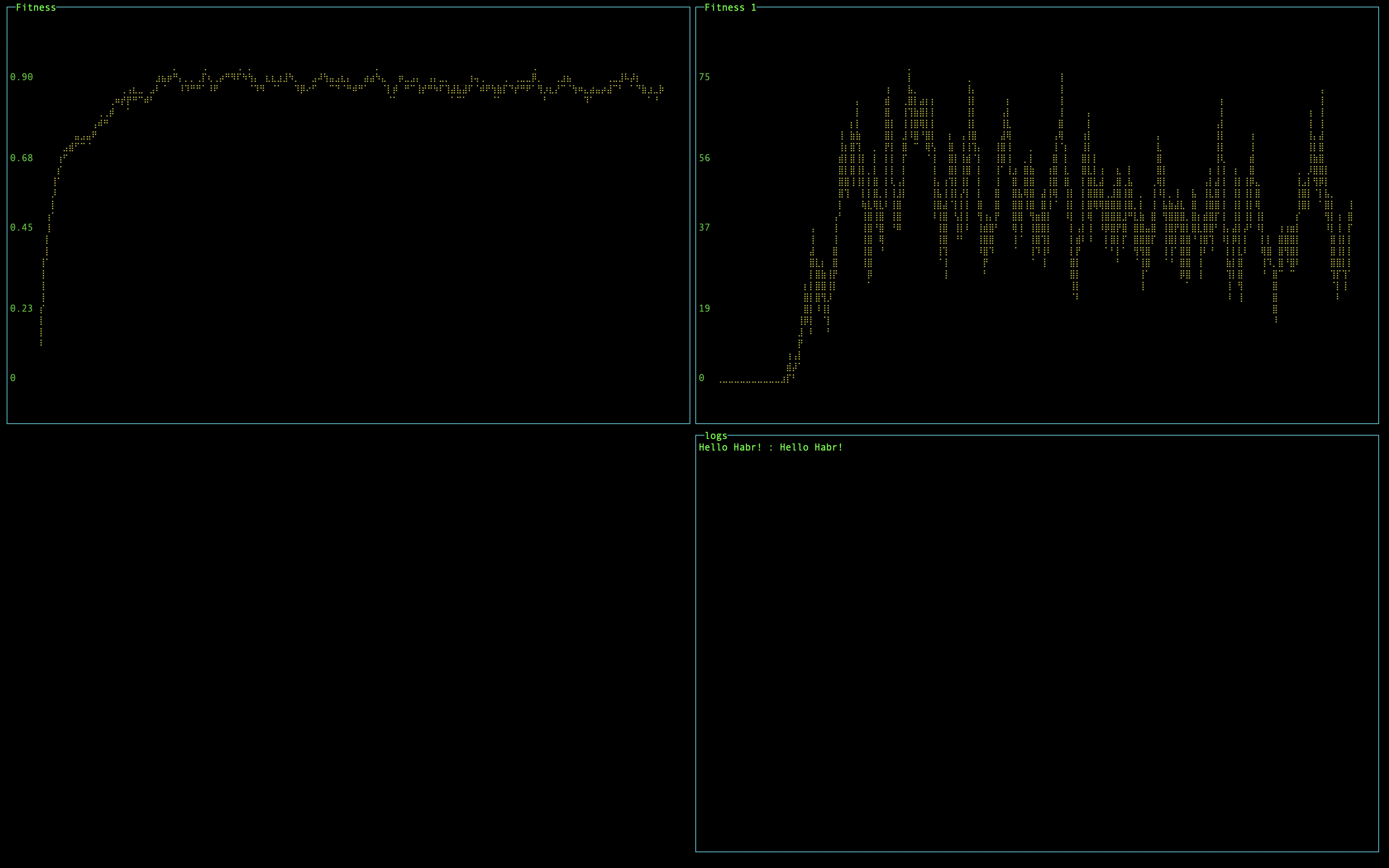
, .
