في هذا المثال الصغير ، أريد أن أوضح كيفية تحليل البيانات من المواقع وكيفية استخدامها بشكل أكبر للتحليل. للقيام بذلك ، قمت بتحليل جدول تقييمات القبيلة من لعبة World of Tanks ونظرت في كيفية ارتباط تصنيف القبيلة مع البيانات الأخرى.
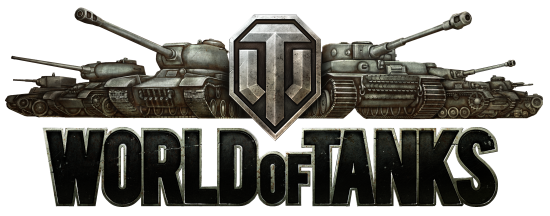
1. تحليل البيانات
import numpy as np
import pandas as pd
from scrapy.selector import Selector
import matplotlib.pyplot as plt
import seaborn as sns
%matplotlib inline
sns.set(rc={'figure.figsize':(20, 5)})
javascript , - scrapy html , ( selenium) scrapy Selector
.
with open(' _ Wargaming.net.html', 'r') as f:
html_text = f.read()
selector = Selector(text=html_text)
xpath
- youtube.com, . , .. (. ), , text
, ( ), , , .
df = pd.DataFrame()
table = selector.xpath('//div[@class="js-widget-content"]/div[2]/div[2]/div') + selector.xpath('//div[@class="js-widget-content"]/div[2]/div[4]/div')
for row in table:
text = row.xpath('*//text()').extract()
clan = text[-10]
cr = int(text[-7].replace(' ',''))
wpr = int(text[-6].replace(' ',''))
abd = float(text[-5].replace(',', '.'))
avl_10 = int(text[-4])
fsh = int(text[-3].replace(' ',''))
wgm = int(text[-2].replace(' ',''))
wsh = int(text[-1].replace(' ',''))
df = df.append({'Clan' : clan,
'CR' : cr,
'wPR' : wpr,
'aB_D' : abd,
'aVL10' : avl_10,
'fSH' : fsh,
'wGM' : wgm,
'wSH' : wsh},
ignore_index=True)
df
, 25, 5 .
print('- :', len(df))
- : 25
df.head()
| CR | Clan | aB_D | aVL10 | fSH | wGM | wPR | wSH |
---|
0 | 15486.0 | [CM-1] | 18.20 | 34.0 | 4083.0 | 2253.0 | 10325.0 | 2294.0 |
---|
1 | 15148.0 | [R-BOY] | 18.86 | 37.0 | 3745.0 | 1943.0 | 10267.0 | 2066.0 |
---|
2 | 15041.0 | [CYS] | 17.47 | 32.0 | 3649.0 | 2300.0 | 10251.0 | 1857.0 |
---|
3 | 14984.0 | [I-YAN] | 16.85 | 28.0 | 4080.0 | 2468.0 | 8992.0 | 2290.0 |
---|
4 | 14952.0 | [YETT1] | 17.41 | 29.0 | 4222.0 | 2159.0 | 8387.0 | 2474.0 |
---|
2.
, - , . .
plt.xticks(rotation=45, ha="right")
ax = sns.lineplot(x='Clan', y='CR', data=df, marker='o', color='r', sort=False)
ax.set(xlabel='', ylabel=' ')
ax.set(xticks=df['Clan'].values);

. , ?
def draw_corr(df, y1, y1_label, y2='CR', y2_label=' '):
fig, ax = plt.subplots()
plt.xticks(rotation=45, ha="right")
sns.lineplot(x='Clan', y=y1, data=df, marker='o', color='b', label=y1_label, sort=False)
ax.set(xlabel='', ylabel=y1_label)
plt.legend(bbox_to_anchor=(0.01, 0.95), loc='upper left')
ax2 = ax.twinx()
sns.lineplot(x='Clan', y=y2, data=df, marker='o', color='r', label=y2_label, sort=False)
ax2.set(ylabel=y2_label)
plt.legend(bbox_to_anchor=(0.01, 0.85), loc='upper left');
draw_corr(df, 'wPR', ' ')

draw_corr(df, 'aVL10', ' ')

3.
تم كتابة هذه المقالة خصيصًا لإظهار أنه لا يوجد شيء معقد في تحليل البيانات والتحليلات. من البيانات التي تم الحصول عليها ، يمكنك عمل المزيد من الرسوم البيانية للارتباط ، وبناء الرسوم البيانية ، إذا لزم الأمر ، وإجراء بعض التنبؤات ، إلخ.
→ كود المصدر