, 90% , 100% .
Fintess 1, 100% . 20 75 .
. Hello Habr! . To be or not to be:

, 1000 . , .
:
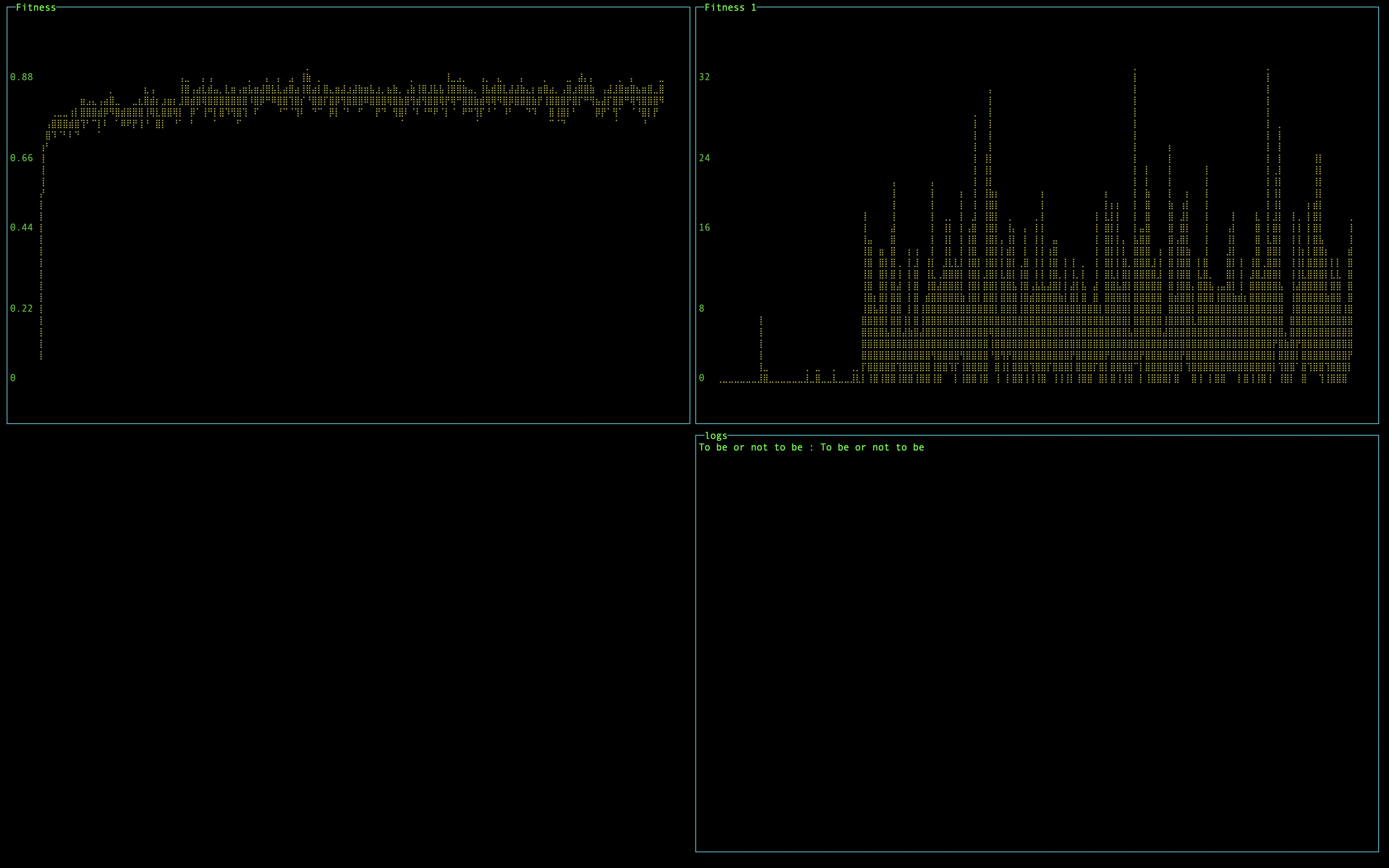
, 0.1% 1%?

, 80% 31-33%. .
. , , .
, ? , .
. .

, . 3 : adaptive — , adaptiveThreshold — , cutLength — .
:
const TARGET = 'To be or not to be To be or not to be';
const GENERATIONS = 1000;
const POPULATION_NUMBER = 150;
const MUTATION_RATE = 0.01;
const population = new Population(TARGET, DNA, POPULATION_NUMBER, MUTATION_RATE, true, 0.5, 7);

, . 1000 , . , ?
. . . . , , 100% .
, , .
:
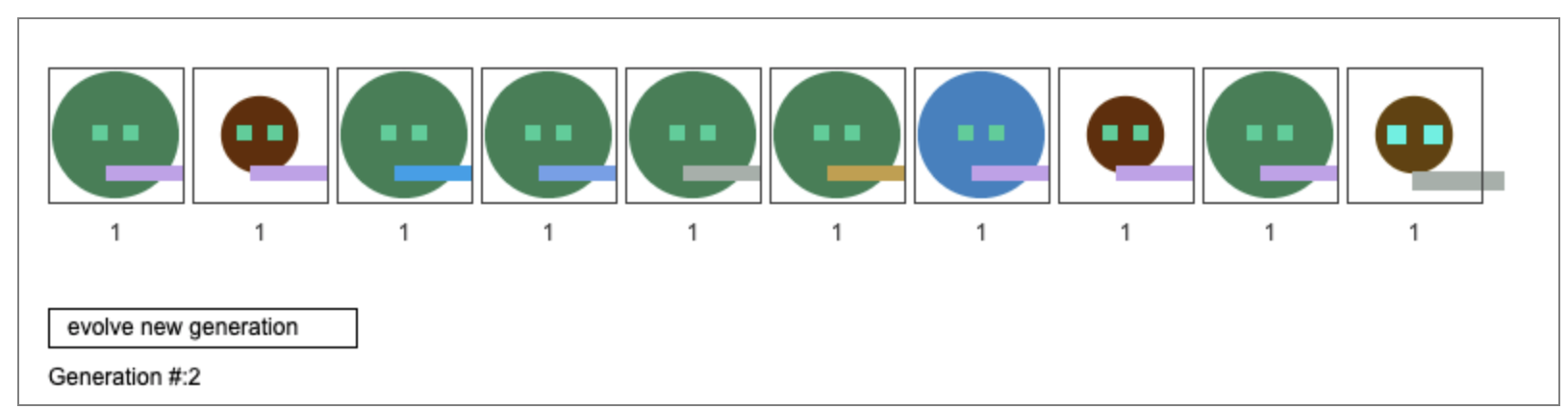
( , . )
:
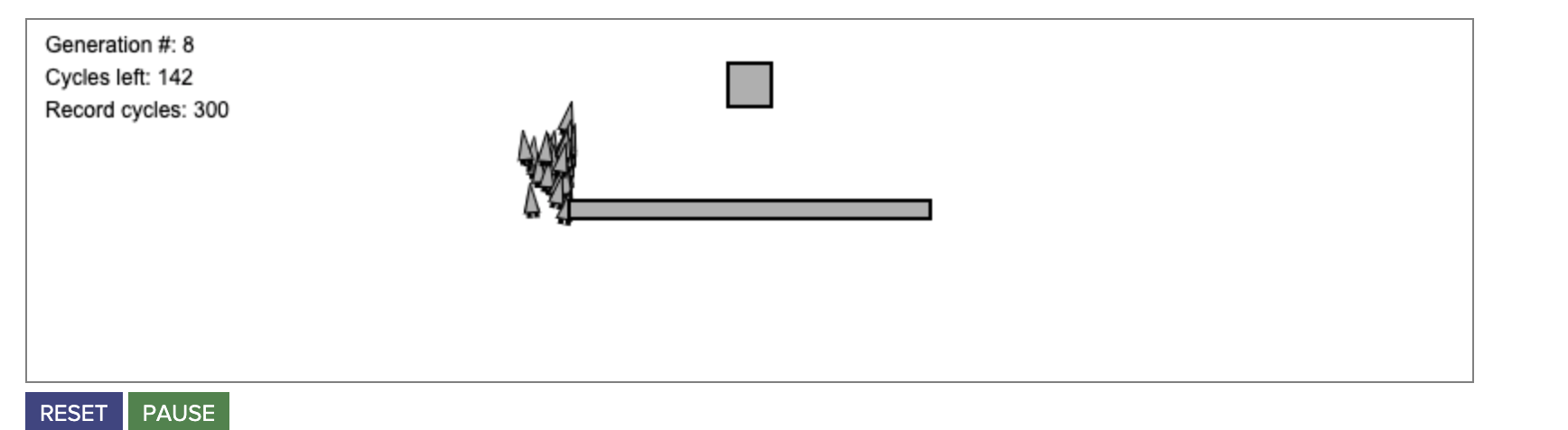
:
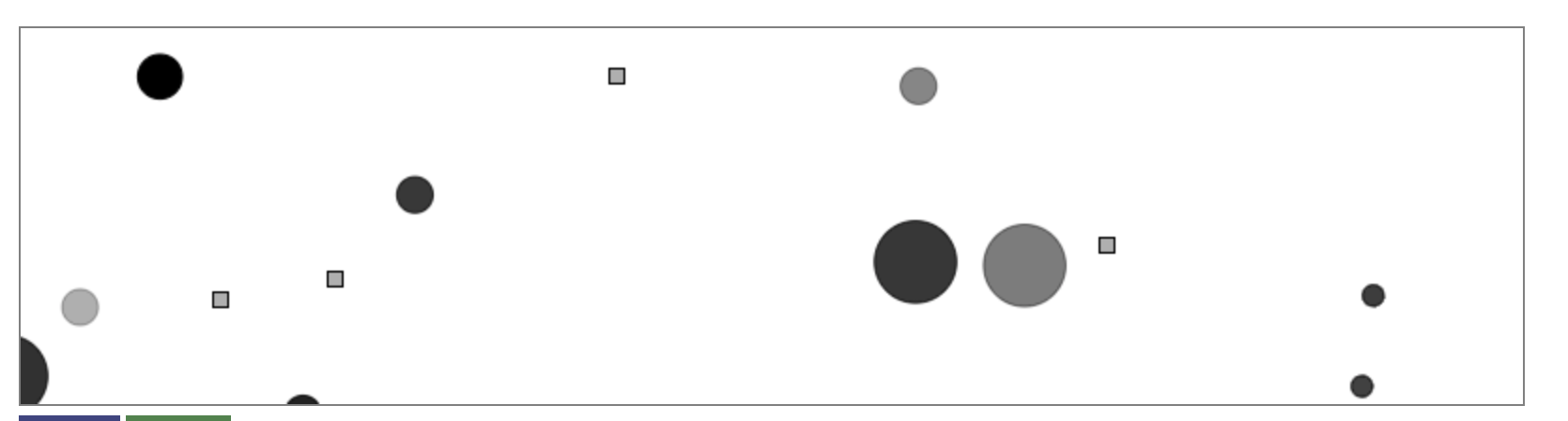
( — )
.
سننظر في مثل هذه الحلول في المقالة التالية. يبدو أنني دخلت أيضًا في مجال نظري مثل اللغة https://processing.org/ وإطار HYPE الذي يتم إنشاء العديد من الأشياء المثيرة للاهتمام. سأحاول اللعب معهم.